AutoML aims to ease the process of building ML models by automating commonly-used steps, such as feature preprocessing, model selection, and hyperparameters tuning. You will see each of these steps in detail in coming chapters and you will actually build an AutoML system to have a deeper understanding of the available tools and libraries for AutoML.
Without getting into the details, it's useful to review what an ML model is and how you train one.
ML algorithms will work on your data to find certain patterns, and this learning process is called model training. As a result of model training, you will have an ML model that supposedly will give you insights/answers about the data without requiring you to write explicit rules.
When you are using ML models in practice, you will throw a bunch of numerical data as input for training the algorithm. The output of the training process is a ML model that you can use to make predictions. Predictions can help you to decide whether your server should be maintained in the next four hours based on its current state, or whether a customer of yours is going to switch to your competitor or not.
Sometimes the problem you are solving will not be well-defined and you will not even know what kind of answers you are looking for. In such cases, ML models will help you to explore your dataset, such as identifying a cluster of customers that are similar to each other in terms of behavior or finding the hierarchical structure of stocks based on their correlations.
What do you do when your model comes up with clusters of customers? Well, you at least know this: customers that belong to the same cluster are similar to each other in terms of their features, such as their age, profession, marital status, gender, product preferences, daily/weekly/monthly spending habits, total amount spent, and so on. Customers who belong to different clusters are dissimilar to each other. With such an insight, you can utilize this information to create different ad campaigns for each cluster.
To put things into a more technical perspective, let's understand this process in simple mathematical terms. There is a dataset X, which contains n examples. These examples could represent customers or different species of animals. Each example is usually a set of real numbers, which are called features, for example if we have a female, 35 year old customer who spent $12000 at your store, you can represent this customer with the following vector (0.0, 35.0, 12000.0). Note that the gender is represented with 0.0, this means that a male customer would have 1.0 for that feature. The size of the vector represents the dimensionality. Since this is a vector of size three, which we usually denote by m, this is a three-dimensional dataset.
Depending on the problem type, you might need to have a label for each example. For example, if this is a supervised learning problem such as binary classification, you could label your examples with 1.0 or 0.0 and this new variable is called label or target variable. The target variable is usually referred to as y.
Having x and y, an ML model is simply a function, f, with weights, w (model parameters):
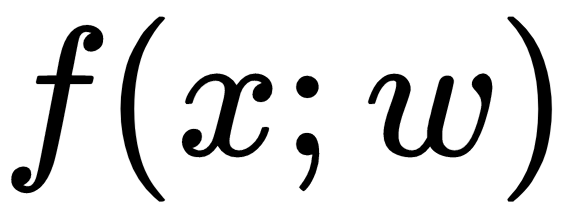
Model parameters are learned during the training process, but there are also other parameters that you might need to set before training starts, and these parameters are called hyperparameters, which will be explained shortly.
Features in your dataset usually should be preprocessed before being used in model training. For example, some of the ML models implicitly assume that features are distributed normally. In many real-life scenarios this is not the case, and you can benefit from applying feature transformations such as log transformation to have them normally distributed.
Once feature processing is done and model hyperparameters are set, model training starts. At the end of model training, model parameters will be learned and we can predict the target variable for new data that the model has not seen before. Prediction made by the model is usually referred to as :
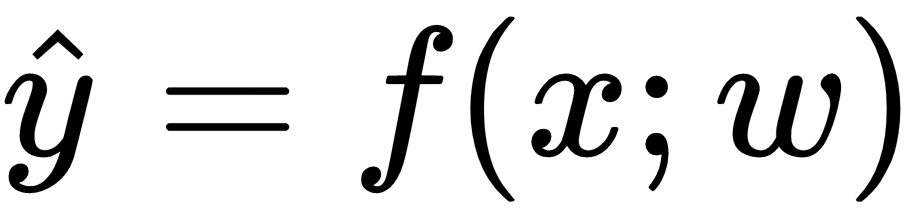
What really happens during training? Since we know the labels for the dataset we used for training, we can iteratively update our model parameters based on the comparison of what our current model predicts and what the original label was.
This comparison is based on a function called loss function (or cost function), . Loss function represents the inaccuracy of predictions. Some of the common loss functions you may have heard of are square loss, hinge loss, logistic loss, and cross-entropy loss.
Once model training is done, you will test the performance of your ML model on test data, which is the dataset that has not been used in the training process, to see how well your model generalizes. You can use different performance metrics to assess the performance; based on the results, you should go back to previous steps and do multiple adjustments to achieve better performance.
