In this section, we will look at some of the most popular frameworks regarding deep learning. In short, almost all libraries provide the possibility of using the graphics processor to speed up the learning process, and are released under an open license and are the result of implementation by university research groups.Before starting the comparison, refer figure 13 which is one of the most complete charts of neural networks till date. If you see the URL and related papers, you will find that the idea of neural networks is pretty old and the software frameworks that we are going to compare below also adapts similar architecture during their framework development:
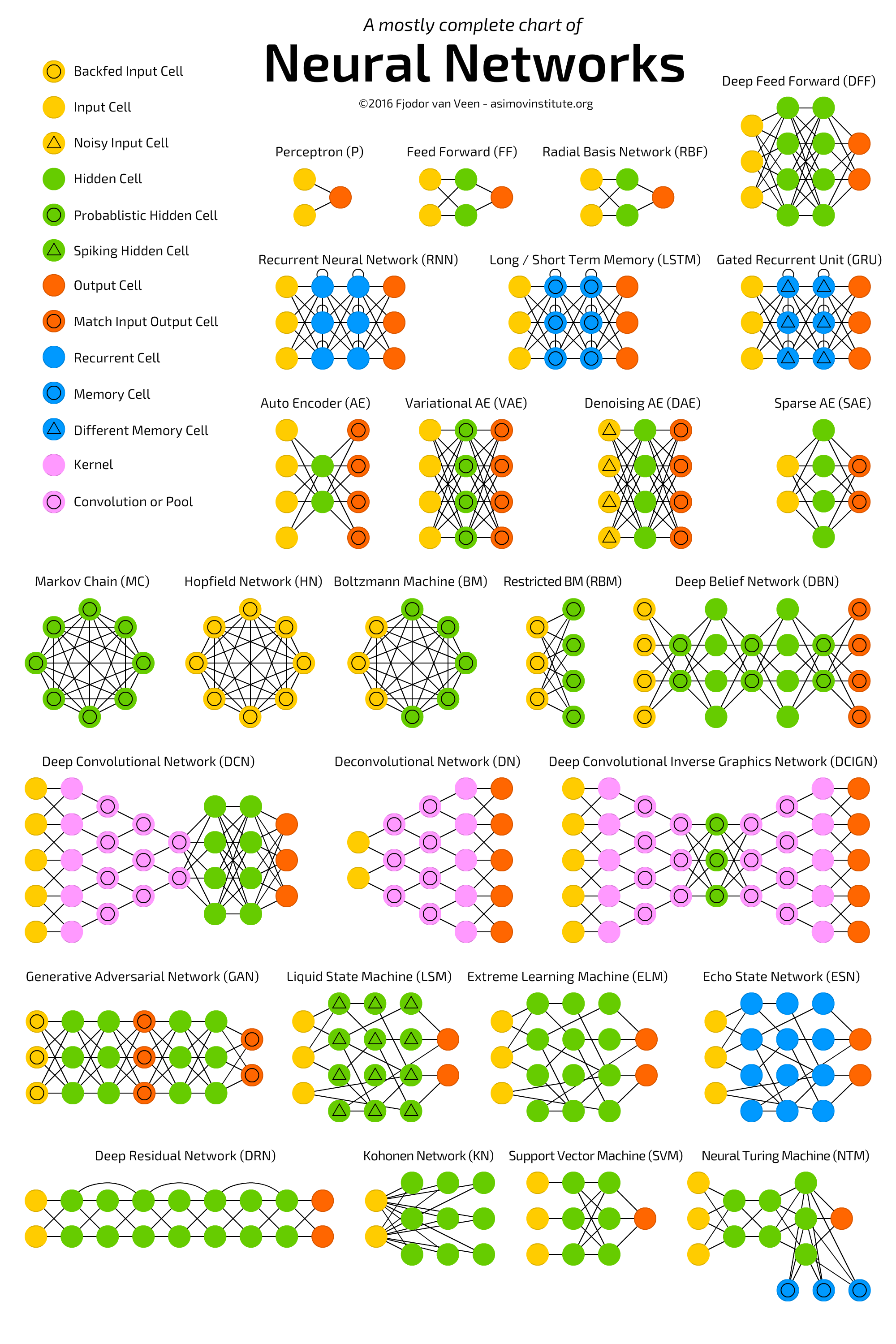
Figure 13: complete charts of neural networks (Source: http://www.asimovinstitute.org/neural-network-zoo/)
- Theano: This is probably the most widespread library. Written in Python, one of the languages most used in the field of machine learning (also in TensorFlow), allows the calculation also GPU, coming to have 24x the performance of the CPU. It allows you to define, optimize, and evaluate complex mathematical expressions such as multidimensional arrays.
- Caffe: This was developed primarily by Berkeley Vision and Learning Center (BVLC), and is a framework designed to stand out in terms of expression, speed, and modularity. Its unique architecture encourages application and innovation by allowing easier transition from calculation of CPU to GPU. The large community of users allowed for considerable development in recent times. It is written in Python, but the installation process can be long due to the numerous support libraries to compile.
- Torch: This is a vast ecosystem for machine learning which offers a large number of algorithms and functions, including those for deep learning and processing of various types of multimedia, such as audio and video data, with particular attention to parallel computing. It provides an excellent interface for the C language and has a large community of users. Torch is a library that extends the scripting language Lua, and is intended to provide a flexible environment for designing and training machine learning systems. Torch is a self-contained and highly portable framework on each platform (for example, Windows, Mac, Linux, and Android) and scripts manage to run on these platforms without modification. The Torch package provides many useful features for different applications.
Finally, the following table provides a summary of each framework's salient features, including TensorFlow, which will be described (of course!!) in the coming chapters:
TensorFlow | Torch | Caffe | Theano | |
Programming language used |
Python and C++ | Lua | C++ | Python |
GPU card support | Yes | Yes | Yes | By default, no |
Pros |
|
|
|
|
Cons |
|
|
|
|