



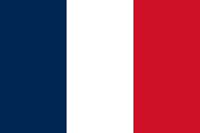


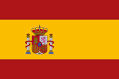



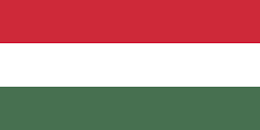

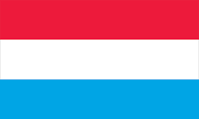





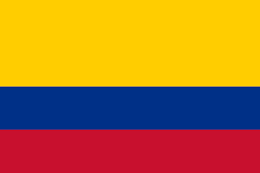
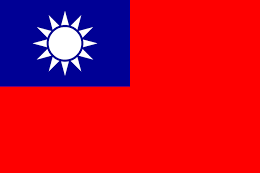
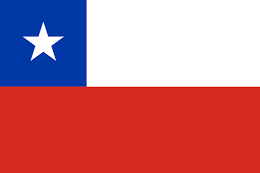



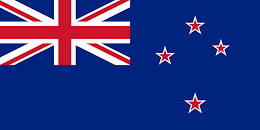
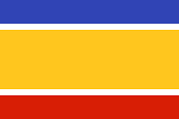
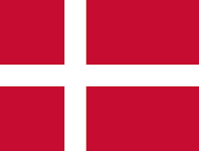
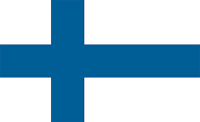







