Anomaly detection with one-class SVC
The design of the one-class SVC is an extension of the binary SVC. The main difference is that a single class contains most of the baseline (or normal) observations. A reference point, known as the SVC origin, replaces the second class. The outliers (or abnormal) observations reside beyond (or outside) the support vector of the single class:
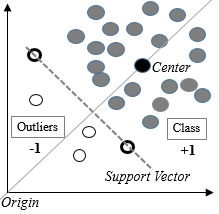
The visualization of the one-class SVC
The outlier observations have a labeled value of -1, while the remaining training sets are labeled +1. In order to create a relevant test, we add four more companies that have drastically cut their dividends (ticker symbols WLT, RGS, MDC, NOK, and GM). The dataset includes the stock prices and financial metrics recorded prior to the cut in dividends.
The implementation of this test case is very similar to the binary SVC driver code, except for the following:
The classifier uses the Nu-SVM formulation,
OneSVFormulation
The labeled data is generated by assigning -1 to companies that...