Training loss comparison
During training, the learning rate might be strong after a certain number of epochs for fine-tuning. Decreasing the learning rate when the loss does not decrease anymore will help during the last steps of training. To decrease the learning rate, we need to define it as an input variable during compilation:
lr = T.scalar('learning_rate') train_model = theano.function(inputs=[x,y,lr], outputs=cost,updates=updates)
During training, we adjust the learning rate, decreasing it if the training loss is not better:
if (len(train_loss) > 1 and train_loss[-1] > train_loss[-2]): learning_rate = learning_rate * 0.5
As a first experiment, let's see the impact of the size of the hidden layer on the training loss for a simple RNN:
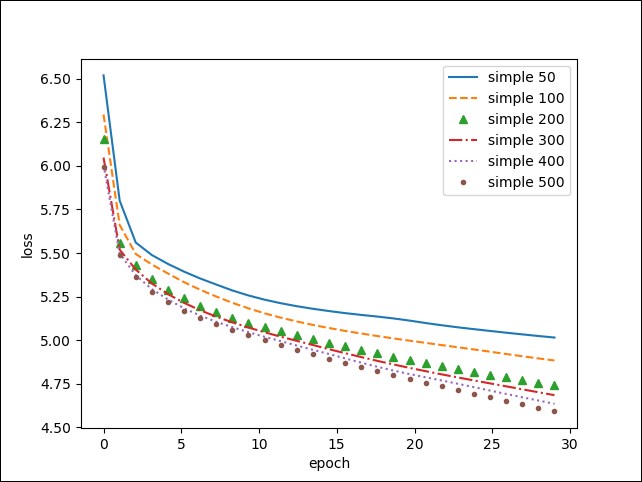
More hidden units improve training speed and might be better in the end. To check this, we should run it for more epochs.
Comparing the training of the different network types, in this case, we do not observe any improvement with LSTM and GRU:
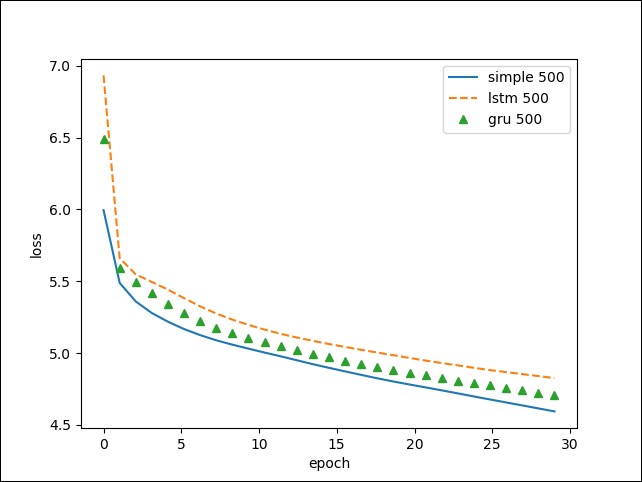
This...