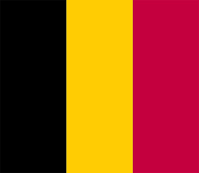

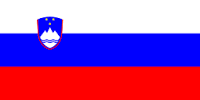





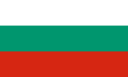


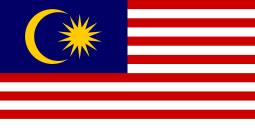



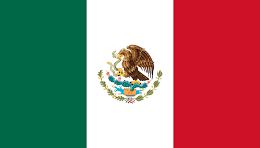

In this article, Pradeepta Mishra, the author of R Data Mining Blueprints, says that in this age of Internet, everything available over the Internet is not useful for everyone. Different companies and entities use different approaches in finding out relevant content for their audiences. People started building algorithms to construct relevance score, based on that, recommendation can be build and suggested to the users. From our day to day life, every time I see an image on Google, 3-4 other images are recommended to me by Google. Every time I look for some videos on YouTube, 10 more videos are recommended to me. Every time I visit Amazon to buy some products, 5-6 products are recommended to me. And every time I read one blog or article, a few more articles and blogs are recommended to me. This is an evidence of algorithmic forces at play to recommend certain things based on users’ preferences or choices, since the users’ time is precious and content available over the Internet is unlimited. Hence, a recommendation engine helps organizations customize their offerings based on user preferences so that the user need not have to spend time in exploring what is required.
In this article, the reader will learn the implementation of product recommendation using R.
(For more resources related to this topic, see here.)
The dataset contains a sample of 5000 users from the anonymous ratings data from the Jester Online Joke Recommender System collected between April 1999 and May 2003 (Golberg, Roeder, Gupta, and Perkins 2001). The dataset contains ratings for 100 jokes on a scale from -10 to 10. All users in the dataset have rated 36 or more jokes. Let's load the recommenderlab library and the Jester5K dataset:
> library("recommenderlab")
> data(Jester5k)
> Jester5k@data@Dimnames[2]
[[1]]
[1] "j1" "j2" "j3" "j4" "j5" "j6" "j7" "j8" "j9"
[10] "j10" "j11" "j12" "j13" "j14" "j15" "j16" "j17" "j18"
[19] "j19" "j20" "j21" "j22" "j23" "j24" "j25" "j26" "j27"
[28] "j28" "j29" "j30" "j31" "j32" "j33" "j34" "j35" "j36"
[37] "j37" "j38" "j39" "j40" "j41" "j42" "j43" "j44" "j45"
[46] "j46" "j47" "j48" "j49" "j50" "j51" "j52" "j53" "j54"
[55] "j55" "j56" "j57" "j58" "j59" "j60" "j61" "j62" "j63"
[64] "j64" "j65" "j66" "j67" "j68" "j69" "j70" "j71" "j72"
[73] "j73" "j74" "j75" "j76" "j77" "j78" "j79" "j80" "j81"
[82] "j82" "j83" "j84" "j85" "j86" "j87" "j88" "j89" "j90"
[91] "j91" "j92" "j93" "j94" "j95" "j96" "j97" "j98" "j99"
[100] "j100"
The following image shows the distribution of real ratings given by 2000 users.
> data<-sample(Jester5k,2000)
> hist(getRatings(data),breaks=100,col="blue")
The input dataset contains the individual ratings; the normalization function reduces the individual rating bias by centering the row (which is a standard z-score transformation), subtracting each element from the mean, and then dividing by standard deviation. The following graph shows normalized ratings for the preceding dataset:
> hist(getRatings(normalize(data)),breaks=100,col="blue4")
To create a recommender system:
A recommendation engine is created using the recommender() function. A new recommendation algorithm can be added by the user using the recommenderRegistry$get_entries() function:
> recommenderRegistry$get_entries(dataType = "realRatingMatrix")
$IBCF_realRatingMatrix
Recommender method: IBCF
Description: Recommender based on item-based collaborative filtering (real data).
Parameters:
k method normalize normalize_sim_matrix alpha na_as_zero minRating
1 30 Cosine center FALSE 0.5 FALSE NA
$POPULAR_realRatingMatrix
Recommender method: POPULAR
Description: Recommender based on item popularity (real data).
Parameters: None
$RANDOM_realRatingMatrix
Recommender method: RANDOM
