



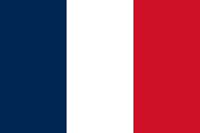


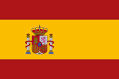




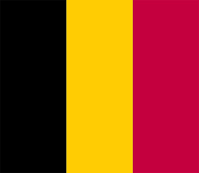
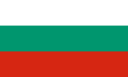
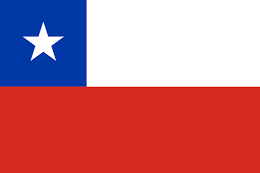
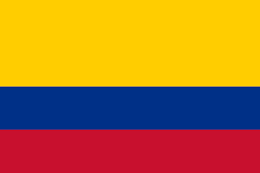
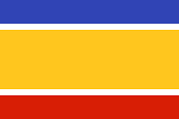

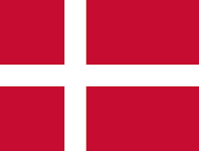



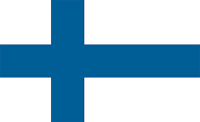

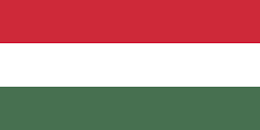






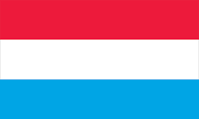
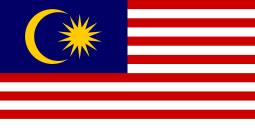

