Governing your ML system
A great part of system governance involves quality assurance and control, model auditing, and reporting to have end-to-end trackability and compliance with regulations. The ML systems' efficacy (that is, its ability to produce a desired or intended result) is dependent on the way it is governed to achieve maximum business value. So far, we have monitored and analyzed our deployed model for inference data:
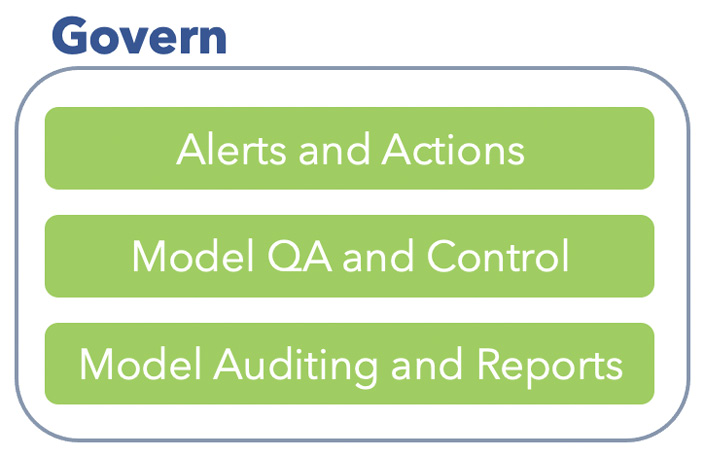
Figure 12.27 – Components of governing your ML system
The efficacy of an ML system can be determined by using smart actions that are taken based on monitoring and alerting. In the next chapter, we will explore ML system governance in terms of alerts and actions, model QA and control, and model auditing and reports.