Description: Produce random recommendations (real ratings).
Parameters: None
$SVD_realRatingMatrix
Recommender method: SVD
Description: Recommender based on SVD approximation with column-mean imputation (real data).
Parameters:
k maxiter normalize minRating
1 10 100 center NA
$SVDF_realRatingMatrix
Recommender method: SVDF
Description: Recommender based on Funk SVD with gradient descend (real data).
Parameters:
k gamma lambda min_epochs max_epochs min_improvement normalize
1 10 0.015 0.001 50 200 1e-06 center
minRating verbose
1 NA FALSE
$UBCF_realRatingMatrix
Recommender method: UBCF
Description: Recommender based on user-based collaborative filtering (real data).
Parameters:
method nn sample normalize minRating
1 cosine 25 FALSE center NA
The preceding registry command helps in identifying the methods available in the recommenderlab parameters for the model.
There are six different methods for implementing recommender systems, such as popular, item-based, user-based, PCA, random, and SVD. Let's start the recommendation engine using the popular method:
> rc <- Recommender(Jester5k, method = "POPULAR")
> rc
Recommender of type 'POPULAR' for 'realRatingMatrix'
learned using 5000 users.
> names(getModel(rc))
[1] "topN" "ratings"
[3] "minRating" "normalize"
[5] "aggregationRatings" "aggregationPopularity"
[7] "minRating" "verbose"
> getModel(rc)$topN
Recommendations as 'topNList' with n = 100 for 1 users.
The objects such as top N, verbose, aggregation popularity, and so on, can be printed using names of the getmodel()command:
recom <- predict(rc, Jester5k, n=5)
recom
To generate a recommendation, we can use the predict function against the same dataset and validate the accuracy of the predictive model. Here we are generating the top 5 recommended jokes to each of the users. The result of the prediction is as follows:
> head(as(recom,"list"))
$u2841
[1] "j89" "j72" "j76" "j88" "j83"
$u15547
[1] "j89" "j93" "j76" "j88" "j91"
$u15221
character(0)
$u15573
character(0)
$u21505
[1] "j89" "j72" "j93" "j76" "j88"
$u15994
character(0)
For the same Jester5K dataset, let's try to implement item-based collaborative filtering (IBCF):
> rc <- Recommender(Jester5k, method = "IBCF")
> rc
Recommender of type 'IBCF' for 'realRatingMatrix'
learned using 5000 users.
> recom <- predict(rc, Jester5k, n=5)
> recom
Recommendations as 'topNList' with n = 5 for 5000 users.
> head(as(recom,"list"))
$u2841
[1] "j85" "j86" "j74" "j84" "j80"
$u15547
[1] "j91" "j87" "j88" "j89" "j93"
$u15221
character(0)
$u15573
character(0)
$u21505
[1] "j78" "j80" "j73" "j77" "j92"
$u15994
character(0)
The Principal component analysis (PCA) method is not applicable for real-rating-based datasets; this is because getting a correlation matrix and subsequent eigenvector and eigenvalue calculations would not be accurate. Hence we will not show its application. Next we are going to show how the random method works:
> rc <- Recommender(Jester5k, method = "RANDOM")
> rc
Recommender of type 'RANDOM' for 'ratingMatrix'
learned using 5000 users.
> recom <- predict(rc, Jester5k, n=5)
> recom
Recommendations as 'topNList' with n = 5 for 5000 users.
> head(as(recom,"list"))
[[1]]
[1] "j90" "j74" "j86" "j78" "j85"
[[2]]
[1] "j87" "j88" "j74" "j92" "j79"
[[3]]
character(0)
[[4]]
character(0)
[[5]]
[1] "j95" "j86" "j93" "j78" "j83"
[[6]]
character(0)
In the recommendation engine, the SVD approach is used to predict the missing ratings so that a recommendation can be generated. Using the singular value decomposition (SVD) method, the following recommendation can be generated:
> rc <- Recommender(Jester5k, method = "SVD")
> rc
Recommender of type 'SVD' for 'realRatingMatrix'
learned using 5000 users.
> recom <- predict(rc, Jester5k, n=5)
> recom
Recommendations as 'topNList' with n = 5 for 5000 users.
> head(as(recom,"list"))
$u2841
[1] "j74" "j71" "j84" "j79" "j80"
$u15547
[1] "j89" "j93" "j76" "j81" "j88"
$u15221
character(0)
$u15573
character(0)