At this point, you should have an overall idea of what training an ML model looks like under the hood.
What is AutoML then? When we are talking about AutoML, we mostly refer to automated data preparation (namely feature preprocessing, generation, and selection) and model training (model selection and hyperparameter optimization). The number of possible options for each step of this process can vary vastly depending on the problem type.
AutoML allows researchers and practitioners to automatically build ML pipelines out of these possible options for every step to find high-performing ML models for a given problem.
The following figure shows a typical ML model life cycle with a couple of examples for every step:
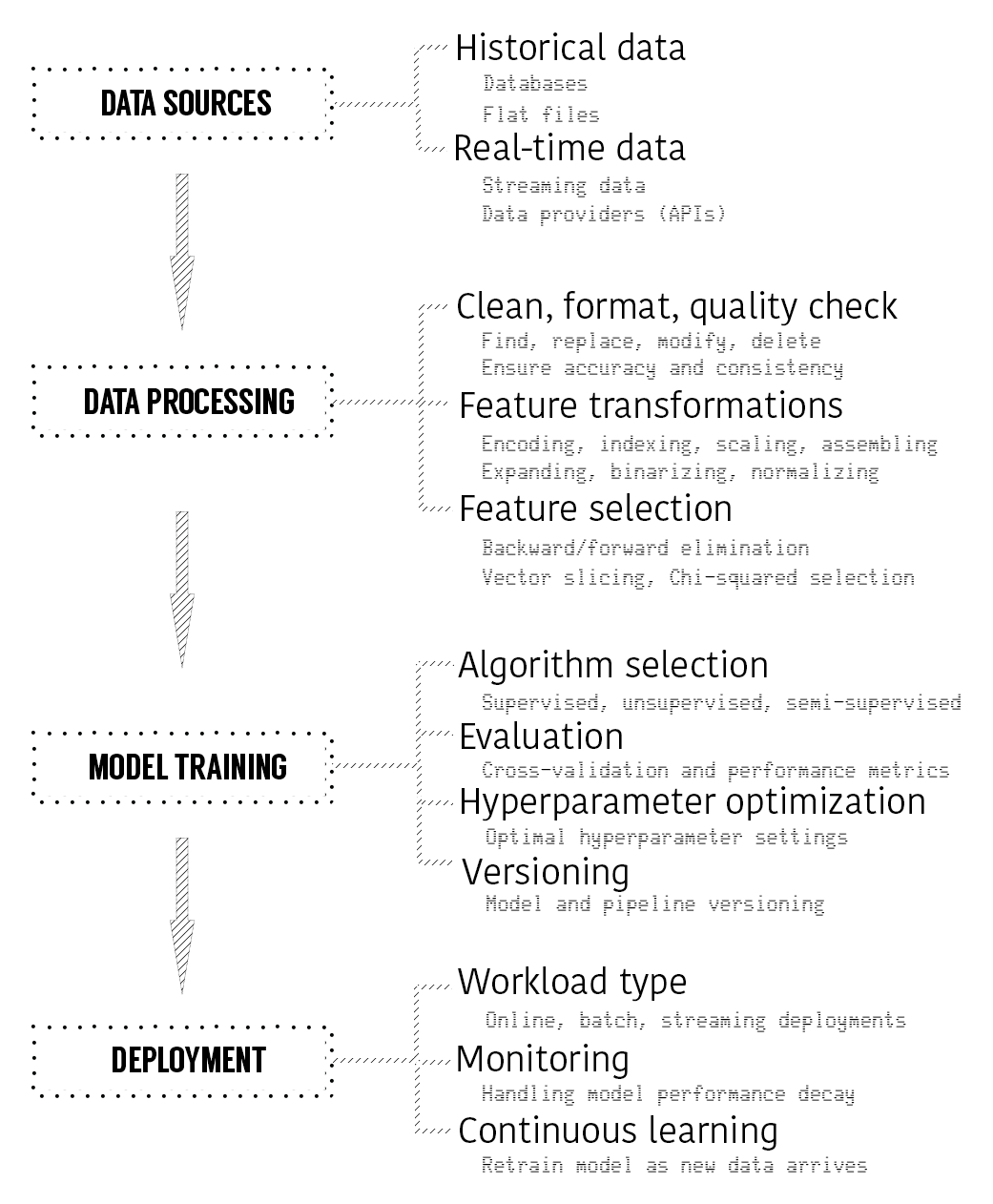
Data can be ingested from various sources such as flat files, databases, and APIs. Once you are able to ingest the data, you should process it to make it ready for ML and there are typical operations such as cleaning and formatting, feature transformation, and feature selection. After data processing, your final dataset should be ready for ML and you will shortlist candidate algorithms to work. Shortlisted algorithms should be validated and tuned through techniques such as cross-validation and hyperparameter optimization. Your final model will be ready to be operationalized with suitable workload type such as online, batch and streaming deployment. Once model is in production, you need to monitor its performance and take necessary action if needed such as re-training, re-evaluation, and re-deployment.
