In this chapter, we will discuss the concept of soft clustering, which allows us to obtain a membership degree for each sample of a dataset with respect to a defined cluster configuration. That is, considering a range from 0% to 100%, we want to know to what extent xi belong to a cluster. The extreme values are 0, which means that xi is completely outside the domain of the cluster and 1 (100%), indicating that xi is fully assigned to a single cluster. All intermediate values imply a partial domain of two or more different clusters. Therefore, in contrast with hard clustering, here, we are interested in determining not a fixed assignment, but a vector with the same properties of a probability distribution (or a probability itself). Such an approach allows having better control over borderline samples and helps us in finding out a suitable...




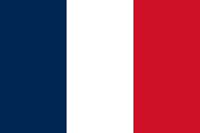


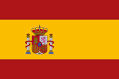



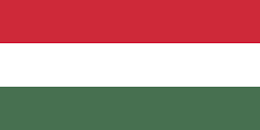

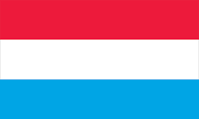





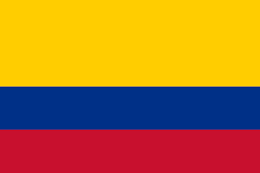
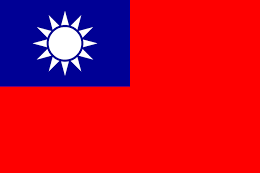
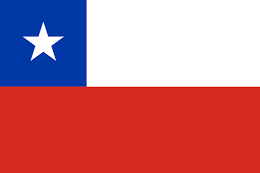



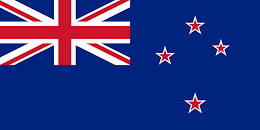
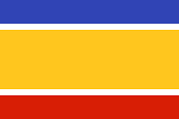
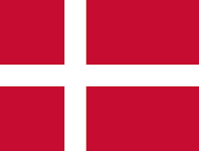
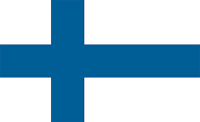







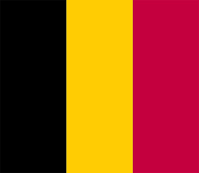

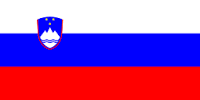





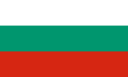


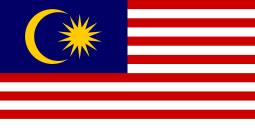



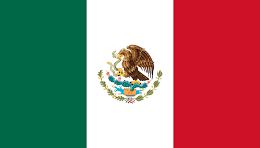
