Chapter 5: Foundations of Recurrent Neural Network
Activity 6: Solve a problem with RNN – Author Attribution
Solution:
Prepare the data
We begin by setting up the data pre-processing pipeline. For each one of the authors, we aggregate all the known papers into a single long text. We assume that style does not change across the various papers, hence a single text is equivalent to multiple small ones yet it is much easier to deal with programmatically.
For each paper of each author we perform the following steps:
- Convert all text into lower-case (ignoring the fact that capitalization may be a stylistic property)
- Converting all newlines and multiple whitespaces into single whitespaces
- Remove any mention of the authors' names, otherwise we risk data leakage (authors names are hamilton and madison)
- Do the above steps in a function as it is needed for predicting the unknown papers.
import numpy as np
import os
from sklearn.model_selection import train_test_split
# Classes for A/B/Unknown
A = 0
B = 1
UNKNOWN = -1
def preprocess_text(file_path):
with open(file_path, 'r') as f:
lines = f.readlines()
text = ' '.join(lines[1:]).replace("\n", ' ').replace(' ',' ').lower().replace('hamilton','').replace('madison', '')
text = ' '.join(text.split())
return text
# Concatenate all the papers known to be written by A/B into a single long text
all_authorA, all_authorB = '',''
for x in os.listdir('./papers/A/'):
all_authorA += preprocess_text('./papers/A/' + x)
for x in os.listdir('./papers/B/'):
all_authorB += preprocess_text('./papers/B/' + x)
# Print lengths of the large texts
print("AuthorA text length: {}".format(len(all_authorA)))
print("AuthorB text length: {}".format(len(all_authorB)))
The output for this should be as follows:
Figure 5.34: Text length count
The next step is to break the long text for each author into many small sequences. As described above, we empirically choose a length for the sequence and use it throughout the model's lifecycle. We get our full dataset by labeling each sequence with its author.
To break the long texts into smaller sequences we use the Tokenizer class from the keras framework. In particular, note that we set it up to tokenize according to characters and not words.
- Choose SEQ_LEN hyper parameter, this might have to be changed if the model doesn't fit well to training data.
- Write a function make_subsequences to turn each document into sequences of length SEQ_LEN and give it a correct label.
- Use Keras Tokenizer with char_level=True
- Fit the tokenizer on all the texts
- Use this tokenizer to convert all texts into sequences using texts_to_sequences()
- Use make_subsequences() to turn these sequences into appropriate shape and length
from keras.preprocessing.text import Tokenizer
# Hyperparameter - sequence length to use for the model
SEQ_LEN = 30
def make_subsequences(long_sequence, label, sequence_length=SEQ_LEN):
len_sequences = len(long_sequence)
X = np.zeros(((len_sequences - sequence_length)+1, sequence_length))
y = np.zeros((X.shape[0], 1))
for i in range(X.shape[0]):
X[i] = long_sequence[i:i+sequence_length]
y[i] = label
return X,y
# We use the Tokenizer class from Keras to convert the long texts into a sequence of characters (not words)
tokenizer = Tokenizer(char_level=True)
# Make sure to fit all characters in texts from both authors
tokenizer.fit_on_texts(all_authorA + all_authorB)
authorA_long_sequence = tokenizer.texts_to_sequences([all_authorA])[0]
authorB_long_sequence = tokenizer.texts_to_sequences([all_authorB])[0]
# Convert the long sequences into sequence and label pairs
X_authorA, y_authorA = make_subsequences(authorA_long_sequence, A)
X_authorB, y_authorB = make_subsequences(authorB_long_sequence, B)
# Print sizes of available data
print("Number of characters: {}".format(len(tokenizer.word_index)))
print('author A sequences: {}'.format(X_authorA.shape))
print('author B sequences: {}'.format(X_authorB.shape))
The output should be as follows:
Figure 5.35: Character count of sequences
- Compare the number of raw characters to the number of labeled sequences for each author. Deep Learning requires many examples of each input. The following code calculates the number of total and unique words in the texts.
# Calculate the number of unique words in the text
word_tokenizer = Tokenizer()
word_tokenizer.fit_on_texts([all_authorA, all_authorB])
print("Total word count: ", len((all_authorA + ' ' + all_authorB).split(' ')))
print("Total number of unique words: ", len(word_tokenizer.word_index))
The output should be as follows:
Figure 5.36: Total word count and unique word count
We now proceed to create our train, validation sets.
- Stack x data together and y data together.
- Use train_test_split to split the dataset into 80% training and 20% validation.
- Reshape the data to make sure that they are sequences of correct length.
# Take equal amounts of sequences from both authors
X = np.vstack((X_authorA, X_authorB))
y = np.vstack((y_authorA, y_authorB))
# Break data into train and test sets
X_train, X_val, y_train, y_val = train_test_split(X,y, train_size=0.8)
# Data is to be fed into RNN - ensure that the actual data is of size [batch size, sequence length]
X_train = X_train.reshape(-1, SEQ_LEN)
X_val = X_val.reshape(-1, SEQ_LEN)
# Print the shapes of the train, validation and test sets
print("X_train shape: {}".format(X_train.shape))
print("y_train shape: {}".format(y_train.shape))
print("X_validate shape: {}".format(X_val.shape))
print("y_validate shape: {}".format(y_val.shape))
The output is as follows:
Figure 5.37: Testing and training datasets
Finally, we construct the model graph and perform the training procedure.