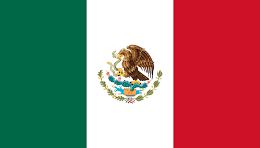

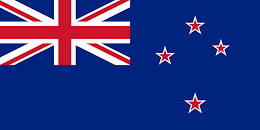







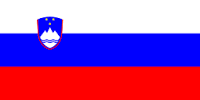




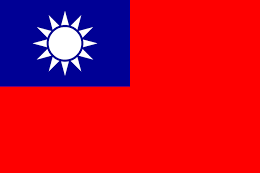



In this article by Ralph Winters, the author of the book Practical Predictive Analytics we will explore the idea of how to start with predictive analysis.
"In God we trust, all other must bring Data" – Deming
(For more resources related to this topic, see here.)
I enjoy explaining Predictive Analytics to people because it is based upon a simple concept: Predicting the probability of future events based upon historical data. Its history may date back to at least 650 BC. Some early examples include the Babylonians, who tried to predict short term weather changes based cloud appearances and haloes
Medicine also has a long history of a need to classify diseases. The Babylonian king Adad-apla-iddina decreed that medical records be collected to form the “Diagnostic Handbook”. Some “predictions” in this corpus list treatments based on the number of days the patient had been sick, and their pulse rate. One of the first instances of bioinformatics!
In later times, specialized predictive analytics were developed at the onset of the Insurance underwriting industries. This was used as a way to predict the risk associated with insuring Marine Vessels. At about the same time, Life Insurance companies began predicting the age that a person would live in order to set the most appropriate premium rates. [i]Although the idea of prediction always seemed to be rooted early in humans’ ability to want to understand and classify, it was not until the 20th century, and the advent of modern computing that it really took hold.
In addition to aiding the US government in the 1940 with breaking the code, Alan Turing also worked on the initial computer chess algorithms which pitted man vs. machine. Monte Carlo simulation methods originated as part of the Manhattan project, where mainframe computers crunched numbers for days in order to determine the probability of nuclear attacks.
In the 1950’s Operation Research theory developed, in which one could optimize the shortest distance between two points. To this day, these techniques are used in logistics by companies such as UPS and Amazon.
Non mathematicians have also gotten into the act. In the 1970’s, Cardiologist Lee Goldman (who worked aboard a submarine) spend years developing a decision tree which did this efficiently. This helped the staff determine whether or not the submarine needed to resurface in order to help the chest pain sufferer!
What many of these examples had in common was that history was used to predict the future. Along with prediction, came understanding of cause and effect and how the various parts of the problem were interrelated. Discovery and insight came about through methodology and adhering to the scientific method.
Most importantly, the solutions came about in order to find solutions to important, and often practical problems of the times. That is what made them unique.
We have come a long way from then, and Practical Analytics solutions have furthered growth in so many different industries. The internet has had a profound effect on this; it has enabled every click to be stored and analyzed. More data is being collected and stored, some with very little effort. That in itself has enabled more industries to enter Predictive Analytics.
Marketing has always been concerned with customer acquisition and retention, and has developed predictive models involving various promotional offers and customer touch points, all geared to keeping customers and acquiring new ones. This is very pronounced in certain industries, such as wireless and online shopping cards, in which customers are always searching for the best deal. Specifically, advanced analytics can help answer questions like "If I offer a customer 10% off with free shipping, will that yield more revenue than 15% off with no free shipping?". The 360-degree view of the customer has expanded the number of ways one can engage with the customer, therefore enabling marketing mix and attribution modeling to become increasingly important. Location based devices have enabled marketing predictive applications to incorporate real time data to issue recommendation to the customer while in the store.
Predictive Analytics in Healthcare has its roots in clinical trials, which uses carefully selected samples to test the efficacy of drugs and treatments. However, healthcare has been going beyond this. With the advent of sensors, data can be incorporated into predictive analytics to monitor patients with critical illness, and to send alerts to the patient when he is at risk. Healthcare companies can now predict which individual patients will comply with courses of treatment advocated by health providers. This will send early warning signs to all parties, which will prevent future complications, as well as lower the total costs of treatment.
Other examples can be found in just about every other industry. Here are just a few:
Although these industries can be quite different, the goals of predictive analytics are typically implement to increase revenue, decrease costs, or alter outcomes for the better.
So what skills do you need to be successful in Predictive Analytics? I believe that there are 3 basic skills that are needed:
Along with the term Predictive Analytics, here are some terms which are very much related:
Originally predictive analytics was performed by hand, by statisticians on mainframe computers using a progression of various language such as FORTRAN etc. Some of these languages are still very much in use today. FORTRAN, for example, is still one of the fasting performing languages around, and operators with very little memory.
Nowadays, there are some many choices on which software to use, and many loyalists remain true to their chosen software. The reality is, that for solving a specific type of predictive analytics problem, there exists a certain amount of overlap, and certainly the goal is the same. Once you get a hang of the methodologies used for predictive analytics in one software packages, it should be fairly easy to translate your skills to another package.
Open source emphasis agile development, and community sharing. Of course, open source software is free, but free must also be balance in the context of TCO (Total Cost of Ownership)
The R language is derived from the "S" language which was developed in the 1970’s. However, the R language has grown beyond the original core packages to become an extremely viable environment for predictive analytics.
Although R was developed by statisticians for statisticians, it has come a long way from its early days. The strength of R comes from its 'package' system, which allows specialized or enhanced functionality to be developed and 'linked' to the core system.
Although the original R system was sufficient for statistics and data mining, an important goal of R was to have its system enhanced via user written contributed packages. As of this writing, the R system contains more than 8,000 packages. Some are of excellent quality, and some are of dubious quality. Therefore, the goal is to find the truly useful packages that add the most value.
Most, if not all of the R packages in use, address most of the common predictive analytics tasks that you will encounter. If you come across a task that does not fit into any category, chances are good that someone in the R community has done something similar. And of course, there is always a chance that someone is developing a package to do exactly what you want it to do. That person could be eventually be you!.
