The basic foundation for ANNs is the same, but various neural network models have been designed during its evolution. The following are a few of the ANN models:
- Adaptive Linear Element (ADALINE), is a simple perceptron which can solve only linear problems. Each neuron takes the weighted linear sum of the inputs and passes it to a bi-polar function, which either produces a +1 or -1 depending on the sum. The function checks the sum of the inputs passed and if the net is >= 0, it is +1, else it is -1.
- Multiple ADALINEs (MADALINE), is a multilayer network of ADALINE units.
- Perceptrons are single layer neural networks (single neuron or unit), where the input is multidimensional (vector) and the output is a function on the weight sum of the inputs.
- Radial basis function network is an ANN where a radial basis function is used as an activation function. The network output is a linear combination of radial basis functions of the inputs and some neuron parameters.
- Feed-forward is the simplest form of neural networks. The data is processed across layers without any loops are cycles. We will study the following feed- forward networks in this book:
- Autoencoder
- Probabilistic
- Time delay
- Covolutional
- Recurrent Neural Networks (RNNs), unlike feed-forward networks, propagate data forward and also backwards from later processing stages to earlier stages. The following are the types of RNNs; we shall study them in our later chapters:
- Hopfield networks
- Boltzmann machine
- Self Organizing Maps (SOMs)
- Bidirectional Associative Memory (BAM)
- Long Short Term Memory (LSTM)
The following images depict (a) Recurrent neural network and (b) Forward neural network:
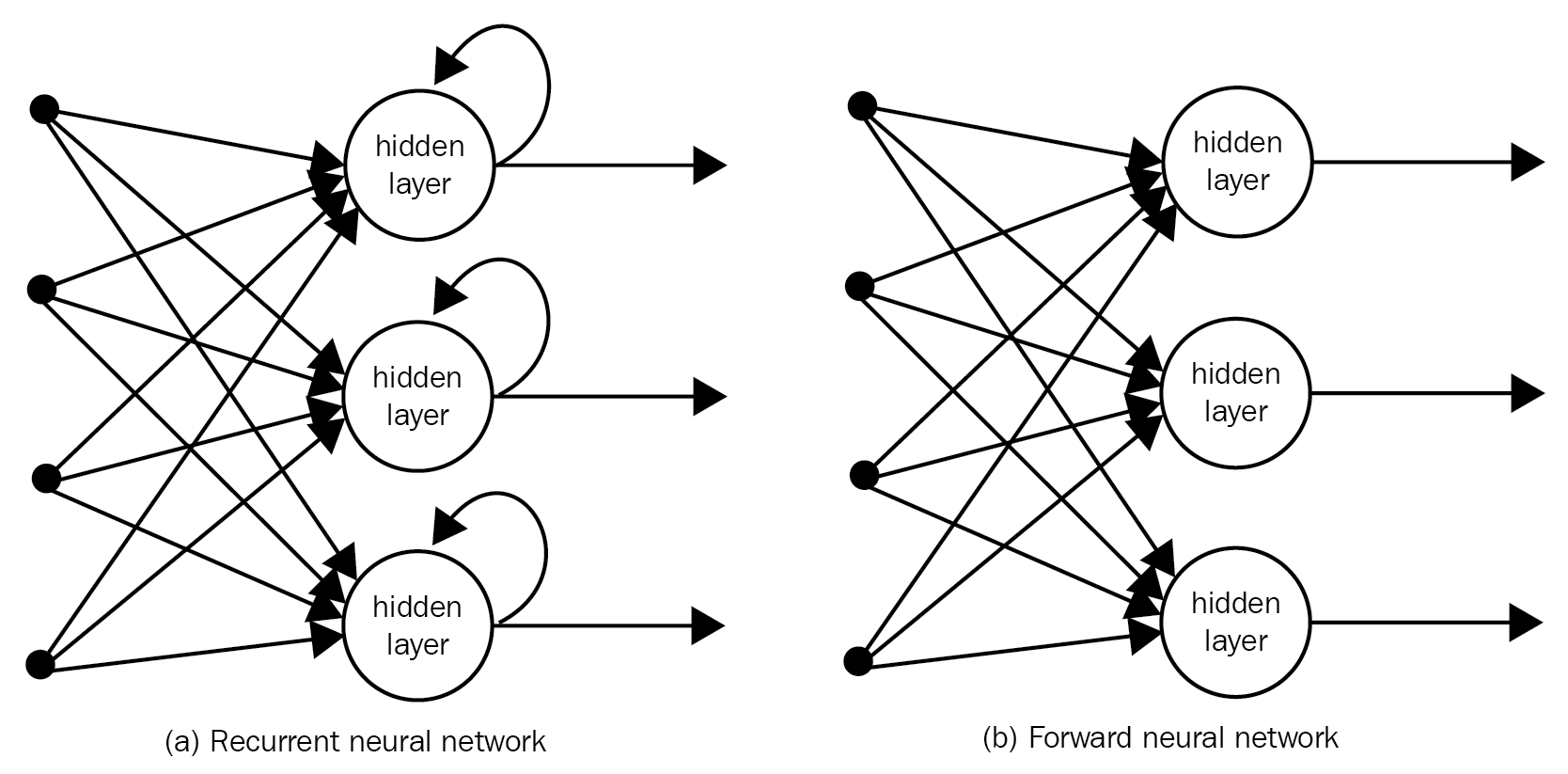