The bias/variance trade-off
We have discussed the concept of bias and variance briefly in the previous chapters. When we are discussing these two concepts, we are generally speaking of supervised learning algorithms. We are specifically talking about deriving errors from our predictive models due to bias and variance.
Errors due to bias
When speaking of errors due to bias, we are speaking of the difference between the expected prediction of our model and the actual (correct) value, which we are trying to predict. Bias, in effect, measures how far, in general, our model's predictions are from the correct value.
Think about bias as simply being the difference between a predicted value and the actual value. For example, consider that our model, represented as F(x), predicts the value of 29, as follows:
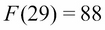
Here, the value of 29 should have been predicted as 79:

If a machine learning model tends to be very accurate in its prediction (regression or classification), then it is considered a low bias...