$u21505
[1] "j80" "j73" "j100" "j72" "j78"
$u15994
character(0)
The result from user-based collaborative filtering is shown as follows:
> rc <- Recommender(Jester5k, method = "UBCF")
> rc
Recommender of type 'UBCF' for 'realRatingMatrix'
learned using 5000 users.
> recom <- predict(rc, Jester5k, n=5)
> recom
Recommendations as 'topNList' with n = 5 for 5000 users.
> head(as(recom,"list"))
$u2841
[1] "j81" "j78" "j83" "j80" "j73"
$u15547
[1] "j96" "j87" "j89" "j76" "j93"
$u15221
character(0)
$u15573
character(0)
$u21505
[1] "j100" "j81" "j83" "j92" "j96"
$u15994
character(0)
Now let's compare the results obtained from all the five different algorithms except PCA (because PCA requires a binary dataset; it does not accept a real ratings matrix).
Table 4: Comparison of results between different recommendation algorithms
Popular |
IBCF |
Random method |
SVD |
UBCF |
|
> head(as(recom,"list")) |
> head(as(recom,"list")) |
> head(as(recom,"list")) |
> head(as(recom,"list")) |
> head(as(recom,"list")) |
|
$u2841 |
$u2841 |
[[1]] |
$u2841 |
$u2841 |
|
[1] "j89" "j72" "j76" "j88" "j83" |
[1] "j85" "j86" "j74" "j84" "j80" |
[1] "j90" "j74" "j86" "j78" "j85" |
[1] "j74" "j71" "j84" "j79" "j80" |
[1] "j81" "j78" "j83" "j80" "j73" |
|
|
|
|
|
|
|
$u15547 |
$u15547 |
[[2]] |
$u15547 |
$u15547 |
|
[1] "j89" "j93" "j76" "j88" "j91" |
[1] "j91" "j87" "j88" "j89" "j93" |
[1] "j87" "j88" "j74" "j92" "j79" |
[1] "j89" "j93" "j76" "j81" "j88" |
[1] "j96" "j87" "j89" "j76" "j93" |
|
|
|
|
|
|
|
$u15221 |
$u15221 |
[[3]] |
$u15221 |
$u15221 |
|
character(0) |
character(0) |
character(0) |
character(0) |
character(0) |
|
|
|
|
|
|
|
$u15573 |
$u15573 |
[[4]] |
$u15573 |
$u15573 |
|
character(0) |
character(0) |
character(0) |
character(0) |
character(0) |
|
|
|
|
|
|
|
$u21505 |
$u21505 |
[[5]] |
$u21505 |
$u21505 |
|
[1] "j89" "j72" "j93" "j76" "j88" |
[1] "j78" "j80" "j73" "j77" "j92" |
[1] "j95" "j86" "j93" "j78" "j83" |
[1] "j80" "j73" "j100" "j72" "j78" |
[1] "j100" "j81" "j83" "j92" "j96" |
|
|
|
|
|
|
|
$u15994 |
$u15994 |
[[6]] |
$u15994 |
$u15994 |
|
character(0) |
character(0) |
character(0) |
character(0) |
character(0) |
|
One thing is clear from the above table. For users 15573 and 15221, none of the five methods generate recommendation. Hence it is important to look at methods to evaluate the recommendation results. To validate the accuracy of the model, let's implement accuracy measures and compare the accuracies of all the models.
For the evaluation of the model results, the dataset is divided into 90% for training and 10% for testing the algorithm. The definition of a good rating is updated as 5:
> e <- evaluationScheme(Jester5k, method="split",
+ train=0.9,given=15, goodRating=5)
> e
Evaluation scheme with 15 items given
Method: 'split' with 1 run(s).
Training set proportion: 0.900
Good ratings: >=5.000000
Data set: 5000 x 100 rating matrix of class 'realRatingMatrix' with 362106 ratings.
The following script is used to build the collaborative filtering model and apply it on a new dataset for predicting the ratings. Then the prediction accuracy is computed. The error matrix is shown as follows:
> #User based collaborative filtering
> r1 <- Recommender(getData(e, "train"), "UBCF")
> #Item based collaborative filtering
> r2 <- Recommender(getData(e, "train"), "IBCF")
> #PCA based collaborative filtering
> #r3 <- Recommender(getData(e, "train"), "PCA")
> #POPULAR based collaborative filtering
> r4 <- Recommender(getData(e, "train"), "POPULAR")
> #RANDOM based collaborative filtering
> r5 <- Recommender(getData(e, "train"), "RANDOM")
> #SVD based collaborative filtering
> r6 <- Recommender(getData(e, "train"), "SVD")
> #Predicted Ratings
> p1 <- predict(r1, getData(e, "known"), type="ratings")
> p2 <- predict(r2, getData(e, "known"), type="ratings")
> #p3 <- predict(r3, getData(e, "known"), type="ratings")
> p4 <- predict(r4, getData(e, "known"), type="ratings")
