Shared memory versus distributed memory
Conceptually, parallel computing and distributed computing look very similar—after all, they both are about breaking up some computation into several smaller parts and running those on processors. Some of you might ponder upon the fact that in one case the processors in use are part of the same computer, whereas in the other case they are physically on different computers; is this just a trivial technicality?
The answer is maybe. As we saw, some applications are fundamentally distributed. Others simply need more performance than they can get on a single box. For some of these applications, the answer is maybe yes—it does not really matter where the processing power comes from. However, in all cases, the physical location of the hardware resources in use has significant implications.
Possibly, the most obvious difference between parallel and distributed computing is in the underlying memory architecture and access patterns. In the case of a parallel application, all concurrent tasks can—in principle—access the same memory space. Here, we have to say in principle because as we have already saw, parallelism does not necessary imply the use of threads (which can indeed access the same memory space).
In the following figure, we see a typical shared-memory architecture where four processors (the four CPU boxes in the following diagram) can all access the same memory address space (that is, the Memory box). If our application were to use threads, then those would be able to access exactly the same memory locations, if needed:
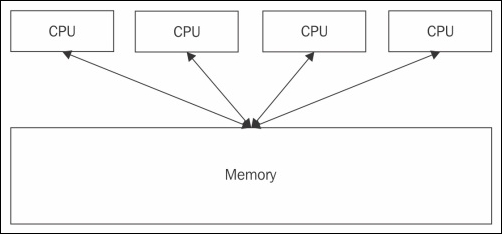
In the case of a distributed application, however, the various concurrent tasks cannot normally access the same memory space. The reason being that some tasks run on one computer and others on another, physically separated, computer.
Since these computers are able to talk to each other over the network, one could imagine writing a software layer (a middleware) that could present our application with a unified logical (as opposed to physical) memory space. These types of middlewares do exist and implement what is known as distributed shared-memory architecture. We will not examine these systems in this book.
In the following figure, we have the same four CPUs as before, that are organized now in a shared-memory architecture. Each CPU has access to its own private memory and cannot see any other CPU memory space. The four computers (indicated by the boxes surrounding their CPU and memory) communicate through the network (the black line connecting them). Each data transfer between boxes happens over the network:
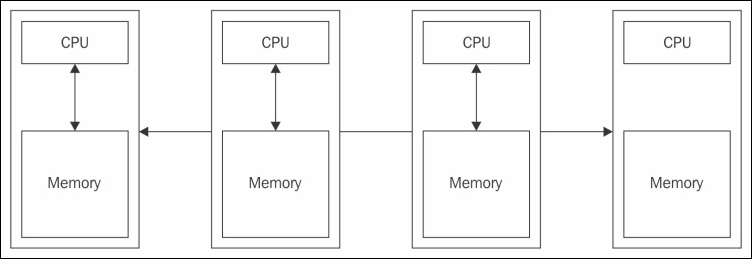
In reality, the computers one is likely to use nowadays are a hybrid of the two extremes that we just described in the previous section. Computers communicate over the network just like in a pure distributed-memory architecture. However, each computer has more than one processor and/or processor core, implementing a shared-memory architecture. The following figure schematically illustrates such a hybrid architecture that is shared within each individual computer (indicated by a box enclosing two CPUs and its own memory) and distributed across boxes (linked together via the network):
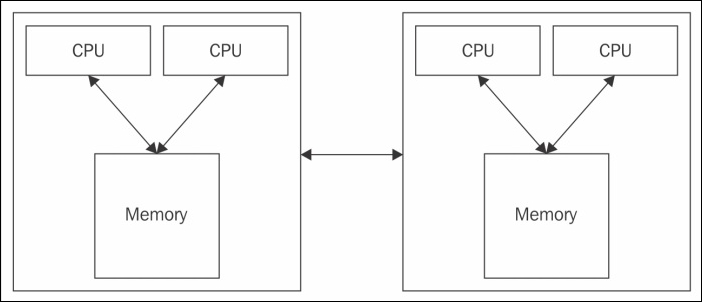
Each of these architectures has its own pros and cons. In the case of shared-memory systems, sharing data across various concurrent threads of a single executable is quite fast and tremendously faster than using the network. In addition, having a single, uniform memory address space makes writing the code arguably simpler.
At the same time, special care has to be exercised in the design of the program to avoid multiple threads from stepping on each other's toes and changing variables behind each other's backs.
A distributed memory system tends to be very scalable and cheap to assemble; need more power? Simply add another box. Another advantage is that processors can access their own memory in isolation without worrying about race conditions (while this is technically true, oftentimes, different tasks running in parallel need to read and write data in a common repository, for example, a database or a shared filesystem. In those cases, we still have to deal with race conditions). Disadvantages of this system include the fact that programmers need to implement their own strategy to move data around and they need to worry about issues of data locality. Also, not all algorithms easily map to these architectures.