Implementing the graph attention layer in NumPy
As previously stated, neural networks work in terms of matrix multiplications. Therefore, we need to translate our individual embeddings into operations for the entire graph. In this section, we will implement the original graph attention layer from scratch to properly understand the inner workings of self-attention. Naturally, this process can be repeated several times to create multi-head attention.
The first step consists of translating the original graph attention operator in terms of matrices. This is how we defined it in the last section:
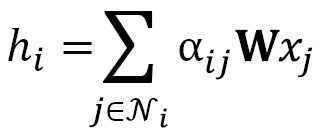
By taking inspiration from the graph linear layer, we can write the following:

Where is a matrix that stores every
.
In this example, we will use the following graph from the previous chapter:
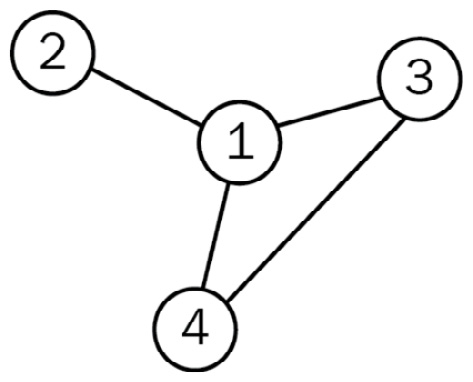
Figure 7.3 – Simple graph where nodes have different numbers of neighbors
The graph must provide two important...