- Create a model using RNN and Dense layers.
- Since its a binary classification problem, the output layer should be Dense with sigmoid activation.
- Compile the model with optimizer, appropriate loss function and metrics.
- Print the summary of the model.
from keras.layers import SimpleRNN, Embedding, Dense
from keras.models import Sequential
from keras.optimizers import SGD, Adadelta, Adam
Embedding_size = 100
RNN_size = 256
model = Sequential()
model.add(Embedding(len(tokenizer.word_index)+1, Embedding_size, input_length=30))
model.add(SimpleRNN(RNN_size, return_sequences=False))
model.add(Dense(1, activation='sigmoid'))
model.compile(optimizer='adam', loss='binary_crossentropy', metrics = ['accuracy'])
model.summary()
The output is as follows:
Figure 5.38: Model summary
- Decide upon the batch size, epochs and train the model using training data and validate with validation data
- Based on the results, go back to model above, change it if needed (use more layers, use regularization, dropout, etc., use different optimizer, or a different learning rate, etc.)
- Change Batch_size, epochs if needed.
Batch_size = 4096
Epochs = 20
model.fit(X_train, y_train, batch_size=Batch_size, epochs=Epochs, validation_data=(X_val, y_val))
The output is as follows:
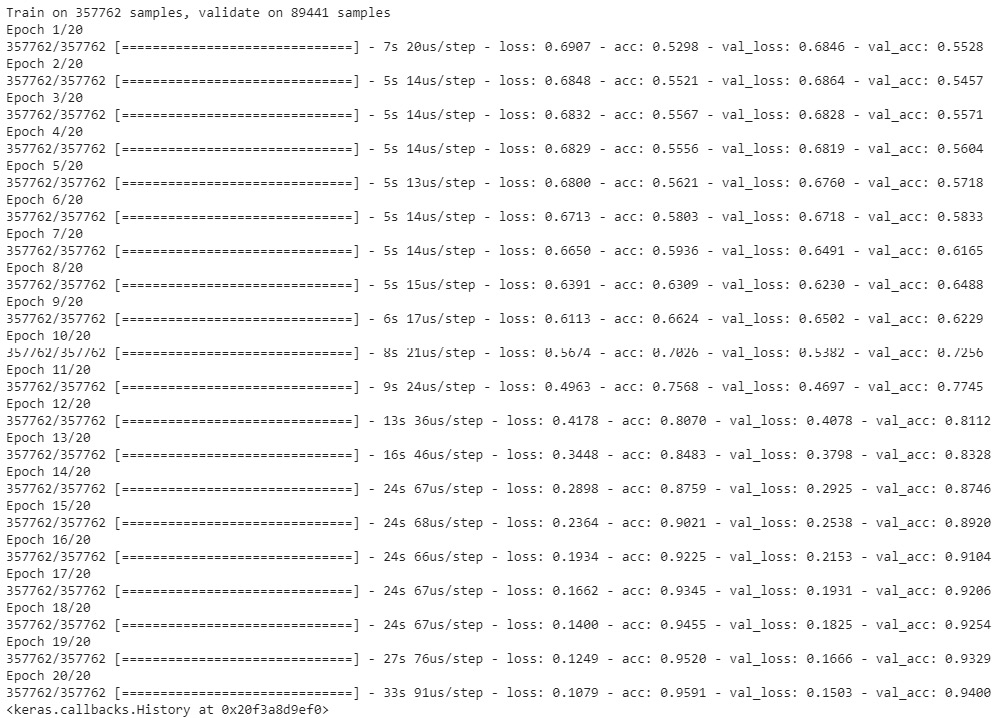
Figure 5.39: Epoch training
Applying the Model to the Unknown Papers
Do this all the papers in the Unknown folder
- Preprocess them same way as training set (lower case, removing white lines, etc.)
- Use tokenizer and make_subsequences function above to turn them into sequences of required size.
- Use the model to predict on these sequences.
- Count the number of sequences assigned to author A and the ones assigned to author B
- Based on the count, pick the author with highest votes/count
for x in os.listdir('./papers/Unknown/'):
unknown = preprocess_text('./papers/Unknown/' + x)
unknown_long_sequences = tokenizer.texts_to_sequences([unknown])[0]
X_sequences, _ = make_subsequences(unknown_long_sequences, UNKNOWN)
X_sequences = X_sequences.reshape((-1,SEQ_LEN))
votes_for_authorA = 0
votes_for_authorB = 0
y = model.predict(X_sequences)
y = y>0.5
votes_for_authorA = np.sum(y==0)
votes_for_authorB = np.sum(y==1)
print("Paper {} is predicted to have been written by {}, {} to {}".format(
x.replace('paper_','').replace('.txt',''),
("Author A" if votes_for_authorA > votes_for_authorB else "Author B"),
max(votes_for_authorA, votes_for_authorB), min(votes_for_authorA, votes_for_authorB)))
The output is as follows:
