In this chapter, we dug in and learned more of the inner workings of RL by understanding the differences between model-based versus off-model and/or policy-based algorithms. As we learned, Unity ML-Agents uses the PPO algorithm, a powerful and flexible policy learning model that works exceptionally well when training control, or what is sometimes referred to as marathon RL. After learning more basics, we jumped into other RL improvements in the form of Actor-Critic, or advantage training, and what options ML-Agents supports. Next, we looked at the evolution of PPO and its predecessor, the TRPO algorithm, how they work at a basic level, and how they affect training. This is where we learned how to modify one of the control samples to create a new joint on the Reacher arm. We finished the chapter by looking at how multi-agent policy training can be improved on, again by...




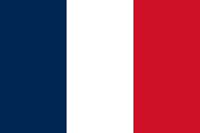


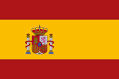




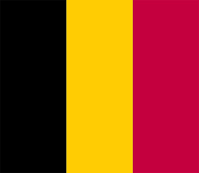
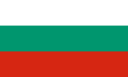
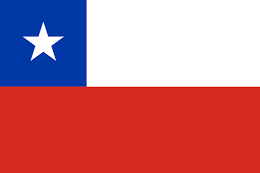
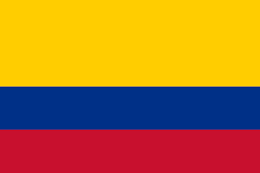
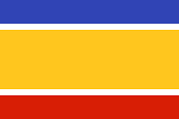

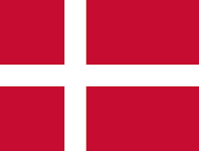



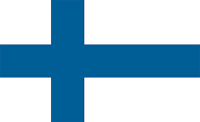

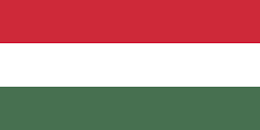






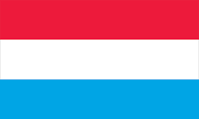
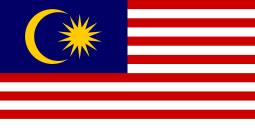

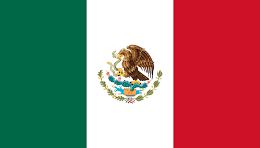

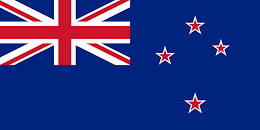







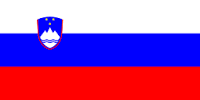




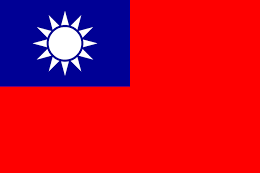


