



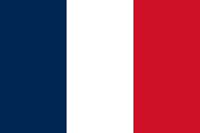


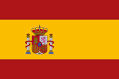



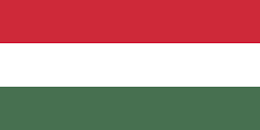

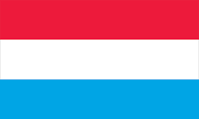





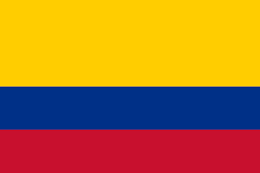
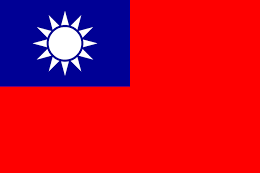
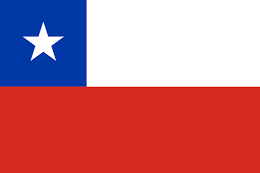



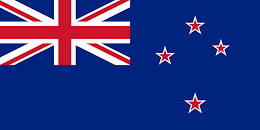
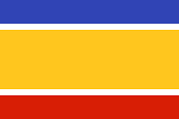
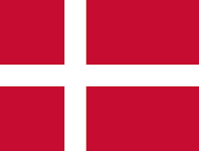
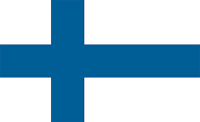







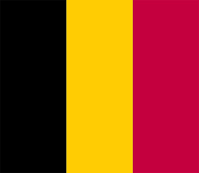

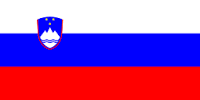





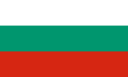


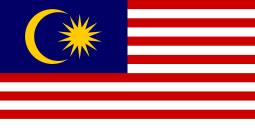



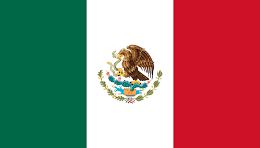

Your work has real consequences. And your projects will often be larger than what you know or can do. As such, the first step toward creating impact with intention is to define the project scope, purpose, outcomes and assets clearly. The next most important factor is choosing the project team.
To become a successful data scientist you must learn how to collaborate. Not only does it make projects fun and efficient, but it also brings in diverse points of view and expertise from other disciplines. This is a great advantage for machine learning projects that attempt to solve complex real-world problems.
You could benefit from working with other technical professionals like web developers, software programmers, data analysts, data administrators, game developers etc. Collaborating with such people will enhance your own domain knowledge and skills and also let you see your work from a broader technical perspective.
Apart from the people involved in the core data and software domain, there are others who also have a primary stake in your project’s success. These include UX designers, people with humanities background if you are building a product intended to participate in society (which most products often are), business development folks, who actually sell your product and bring revenue, marketing people, who are responsible for bringing your product to a much wider audience to name a few. Working with people of diverse skill sets will help market your product right and make it useful and interpretable to the target audience.
In addition to working with a melange of people with diverse skill sets and educational background it is also important to work with people who think differently from you, and who have experiences that are different from yours to get a more holistic idea of the problems your project is trying to tackle and to arrive at a richer and unique set of solutions to solve those problems.
As an aspiring data scientist, you should always keep in mind the ethical aspects surrounding privacy, data sharing, and algorithmic decision-making. Here are some ways to develop a mind inclined to designing ethically-sound data science projects and models.
Finally, for any data science project, always reassess your hypotheses before, during, and after the actual implementation. Always ask yourself these questions after each of the above steps and compare them with the previous answers.
These pointers will help you evaluate your project goals from a customer and business point of view. Additionally, it will also help you in building efficient models which can benefit the society and your organization at large.
With this, we come to the end of our new year resolutions for an aspiring data scientist. However, the beauty of the ideas behind these resolutions is that they are easily transferable to anyone in any job. All you gotta do is get your foundations right, stay relevant, and be mindful of your impact. We hope this gives a great kick start to your career in 2018.
“Motivation is what gets you started. Habit is what keeps you going.” ― Jim Ryun
Happy New Year! May the odds and the God(s) be in your favor this year to help you build your resolutions into your daily routines and habits!