Understanding data ingestion
Data ingestion is the process of copying operational data from data sources to organize it in an analytical database. There are two different techniques for performing this copy: batching data and online data streaming.
It is important to identify latency requirements between the time when the data is generated in the source database and the data availability in the analytical database.
Understanding batch load
When batching the data, the operation is offline. You must define the periodicity for creating the data batch load, collecting data in the data source, and then inserting it into the analytical database.
The periodicity can be hourly, daily, or even monthly, if the requirement of analysis of this data is met. Events that can trigger a batch load can be a new record on a table entity in the database, an action triggered by a user in an application, a manual trigger, and more.
An example of batch processing might be the way we get vote counts in elections. The votes are not counted one by one the moment after the voter has voted, but they are inserted in lots that are processed during election day until the completion of all charges and the definition of the results.
Advantages of batch load
Batch loads can be heavily used in data solutions, but they do not meet all requirements for data solutions. The following are two of the advantages of this ingestion technique:
- It is the most used method by companies that have multiple transactional systems with large volumes of data. This is because due to scheduling loads, it can be made at the most convenient time, such as outside business hours when transactional servers are in lower demand.
- You can monitor the loads to verify where you need to optimize a script or a method independently, so if you need to prioritize one specific load performance, you can manipulate your computing resources to prioritize that load.
Constraints of batch load
To continue the evaluation of the technique, it is important to understand the constraints of adopting batch loads as well:
- There is a delay between the time of data generation on the transactional database and the availability of this data on the analytical database, which sometimes makes it impossible to follow up and immediately make a decision based on the numbers
- The full batch of data must be completed to then begin copying, and if there is any data unavailability, inconsistent data, or network latency between transactional and analytical bases, among other situations, the batch load will fail
Batch loads can be our default data consumption for legacy databases, file repositories, and other types of data sources. But there are business requirements to consume some data in near real time, for monitoring and quick decision-making. And to meet these needs, we have another technique, called data streaming, which loads data online.
Understanding data streaming
In data-streaming-based data ingestion, there is an online connection between the data source and the analytical database, and the pieces of data are processed one by one, in events, right after their generation at this source. For example, for a sales tracking monitoring solution, sales managers need to track sales data in near real time on a dashboard for immediate decision-making. The sales transaction database is linked through a streaming load to the analytical database that receives this data, processes it, and demonstrates it on a monitoring dashboard.
Another example could be a stock exchange and its real-time stock tracking panels. These dashboards receive processed information from purchase and sale transaction data for stock papers in a data stream. See the following figure with the data flow in this scenario:
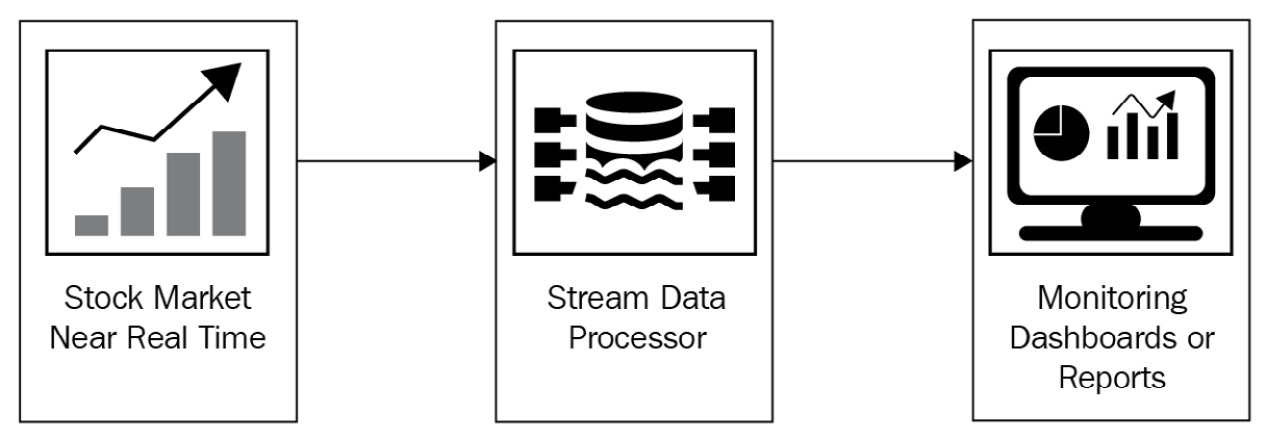
Figure 1.12 – Stock market example diagram
The load on data streaming is not always done online; it can also be done at intervals that load a portion of data. Data streaming is a continuous window of data ingestion between the source and the destination, while in the batch load, each batch opens and closes the connection to the process.
Let us now evaluate the advantages and disadvantages of the data streaming technique.
Advantages of data streaming
The advantages are listed as follows:
- The delay between data creation and analytical processing can be minimal
- The latency between the source and the target in the order of seconds or milliseconds
- Analytical solutions can demonstrate both past data and performance trends, which assists in immediate decision-making while events are happening
Constraints of streaming data load
The disadvantages are listed as follows:
- Most transactional database technologies do not have a native streaming data export technology, so you need to implement this technique through manual control of what has already been ingested and what has not yet been ingested. This generates great complexity.
- The size of each event is usually small to avoid having a very robust infrastructure to maintain this event's queue during the streaming. This makes it impossible to ingest large files, videos, audio, and photos, among others. These loads are often best implemented in batch loads.
In summary, we typically use batch data loads for the most of the structuring operations of the analytical base, the ingestion of the largest volumes of data, and unstructured data.
To understand in practice how these concepts are applied, let’s now evaluate a case study of a complete data solution.