



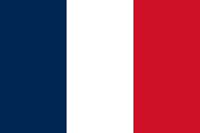


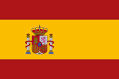



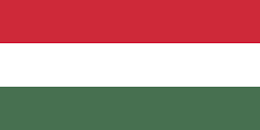

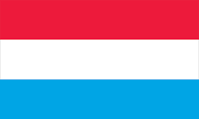





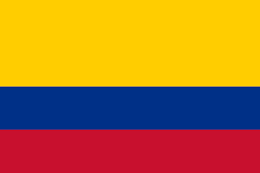
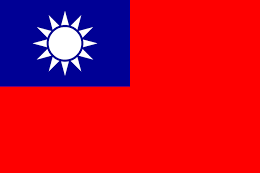
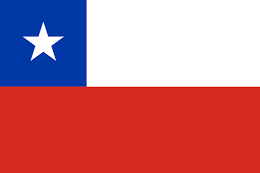



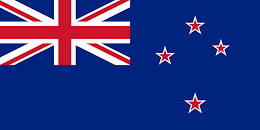
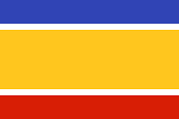
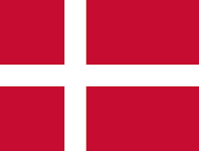
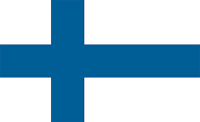







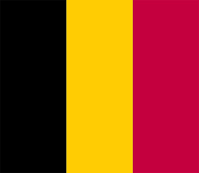

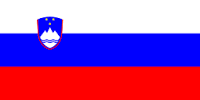





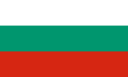


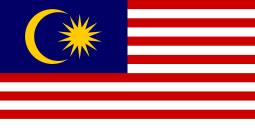



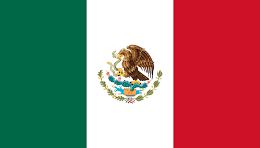

[box type="note" align="" class="" width=""]In this article by Shilpi Saxena and Saurabh Gupta from their book Practical Real-time data Processing and Analytics we shall explore what a near real time architecture looks like and how an NRT app works. [/box]
It's very important to understand the key aspects where the traditional monolithic application systems are falling short to serve the need of the hour:
The answer to the above issues is an architecture that supports streaming and thus provides its end users access to actionable insights in real-time over ever flowing in-streams of real-time fact data.
Before we delve further, it's worthwhile to understand the notation of time:
Looking at this figure, it's very clear to correlate the SLAs with each type of implementation (batch, near real-time, and real-time) and the kinds of use cases each implementation caters to.
For instance, batch implementations have SLAs ranging from a couple of hours to days and such solutions are predominantly deployed for canned/pre-generated reports and trends. The real-time solutions have an SLA of a magnitude of few seconds to hours and cater to situations requiring ad-hoc queries, mid-resolution aggregators, and so on. The real-time application's most mission-critical in terms of SLA and resolutions are where each event accounts for and the results have to return within an order of milliseconds to seconds.
In its essence, NRT Architecture consists of four main components/layers, as depicted in the following figure:
The first step is the collection of data from the source and providing for the same to the "data pipeline", which actually is a logical pipeline that collects the continuous events or streaming data from various producers and provides the same to the consumer stream processing applications. These applications transform, collate, correlate, aggregate, and perform a variety of other operations on this live streaming data and then finally store the results in the low-latency data store. Then, there is a variety of analytical, business intelligence, and visualization tools and dashboards that read this data from the data store and present it to the business user.
This is the beginning of the journey of all data processing, be it batch or real time the foremost and most forthright is the challenge to get the data from its source to the systems for our processing. If I can look at the processing unit as a black box and a data source, and at consumers as publishers and subscribers. It's captured in the following diagram:
The key aspects that come under the criteria for data collection tools in the general context of big data and real-time specifically are as follows:
Apart from this, the data collection tool should be able to cater to data from a variety of sources such as:
The third and a better approach is to go the virtual data lake architecture for data replication.
The stream processing component itself consists of three main sub-components, which are:
The same aspects of the stream processing component are zoomed out and depicted in the diagram as follows:
There are few key attributes that should be catered to by the stream processing component:
The analytical layer is the most creative and interesting of all the components of an NRT application. So far, all we have talked about is backend processing, but this is the layer where we actually present the output/insights to the end user graphically, visually in form of an actionable item.
A few of the challenges these visualization systems should be capable of handling are:
The figure depicts the flow of information from event producers to the collection agents, followed by the brokers and processing engine (transformation, aggregation, and so on) and then the long-term storage. From the storage unit, the visualization tools reap the insights and present them in form of graphs, alerts, charts, Excel sheets, dashboards, or maps, to the business owners who can assimilate the information and take some action based upon it.
The above was an excerpt from the book Practical Real-time data Processing and Analytics.