> p5 <- predict(r5, getData(e, "known"), type="ratings")
> p6 <- predict(r6, getData(e, "known"), type="ratings")
> #calculate the error between the prediction and
> #the unknown part of the test data
> error <- rbind(
+ calcPredictionAccuracy(p1, getData(e, "unknown")),
+ calcPredictionAccuracy(p2, getData(e, "unknown")),
+ #calcPredictionAccuracy(p3, getData(e, "unknown")),
+ calcPredictionAccuracy(p4, getData(e, "unknown")),
+ calcPredictionAccuracy(p5, getData(e, "unknown")),
+ calcPredictionAccuracy(p6, getData(e, "unknown"))
+ )
> rownames(error) <- c("UBCF","IBCF","POPULAR","RANDOM","SVD")
> error
RMSE MSE MAE
UBCF 4.485571 20.12034 3.511709
IBCF 4.606355 21.21851 3.466738
POPULAR 4.509973 20.33985 3.548478
RANDOM 7.917373 62.68480 6.464369
SVD 4.653111 21.65144 3.679550
From the preceding result, UBCF has the lowest error in comparison to other recommendation methods. Here, to evaluate the results of the predictive model, we are using the k-fold cross-validation method. k is assumed to have been taken as 4:
> #Evaluation of a top-N recommender algorithm
> scheme <- evaluationScheme(Jester5k, method="cross", k=4,
+ given=3,goodRating=5)
> scheme
Evaluation scheme with 3 items given
Method: 'cross-validation' with 4 run(s).
Good ratings: >=5.000000
Data set: 5000 x 100 rating matrix of class 'realRatingMatrix' with 362106 ratings.
The result of the models from the evaluation scheme shows the runtime versus prediction time by different cross-validation results for different models. The result is shown as follows:
> results <- evaluate(scheme, method="POPULAR", n=c(1,3,5,10,15,20))
POPULAR run fold/sample [model time/prediction time]
1 [0.14sec/2.27sec]
2 [0.16sec/2.2sec]
3 [0.14sec/2.24sec]
4 [0.14sec/2.23sec]
> results <- evaluate(scheme, method="IBCF", n=c(1,3,5,10,15,20))
IBCF run fold/sample [model time/prediction time]
1 [0.4sec/0.38sec]
2 [0.41sec/0.37sec]
3 [0.42sec/0.38sec]
4 [0.43sec/0.37sec]
> results <- evaluate(scheme, method="UBCF", n=c(1,3,5,10,15,20))
UBCF run fold/sample [model time/prediction time]
1 [0.13sec/6.31sec]
2 [0.14sec/6.47sec]
3 [0.15sec/6.21sec]
4 [0.13sec/6.18sec]
> results <- evaluate(scheme, method="RANDOM", n=c(1,3,5,10,15,20))
RANDOM run fold/sample [model time/prediction time]
1 [0sec/0.27sec]
2 [0sec/0.26sec]
3 [0sec/0.27sec]
4 [0sec/0.26sec]
> results <- evaluate(scheme, method="SVD", n=c(1,3,5,10,15,20))
SVD run fold/sample [model time/prediction time]
1 [0.36sec/0.36sec]
2 [0.35sec/0.36sec]
3 [0.33sec/0.36sec]
4 [0.36sec/0.36sec]
The confusion matrix displays the level of accuracy provided by each of the models. We can estimate the accuracy measures such as precision, recall and TPR, FPR, and so on; the result is shown here:
> getConfusionMatrix(results)[[1]]
TP FP FN TN precision recall TPR FPR
1 0.2736 0.7264 17.2968 78.7032 0.2736000 0.01656597 0.01656597 0.008934588
3 0.8144 2.1856 16.7560 77.2440 0.2714667 0.05212659 0.05212659 0.027200530
5 1.3120 3.6880 16.2584 75.7416 0.2624000 0.08516269 0.08516269 0.046201487
10 2.6056 7.3944 14.9648 72.0352 0.2605600 0.16691259 0.16691259 0.092274243
15 3.7768 11.2232 13.7936 68.2064 0.2517867 0.24036802 0.24036802 0.139945095
20 4.8136 15.1864 12.7568 64.2432 0.2406800 0.30082509 0.30082509 0.189489883
Association rules as a method for recommendation engine, for building product recommendation in a retail/e-commerce scenario.
In this article, we discussed the way of recommending products to users based on similarities in their purchase patterns, content, item-to-item comparison and so on. So far, the accuracy is concerned, always the user-based collaborative filtering is giving better result in a real-rating-based matrix as an input. Similarly, the choice of methods for a specific use case is really difficult, so it is recommended to apply all six different methods. The best one should be selected automatically, and the recommendation should also get updates automatically.
Further resources on this subject: