Suppose you are going to build a machine learning model that will predict whether a credit card transaction is fraudulent or not. Now, based on the available background knowledge and data analysis, you might decide which data fields (aka features) are important for training your model. For example, amount, customer name, buying company name, and the address of the credit card owners are worth to providing for the overall learning process. These are important to consider since, if you just provide a randomly generated transaction ID, that will not carry any information so would not be useful at all. Thus, once you have decided which features to include in your training set, you then need to transform those features to train the model for better learning. The feature transformations help you add additional background information to the training...




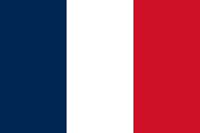


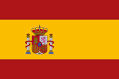



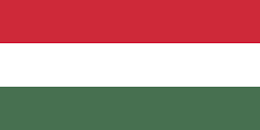

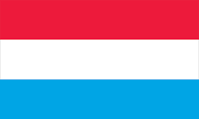





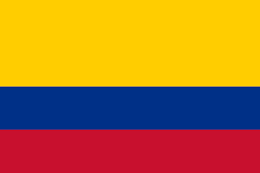
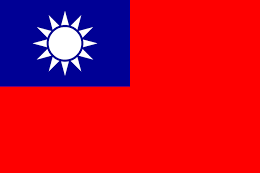
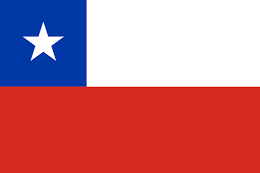



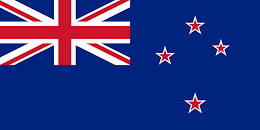
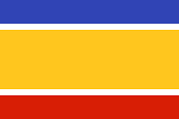
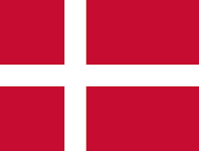
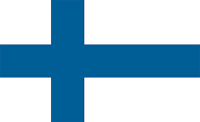







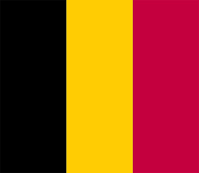

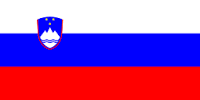





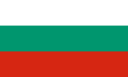


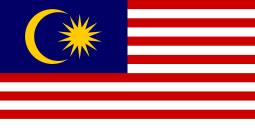



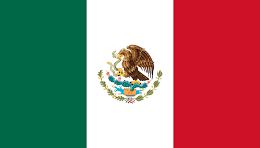
