GCP big data and analytics services
Distinguished from storage and database services, the big data and analytics services focus on the big data processing pipeline: from data ingestion, storing, and processing to visualization, it helps you create a complete cloud-based big data infrastructure:
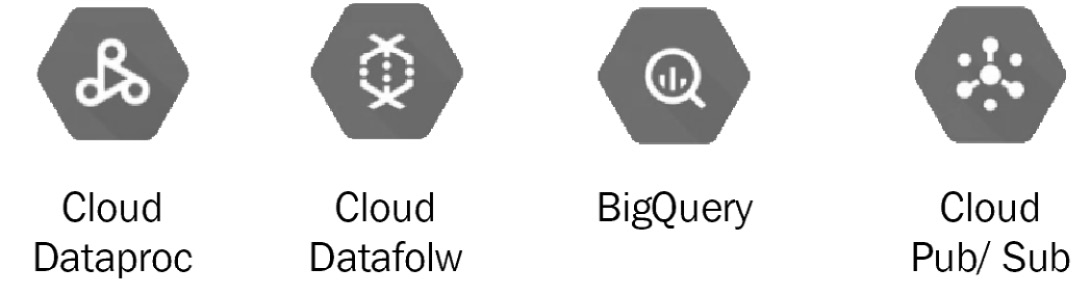
Figure 1.6 – GCP big data and analytics services
As shown in the preceding diagram, the GCP big data and analytics services include Cloud Dataproc, Cloud Dataflow, BigQuery, and Cloud Pub/Sub.
Let’s examine each of them briefly.
Google Cloud Dataproc
Based on the concept of Map-Reduce and the architecture of Hadoop systems, Google Cloud Dataproc is a managed GCP service for processing large datasets. Dataproc provides organizations with the flexibility to provision and configure data processing clusters of varying sizes on demand. Dataproc integrates well with other GCP services. It can operate directly on Cloud Storage files or use Bigtable to analyze data, and it can be integrated with Vertex AI, BigQuery, Dataplex, and other GCP services.
Dataproc helps users process, transform, and understand vast quantities of data. You can use Dataproc to run Apache Spark, Apache Flink, Presto, and 30+ open source tools and frameworks. You can also use Dataproc for data lake modernization, ETL processes, and more.
Google Cloud Dataflow
Cloud Dataflow is a GCP-managed service for developing and executing a wide variety of data processing patterns, including Extract, Transform, Load (ETL), batch, and streaming jobs. Cloud Dataflow is a serverless data processing service that runs jobs written with Apache Beam libraries. Cloud Dataflow executes jobs that consist of a pipeline – a sequence of steps that reads data, transforms it into different formats, and writes it out. A dataflow pipeline consists of a series of pipes, which is a way to connect components, where data moves from one component to the next via a pipe. When jobs are executed on Cloud Dataflow, the service spins up a cluster of VMs, distributes the job tasks to the VMs, and dynamically scales the cluster based on job loads and their performance.
Google Cloud BigQuery
BigQuery is a Google fully managed enterprise data warehouse service that is highly scalable, fast, and optimized for data analytics. It has the following features:
- BigQuery supports ANSI-standard SQL queries, including joins, nested and repeated fields, analytic and aggregation functions, scripting, and a variety of spatial functions via geospatial analytics.
- With BigQuery, you do not physically manage the infrastructure assets. BigQuery’s serverless architecture lets you use SQL queries to answer big business questions with zero infrastructure overhead. With BigQuery’s scalable, distributed analysis engine, you can query petabytes of data in minutes.
- BigQuery integrates seamlessly with other GCP data services. You can query data stored in BigQuery or run queries on data where it lives using external tables or federated queries, including GCS, Bigtable, Spanner, or Google Sheets stored in Google Drive.
- BigQuery helps you manage and analyze your data with built-in features such as ML, geospatial analysis, and business intelligence. We will discuss BigQuery ML later in this book.
Google BigQuery is used in many business cases due to it being SQL-friendly, having a serverless structure, and having built-in integration with other GCP services.
Google Cloud Pub/Sub
GCP Pub/Sub is a widely used cloud service for decoupling many GCP services – it implements an event/message queue pipe to integrate services and parallelize tasks. With the Pub/Sub service, you can create event producers, called publishers, and event consumers, called subscribers. Using Pub/Sub, the publishers communicate with subscribers asynchronously by broadcasting events – a publisher can have multiple subscribers and a subscriber can subscribe to multiple publishers:
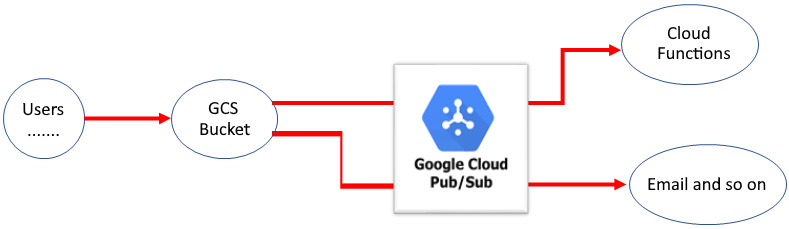
Figure 1.7 – Google Cloud Pub/Sub services
The preceding diagram shows the example we discussed in the GCP Cloud Functions section: after an object is uploaded to a GCS bucket, a request/message can be generated and sent to GCP Pub/Sub, which can trigger an email notification and a cloud function to process the object. When the number of parallel object uploads is huge, Cloud Pub/Sub will help buffer/queue the requests/messages and decouple the GCS service from other cloud services such as Cloud Functions.
So far, we have covered various GCP services, including compute, storage, databases, and data analytics (big data). Now, let’s take a look at various GCP artificial intelligence (AI) services.