Evaluating embeddings – analogical reasoning
Analogical reasoning is a simple and efficient way to evaluate embeddings by predicting syntactic and semantic relationships of the form a is to b as c is to _?, denoted as a : b → c : ?. The task is to identify the held-out fourth word, with only exact word matches deemed correct.
For example, the word woman is the best answer to the question king is to queen as man is to?. Assume that is the representation vector for the word
normalized to unit norm. Then, we can answer the question a : b → c : ? , by finding the word
with the representation closest to:

According to cosine similarity:
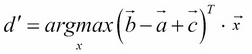
Now let us implement the analogy prediction function using Theano. First, we need to define the input of the function. The analogy function receives three inputs, which are the word indices of a
, b
, and c
:
analogy_a = T.ivector('analogy_a') analogy_b = T.ivector('analogy_b') analogy_c = T.ivector('analogy_c')
Then, we need to map each input to the word embedding...