Closed Source Software such as SAS and SPSS were on the forefront of predictive analytics, and have continued to this day to extend their reach beyond the traditional realm of statistics and machine learning. Closed source software emphasis stability, better support, and security, with better memory management, which are important factors for some companies.
There is much debate nowadays regarding which one is 'better'. My prediction is that they both will coexist peacefully, with one not replacing the other. Data sharing and common API's will become more common. Each has its place within the data architecture and ecosystem is deemed correct for a company. Each company will emphasis certain factors, and both open and closed software systems and constantly improving themselves.
Man does not live by bread alone, so it would behoove you to learn additional tools in addition to R, so as to advance your analytic skills.
Given that the Predictive Analytics space is so huge, once you are past the basics, ask yourself what area of Predictive analytics really interests you, and what you would like to specialize in. Learning all you can about everything concerning Predictive Analytics is good at the beginning, but ultimately you will be called upon because you are an expert in certain industries or techniques. This could be research, algorithmic development, or even for managing analytics teams. But, as general guidance, if you are involved in, or are oriented towards data the analytics or research portion of data science, I would suggest that you concentrate on data mining methodologies and specific data modeling techniques which are heavily prevalent in the specific industries that interest you. For example, logistic regression is heavily used in the insurance industry, but social network analysis is not. Economic research is geared towards time-series analysis, but not so much cluster analysis.
If you are involved more on the data engineering side, concentrate more on data cleaning, being able to integrate various data sources, and the tools needed to accomplish this.
If you are a manager, concentrate on model development, testing and control, metadata, and presenting results to upper management in order to demonstrate value.
Of course, predictive analytics is becoming more of a team sport, rather than a solo endeavor, and the Data Science team is very much alive. There is a lot that has been written about the components of a Data Science team, much of it which can be reduced to the 3 basic skills that I outlined earlier.
Depending upon how you intend to approach a particular problem, look at how two different analytical mindsets can affect the predictive analytics process.
Of course the above examples illustrate two disparate approaches. Combination models, which use the best of both worlds should be the ones we should strive for. A model which has an acceptable prediction error, is stable over, and is simple enough to understand. You will learn later that is this related to Bias/Variance Tradeoff
R Installation is typically done by downloading the software directly from the CRAN site
Although installing R directly from the CRAN site is the way most people will proceed, I wanted to mention some alternative R installation methods. These methods are often good in instances when you are not always at your computer.
After you install R on your own machine, I would give some thought about how you want to organize your data, code, documentation, etc. There probably be many different kinds of projects that you will need to set up, all ranging from exploratory analysis, to full production grade implementations. However, most projects will be somewhere in the middle, i.e. those projects which ask a specific question or a series of related questions. Whatever their purpose, each project you will work on will deserve their own project folder or directory.
We will start by creating folders for our environment. Create a sub directory named “PracticalPredictiveAnalytics” somewhere on your computer. We will be referring to it by this name throughout this book.
Often project start with 3 sub folders which roughly correspond with 1) Data Source, 2) Code Generated Outputs, and 3) The Code itself (in this case R)
Create 3 subdirectories under this Project Data, Outputs, and R. The R directory will hold all of our data prep code, algorithms etc. The Data directory will contain our raw data sources, and the Output directory will contain anything generated by the code. This can be done natively within your own environment, e.g. you can use Windows Explorer to create these folders.
Some important points to remember about constructing projects
R, like many languages and knowledge discovery systems started from the command line (one reason to learn Linux), and is still used by many. However, predictive analysts tend to prefer Graphic User Interfaces, and there are many choices available for each of the 3 different operating systems. Each of them have their strengths and weakness, and of course there is always a matter of preference. Memory is always a consideration with R, and if that is of critical concern to you, you might want to go with a simpler GUI, like the one built in with R. If you want full control, and you want to add some productive tools, you could choose RStudio, which is a full blown GUI and allows you to implement version control repositories, and has nice features like code completion. RCmdr, and Rattle’s unique features are that they offer menus which allow guided point and click commands for common statistical and data mining tasks. They are always both code generators. This is a good for learning, and you can learn by looking at the way code is generated.
Both RCmdr and RStudio offer GUI's which are compatible with Windows, Apple, and Linux operator systems, so those are the ones I will use to demonstrate examples in this book. But bear in mind that they are only user interfaces, and not R proper, so, it should be easy enough to paste code examples into other GUI’s and decide for yourself which ones you like.
After R installation has completed, download and install the RStudio executable appropriate for your operating system
Click the RStudio Icon to bring up the program: The program initially starts with 3 tiled window panes, as shown below. Before we begin to do any actual coding, we will want to set up a new Project.
Create a new project by following these steps:
Now that we have created a project, let’s take a look at of the R Console Window. Click on the window marked “Console” and perform the following steps:
The getwd() command is very important since it will always tell you which directory you are in. Sometimes you will need to switch directories within the same project or even to another project. The command you will use is setwd(). You will supply the directory that you want to switch to, all contained within the parentheses.
This is a situation we will come across later. We will not change anything right now. The point of this, is that you should always be aware of what your current working directory is.
The script window is where all of the R Code is written. You can have several script windows open, all at once.
Press Ctrl + Shift + N to create a new R script. Alternatively, you can go through the menu system by selecting File/New File/R Script. A new blank script window will appear with the name “Untitled1”