Once you are faced with building ML models, you will first do research on the domain you are working on and identify your objective. There are many steps involved in the process which should be planned and documented in advance before you actually start working on it. To learn more about the whole process of project management, you can refer to CRISP-DM model (https://en.wikipedia.org/wiki/Cross-industry_standard_process_for_data_mining), project management is crucially important to deliver a successful application, however, it's beyond the scope of this book.
In terms of building ML pipelines, you will usually have multiple data sources, such as relational databases or flat files, where you can get historical data. You can also have streaming data flowing into your systems from various resources.
You will work on these data sources to understand which of them could be useful for your particular task, then you will proceed to the data processing step where you will do lots of cleaning, formatting, and data quality checks followed by feature transformations and selection.
When you decide that your dataset is ready to be fed into ML models, you will need to think about working with one or more suitable ML models. You will train multiple models, evaluate them, and search for optimal hyperparameter settings. Versioning at this point will help you to keep track of changes. As a result of your experimentation, you will have a performance ML pipeline with every step optimized for performance. The best performing ML pipeline will be the one you would like to test drive in a production environment and that's the point where you would like to operationalize it in the deployment step.
Operationalizing an ML pipeline means that you need to choose a deployment type. Some of the workloads will be for batch processing the data you have in databases, and in that case you need batch deployment. Others could be for processing real-time data provided by various data providers, where you will need streaming deployment.
If you carefully examine each of these steps, especially the options in data processing and training steps are vast. First you need to select appropriate methods and algorithms, then you should also fine-tune hyperparameters for selected methods and algorithms for them to best perform for your given problem.
Just to give a simple example, let's assume that you are done with the steps up to model training step, you need to select a set of ML models to experiment. To make things simpler, let's say the only algorithm you would like to experiment with is k-means, it's just about tuning its parameters.
A k-means algorithm helps to cluster similar data points together. The following code snippet uses the scikit-learn library and you can install it using pip (http://scikit-learn.org/stable/install.html), don't worry if you don't understand every line:
# Sklearn has convenient modules to create sample data.
# make_blobs will help us to create a sample data set suitable for clustering
from sklearn.datasets.samples_generator import make_blobs
X, y = make_blobs(n_samples=100, centers=2, cluster_std=0.30, random_state=0)
# Let's visualize what we have first
import matplotlib.pyplot as plt
import seaborn as sns
plt.scatter(X[:, 0], X[:, 1], s=50)
The output of the preceding code snippet is as follows:
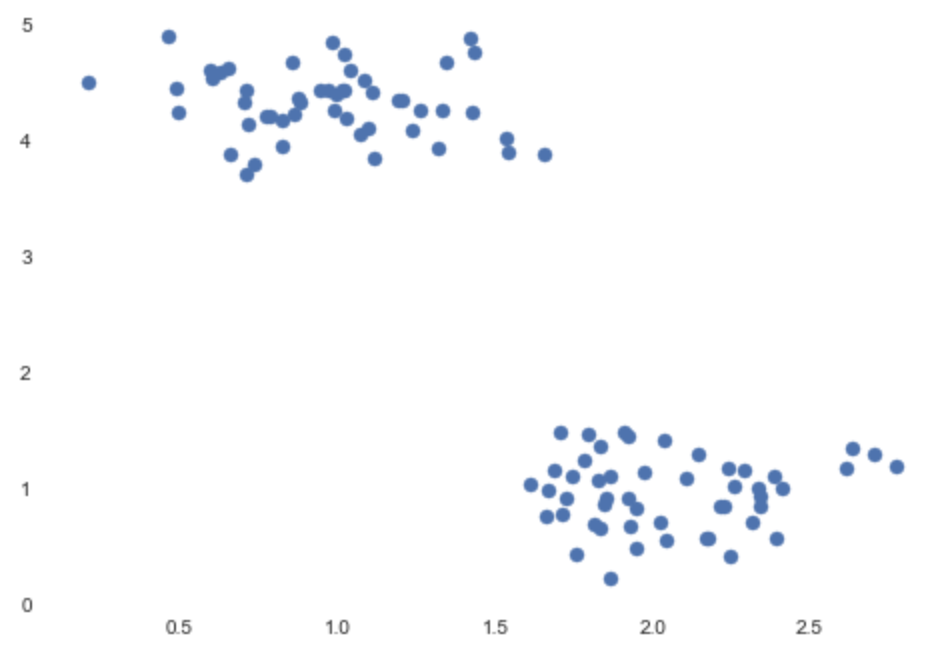
You can easily see that we have two clusters on the plot:
# We will import KMeans model from clustering model family of Sklearn
from sklearn.cluster import KMeans
k_means = KMeans(n_clusters=2)
k_means.fit(X)
predictions = k_means.predict(X)
# Let's plot the predictions
plt.scatter(X[:, 0], X[:, 1], c=predictions, cmap='brg')
The output of the preceding code snippet is as follows:
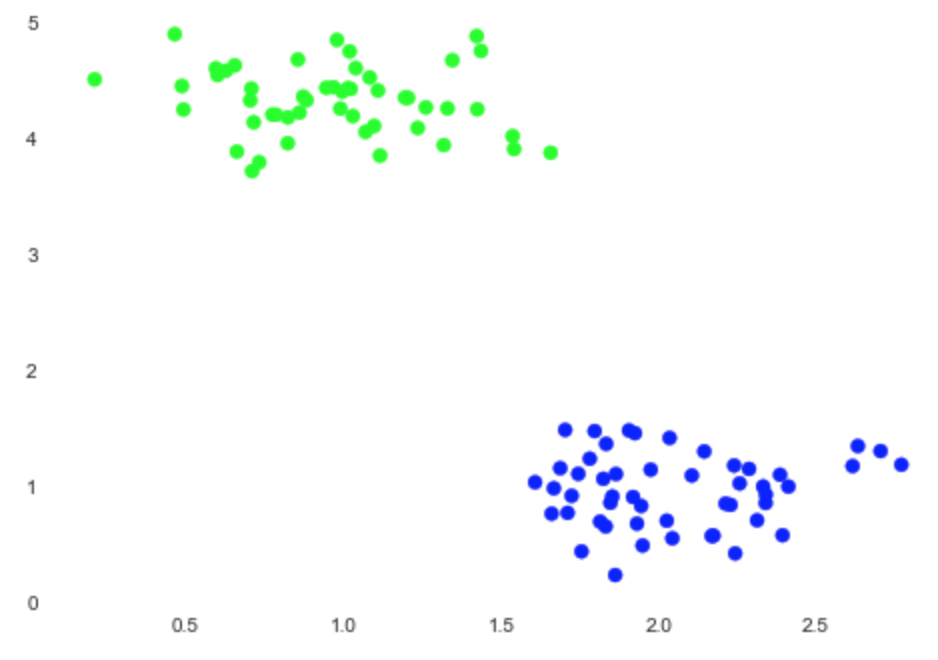
Nice! Our algorithm worked as we expected. Astute readers may have noticed that there was an argument named n_clusters for the k-means model. When you provide this value to the k-means algorithm, it will try to split this dataset into two clusters. As you can guess, k-means's hyperparameter in this case is the number of clusters. The k-means model needs to know this parameter before training.
Different algorithms have different hyperparameters such as depth of tree for decision trees, number of hidden layers, learning rate for neural networks, alpha parameter for Lasso or C, kernel, and gamma for Support Vector Machines (SVMs).
Let's see how many arguments the k-means model has by using the get_params method:
k_means.get_params()
The output will be the list of all parameters that you can optimize:
{'algorithm': 'auto', 'copy_x': True, 'init': 'k-means++', 'max_iter': 300, 'n_clusters': 2, 'n_init': 10, 'n_jobs': 1, 'precompute_distances': 'auto', 'random_state': None, 'tol': 0.0001, 'verbose': 0}
In most real-life use cases, you will neither have resources nor time for trying each possible combination with the options of all steps considered.
AutoML libraries come to your aid at this point by carefully setting up experiments for various ML pipelines, which covers all the steps from data ingestion, data processing, modeling, and scoring.