Before delving further into big data infrastructure, let's have a look at the big data high–level landscape.
The following figure captures high–level segments that demarcate the big data space:
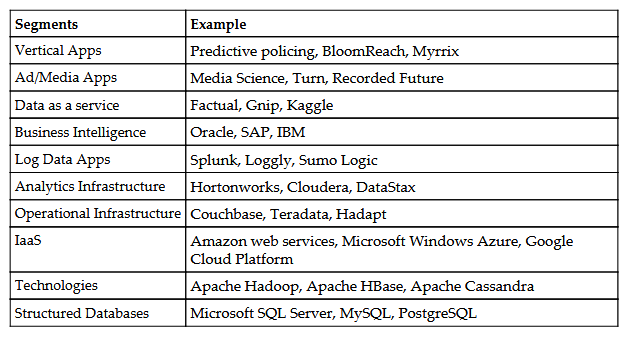
It clearly depicts the various segments and verticals within the big data technology canvas (bottom up).
The key is the bottom layer that holds the data in scalable and distributed mode:
- Technologies: Hadoop, MapReduce, Mahout, Hbase, Cassandra, and so on
- Then, the next level is the infrastructure framework layer that enables the developers to choose from myriad infrastructural offerings depending upon the use case and its solution design
- Analytical Infrastructure: EMC, Netezza, Vertica, Cloudera, Hortonworks
- Operational Infrastructure: Couchbase, Teradata, Informatica and many more
- Infrastructure as a service (IAAS): AWS, Google cloud and many more
- Structured Databases: Oracle, SQLServer, MySQL, Sybase and many more
- The next level specializes in catering to very specific needs in terms of
- Data As A Service (DaaS): Kaggale, Azure, Factual and many more
- Business Intelligence (BI): Qlikview, Cognos, SAP BO and many more
- Analytics and Visualizations: Pentaho, Tableau, Tibco and many more
Today, we see traditional robust RDBMS struggling to survive in a cost–effective manner as a tool for data storage and processing. The scaling of traditional RDBMS, at the compute power expected to process huge amount of data with low latency came at a very high price. This led to the emergence of new technologies, which were low cost, low latency, highly scalable at low cost/open source. To our rescue comes The Yellow Elephant—Hadoop that took the data storage and computation arena by surprise. It's designed and developed as a distributed framework for data storage and computation on commodity hardware in a highly reliable and scalable manner. The key computational methodology Hadoop works on involves distributing the data in chunks over all the nodes in a cluster, and then processing the data concurrently on all the nodes.
Now that you are acquainted with the basics of big data and the key segments of the big data technology landscape, let's take a deeper look at the big data concept with the Hadoop framework as an example. Then, we will move on to take a look at the architecture and methods of implementing a Hadoop cluster; this will be a close analogy to high–level infrastructure and the typical storage requirements for a big data cluster. One of the key and critical aspect that we will delve into is information security in the context of big data.
A couple of key aspects that drive and dictate the move to big data infraspace are highlighted in the following figure:
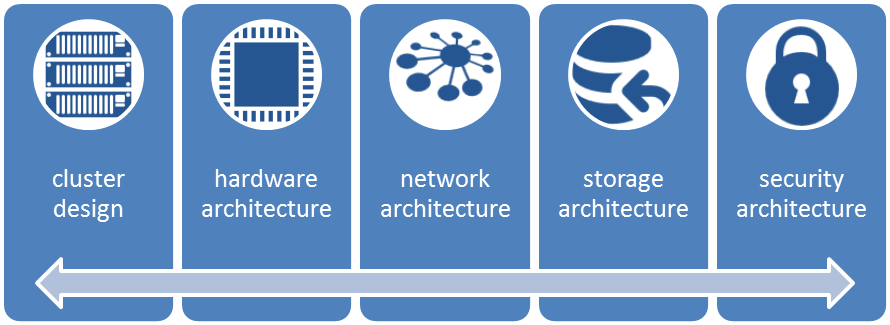
- Cluster design: This is the most significant and deciding aspect for infrastructural planning. The cluster design strategy of the infrastructure is basically the backbone of the solution; the key deciding elements for the same are the application use cases and requirements, workload, resource computation (depending upon memory intensive and compute intensive computations), and security considerations.
Apart from compute, memory, and network utilization, another very important aspect to be considered is storage which will be either cloud–based or on the premises. In terms of the cloud, the option could be public, private, or hybrid, depending upon the consideration and requirements of use case and the organization
- Hardware architecture: A lot on the storage cost aspect is driven by the volume of the data to be stored, archival policy, and the longevity of the data. The decisive factors are as follows:
- The computational needs of the implementations (whether the commodity components would suffice, or if the need is for high–performance GPUs).
- What are the memory needs? Are they low, moderate, or high? This depends upon the in–memory computation needs of the application implementations.
- Network architecture: This may not sound important, but it is a significant driver in big data computational space. The reason is that the key aspect for big data is distributed computation, and thus, network utilization is much higher than what would have been in the case of a single–server, monolithic implementation. In distributed computation, loads of data and intermediate compute results travel over the network; thus, the network bandwidth becomes the throttling agent for the overall solution and depending on key aspect for selection of infrastructure strategy. Bad design approaches sometimes lead to network chokes, where data spends less time in processing but more in shuttling across the network or waiting to be transferred over the wire for the next step in execution.
- Security architecture: Security is a very important aspect of any application space, in big data, it becomes all the more significant due to the volume and diversity of the data, and due to network traversal of the data owing to the compute methodologies. The aspect of the cloud computing and storage options adds further needs to the complexity of being a critical and strategic aspect of big data infraspace.