Docker is a software-contained platform that is used to host multiple software or apps side by side in isolated containers to get better computing density. Unlike virtual machines, containers are built only using libraries and the settings required by any software but do not bundle the entire operating system, thus making it lightweight and efficient.
Installing all three packages at once using Docker
Getting ready
Setting up all three packages could be quite cumbersome depending on the operating system utilized. The following dockerfile code can be used to set up an environment with tensorflow, mxnet with GPU, and h2o installed with all the dependencies:
FROM chstone/mxnet-gpu:latest
MAINTAINER PKS Prakash <prakash5801>
# Install dependencies
RUN apt-get update && apt-get install -y
python2.7
python-pip
python-dev
ipython
ipython-notebook
python-pip
default-jre
# Install pip and Jupyter notebook
RUN pip install --upgrade pip &&
pip install jupyter
# Add R to Jupyter kernel
RUN Rscript -e "install.packages(c('repr', 'IRdisplay', 'crayon', 'pbdZMQ'), dependencies=TRUE, repos='https://cran.rstudio.com')" &&
Rscript -e "library(devtools); library(methods); options(repos=c(CRAN='https://cran.rstudio.com')); devtools::install_github('IRkernel/IRkernel')" &&
Rscript -e "library(IRkernel); IRkernel::installspec(name = 'ir32', displayname = 'R 3.2')"
# Install H2O
RUN Rscript -e "install.packages('h2o', dependencies=TRUE, repos='http://cran.rstudio.com')"
# Install tensorflow fixing the proxy port
RUN pip install tensorflow-gpu
RUN Rscript -e "library(devtools); devtools::install_github('rstudio/tensorflow')"
The current image is created on top of the chstone/mxnet-gpu Docker image.
The chstone/mxnet-gpu is a docker hub repository at https://hub.docker.com/r/chstone/mxnet-gpu/.
How to do it...
Docker will all dependencies can be installed using following steps:
- Save the preceding code to a location with a name, say, Dockerfile.
- Using the command line, go to the file location and use the following command and it is also shown in the screenshot after the command:
docker run -t "TagName:FILENAME"
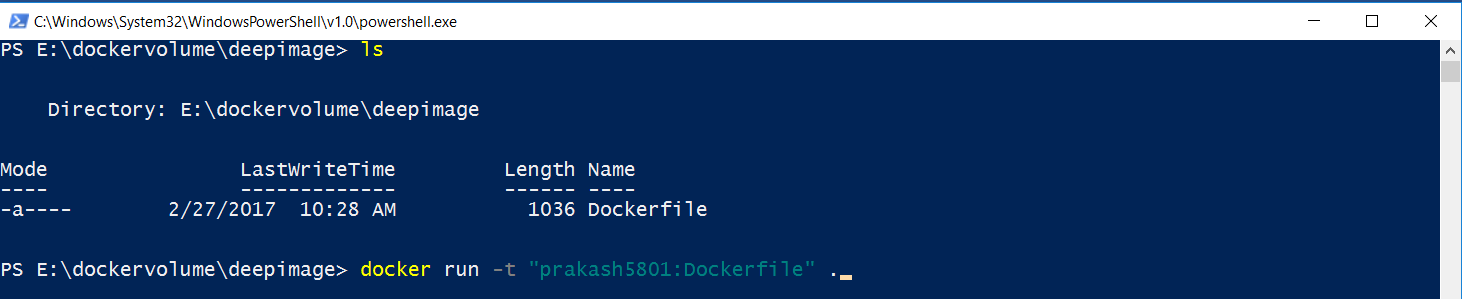
Building the docker image
- Access the image using the docker images command as follows:

View docker images
- Docker images can be executed using the following command:
docker run -it -p 8888:8888 -p 54321:54321 <<IMAGE ID>>

Running a Docker image
Here, the option -i is for interactive mode and -t is to allocate --tty. The option -p is used to forward the port. As we will be running Jupyter on port 8888 and H2O on 54321, we have forwarded both ports to accessible from the local browser.
There's more...
More options for Docker can be checked out using docker run --help.