



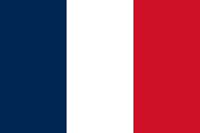


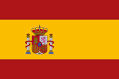



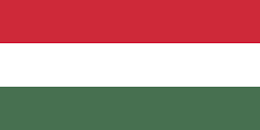

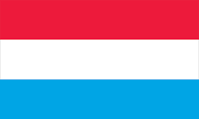





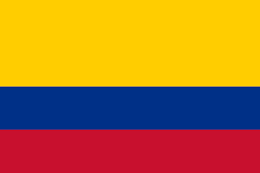
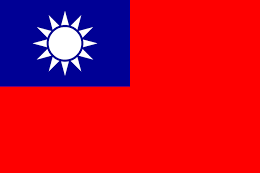
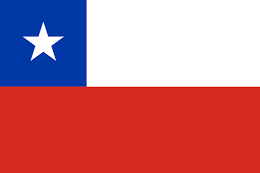



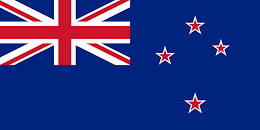
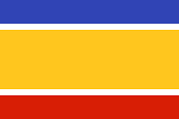
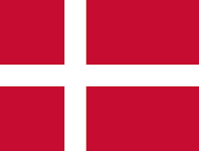
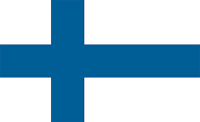







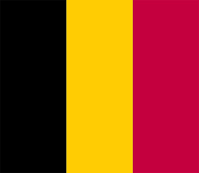

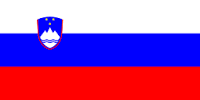





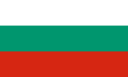


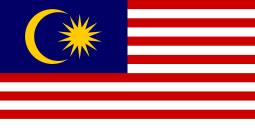



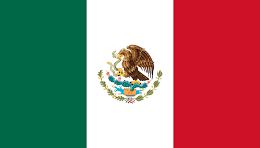

Dive deeper into the world of AI innovation and stay ahead of the AI curve! Subscribe to our AI_Distilled newsletter for the latest insights. Don't miss out – sign up today!
This article is an excerpt from the book, Modern Generative AI with ChatGPT and OpenAI Models, by Valentina Alto. Master core data architecture design concepts and Azure Data & AI services to gain a cloud data and AI architect’s perspective to developing end-to-end solutions.
In the ever-evolving landscape of digital marketing, staying competitive and meeting customer expectations is paramount. This article explores the revolutionary potential of ChatGPT in enhancing multiple aspects of marketing. From refining A/B testing strategies to elevating SEO optimization techniques and harnessing sentiment analysis for measuring customer satisfaction, ChatGPT emerges as a pivotal tool.
Another interesting field where ChatGPT can assist marketers is A/B testing.
A/B testing in marketing is a method of comparing two different versions of a marketing campaign, advertisement, or website to determine which one performs better. In A/B testing, two variations of the same campaign or element are created, with only one variable changed between the two versions. The goal is to see which version generates more clicks, conversions, or other desired outcomes.
An example of A/B testing might be testing two versions of an email campaign, using different subject lines, or testing two versions of a website landing page, with different call-to-action buttons. By measuring the response rate of each version, marketers can determine which version performs better and make data-driven decisions about which version to use going forward.
A/B testing allows marketers to optimize their campaigns and elements for maximum effectiveness, leading to better results and a higher return on investment.
Since this method involves the process of generating many variations of the same content, the generative power of ChatGPT can definitely assist in that.
Let’s consider the following example. I’m promoting a new product I developed: a new, light and thin climbing harness for speed climbers. I’ve already done some market research and I know my niche audience. I also know that one great channel of communication for that audience is publishing on an online climbing blog, of which most climbing gyms’ members are fellow readers.
My goal is to create an outstanding blog post to share the launch of this new harness, and I want to test two different versions of it in two groups. The blog post I’m about to publish and that I want to be the object of my A/B testing is the following:
Figure – An example of a blog post to launch climbing gear
Here, ChatGPT can help us on two levels:
Figure – New version of the blog post generated by ChatGPT
As per my request, ChatGPT was able to regenerate only those elements I asked for (title, subtitle, and closing sentence) so that I can monitor the effectiveness of those elements by monitoring the reaction of the two audience groups.
Figure – Sample blog post published on the climbing blog
We can directly feed ChatGPT with the HTML code and ask it to change some layout elements, such as the position of the buttons or their wording. For example, rather than Buy Now, a reader might be more gripped by an I want one! button.
So, lets feed ChatGPT with the HTML source code:
Figure – ChatGPT changing HTML code
Let’s see what the output looks like:
Figure – New version of the website
As you can see, ChatGPT only intervened at the button level, slightly changing their layout, position, color, and wording.
Indeed, inspecting the source code of the two versions of the web pages, we can see how it differs in the button sections:
Figure – Comparison between the source code of the two versions of the website
ChatGPT is a valuable tool for A/B testing in marketing. Its ability to quickly generate different versions of the same content can reduce the time to market of new campaigns. By utilizing ChatGPT for A/B testing, you can optimize your marketing strategies and ultimately drive better results for your business.
Valentina Alto graduated in 2021 in data science. Since 2020, she has been working at Microsoft as an Azure solution specialist, and since 2022, she has been focusing on data and AI workloads within the manufacturing and pharmaceutical industry. She has been working closely with system integrators on customer projects to deploy cloud architecture with a focus on modern data platforms, data mesh frameworks, IoT and real-time analytics, Azure Machine Learning, Azure Cognitive Services (including Azure OpenAI Service), and Power BI for dashboarding. Since commencing her academic journey, she has been writing tech articles on statistics, machine learning, deep learning, and AI in various publications and has authored a book on the fundamentals of machine learning with Python.