Now that all of the preliminary things are out of the way, we will code our first extremely simple predictive model.
Our first R script is a simple two variable regression model which predicts women’s height based upon weight. The data set we will use is already built into the R package system, and is not necessary to load externally. For quick illustration of techniques, I will sometimes use sample data contained within specific R packages to demonstrate.
Paste the following code into the “Untitled1” scripts that was just created:
require(graphics)
data(women)
head(women)
utils::View(women)
plot(women$height,women$weight)
Click Ctrl+Shift+Enter to run the entire code. The display should change to something similar as displayed below.
What you have actually done is:
The very first statement in the code “require” is just a way of saying that R needs a specific package to run. In this case require(graphics) specifies that the graphics package is needed for the analysis, and it will load it into memory. If it is not available, you will get an error message. However, “graphics” is a base package and should be available
To save this script, press Ctrl-S (File Save) , navigate to the PracticalPredictiveAnalytics/R folder that was created, and name it Chapter1_DataSource
Create another Rscript by Ctrl + Shift + N to create a new R script. A new blank script window will appear with the name “Untitled2”
Paste the following into the new script window
lm_output <- lm(women$height ~ women$weight)
summary(lm_output)
prediction <- predict(lm_output)
error <- women$height-prediction
plot(women$height,error)
Press Ctrl+Shift+Enter to run the entire code. The display should change to something similar to what is displayed below.
Here are some notes and explanations for the script code that you have just ran:
lm_output <- lm(women$height ~ women$weight
There are two operations that you will become very familiar with when running Predictive Models in R.
Note that the execution of this line does not produce any displayed output. You can see if the line was executed by checking the console. If there is any problem with running the line (or any line for that matter) you will see an error message in the console.
summary(lm_output)
The results will appear in the Console window as pictured in the figure above.
Look at the lines market (Intercept), and women$weight which appear under the Coefficients line in the console. The Estimate Column shows the formula needed to derive height from weight. Like any linear regression formula, it includes coefficients for each independent variable (in our case only one variable), as well as an intercept. For our example the English rule would be "Multiply weight by 0.2872 and add 25.7235 to obtain height".
Coefficients:
Estimate Std. Error t value Pr(>|t|)
(Intercept) 25.723456 1.043746 24.64 2.68e-12 ***
women$weight 0.287249 0.007588 37.85 1.09e-14 ***
---
Signif. codes: 0 ‘***’ 0.001 ‘**’ 0.01 ‘*’ 0.05 ‘.’ 0.1 ‘ ’ 1
Residual standard error: 0.44 on 13 degrees of freedom
Multiple R-squared: 0.991, Adjusted R-squared: 0.9903
F-statistic: 1433 on 1 and 13 DF, p-value: 1.091e-14
We have already assigned the output of the lm() function to the lm_output object. Let’s apply another function to lm_output as well. The predict() function “reads” the output of the lm function and predicts (or scores the value), based upon the linear regression equation. In the code we have assigned the output of this function to a new object named "prediction”.
Switch over to the console area, and type “prediction” to see the predicted values for the 15 women. The following should appear in the console.
> prediction
1 2 3 4 5 6 7
58.75712 59.33162 60.19336 61.05511 61.91686 62.77861 63.64035
8 9 10 11 12 13 14
64.50210 65.65110 66.51285 67.66184 68.81084 69.95984 71.39608
15
72.83233
There are 15 predictions. Just to verify that we have one for each of our original observations we will use the nrow() function to count the number of rows.
At the command prompt in the console area, enter the command: nrow(women)
The following should appear:
>nrow(women)
[1] 15
The error object is a vector that was computed by taking the difference between the predicted value of height and the actual height. These are also known as the residual errors, or just residuals.
Since the error object is a vector, you cannot use the nrows() function to get its size. But you can use the length() function:
>length(error)
[1] 15
In all of the above cases, the counts all compute as 15, so all is good.
Some important points to be made regarding this first example:
The R-Square for this model is artificially high. Regression is often used in an exploratory fashion to explore the relationship between height and weight. This does not mean a causal one. As we all know, weight is caused by many other factors, and it is expected that taller people will be heavier.
A predictive modeler who is examining the relationship between height and weight would want probably want to introduce additional variables into the model at the expense of a lower R-Square. R-Squares can be deceiving, especially when they are artificially high
After you are done, press Ctrl-S (File Save), navigate to the PracticalPredictiveAnalytics/R folder that was created, and name it Chapter1_LinearRegression
Sometimes the amount of information output by statistic packages can be overwhelming. Sometime we want to reduce the amount of output and reformat it so it is easier on the eyes. Fortunately, there is an R package which reformats and simplifies some of the more important statistics. One package I will be using is named “stargazer”.
Create another R script by Ctrl + Shift + N to create a new R script.
Enter the following lines and then Press Ctrl+Shift+Enter to run the entire script.
install.packages("stargazer")
library(stargazer)
stargazer(lm_output, title="Lm Regression on Height", type="text")
After the script has been run, the following should appear in the Console:
install.packages("stargazer")
This line will install the package to the default package directory on your machine. Make sure you choose a CRAN mirror before you download.
library(stargazer)
This line loads the stargazer package
stargazer(lm_output, title="Lm Regression on Height", type="text")
The reformatted results will appear in the R Console. As you can see, the output written to the console is much cleaner and easier to read
After you are done, press Ctrl-S (File Save), navigate to the PracticalPredictiveAnalytics/Outputs folder that was created, and name it Chapter1_LinearRegressionOutput
The rest of the book will concentrate on what I think are the core packages used for predictive modeling. There are always new packages coming out. I tend to favor packages which have been on CRAN for a long time and have large user base. When installing something new, I will try to reference the results against other packages which do similar things. Speed is another reason to consider adopting a new package.
In this article we have learned a little about what predictive analytics is and how they can be used in various industries. We learned some things about data, and how they can be organized in projects. Finally, we installed RStudio, and ran a simple linear regression, and installed and used our first package. We learned that it is always good practice to examine data after it has been brought into memory, and a lot can be learned from simply displaying and plotting the data.
Further resources on this subject: