ML lifecycle
One of the early ML projects that I worked on was a fascinating yet daunting sports predictive analytics problem for a major league brand. I was given a list of predictive analytics outcomes to think about to see if there were ML solutions for the problems. I was a casual viewer of the sport; I didn’t know anything about the analytics to be generated, nor the rules of the games in the detail that was needed. I was provided with some sample data but had no idea what to do with it.
The first thing I started to work on was an immersion in the sport itself. I delved into the intricacies of the game, studying the different player positions and events that make up each game and play. Only after being armed with the newfound domain knowledge did the data start to make sense. Together with the stakeholder, we evaluated the impact of the different analytics outcomes and assessed the modeling feasibility based on the data we had. With a clear understanding of the data, we came up with a couple of top ML analytics with the most business impact to focus on. We also decided how they would be integrated into the existing business workflow, and how they would be measured on their impacts.
Subsequently, I delved deeper into the data to ascertain what information was available and what was lacking. The raw dataset had a lot of irrelevant data points that needed to be removed while the relevant data points needed to be transformed to provide the strongest signals for model training. I processed and prepared the dataset based on a few of the ML algorithms I had considered and conducted experiments to determine the best approach. I lacked a tool to track the different experiment results, so I had to document what I had done manually. After some initial rounds of experimentation, it became evident that the existing data was not sufficient to train a high-performance model. Hence, I decided to build a custom deep learning model to incorporate data of different modalities as the data points had temporal dependencies and required additional spatial information for the modeling. The data owner was able to provide the additional datasets I required, and after more experiments with custom algorithms and significant data preparations and feature engineering, I eventually trained a model that met the business objectives.
After completing the model, another hard challenge began – deploying and operationalizing the model in production and integrating it into the existing business workflow and system architecture. We engaged in many architecture and engineering discussions and eventually built out a deployment architecture for the model.
As you can see from my personal experience, the journey from business idea to ML production deployment involved many steps. A typical lifecycle of an ML project follows a formal structure, which includes several essential stages like business understanding, data acquisition and understanding, data preparation, model building, model evaluation, and model deployment. Since a big component of the lifecycle is experimentation with different datasets, features, and algorithms, the whole process is highly iterative. Furthermore, it is essential to note that there is no guarantee of a successful outcome. Factors such as the availability and quality of data, feature engineering techniques (the process of using domain knowledge to extract useful features from raw data), and the capability of the learning algorithms, among others, can all affect the final results.
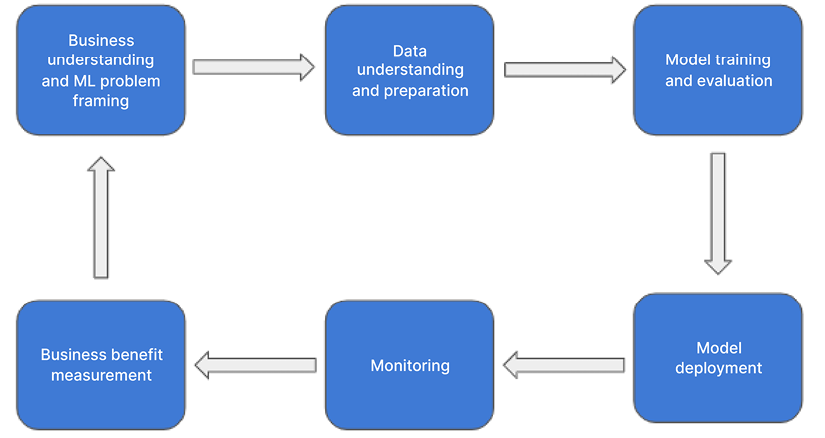
Figure 1.2: ML lifecycle
The preceding figure illustrates the key steps in ML projects, and in the subsequent sections, we will delve into each of these steps in greater detail.
Business problem understanding and ML problem framing
The first stage in the lifecycle is business understanding. This stage involves the understanding of the business goals and defining business metrics that can measure the project’s success. For example, the following are some examples of business goals:
- Cost reduction for operational processes, such as document processing.
- Mitigation of business or operational risks, such as fraud and compliance.
- Product or service revenue improvements, such as better target marketing, new insight generation for better decision making, and increased customer satisfaction.
To measure the success, you may use specific business metrics such as the number of hours reduced in a business process, an increased number of true positive frauds detected, a conversion rate improvement from target marketing, or the number of churn rate reductions. This is an essential step to get right to ensure there is sufficient justification for an ML project and that the outcome of the project can be successfully measured.
After you have defined the business goals and business metrics, you need to evaluate if there is an ML solution for the business problem. While ML has a wide scope of applications, it is not always an optimal solution for every business problem.
Data understanding and data preparation
The saying that “data is the new oil” holds particularly true for ML. Without the required data, you cannot move forward with an ML project. That’s why the next step in the ML lifecycle is data acquisition, understanding, and preparation.
Based on the business problems and ML approach, you will need to gather and comprehend the available data to determine if you have the right data and data volume to solve the ML problem. For example, suppose the business problem to address is credit card fraud detection. In that case, you will need datasets such as historical credit card transaction data, customer demographics, account data, device usage data, and networking access data. Detailed data analysis is then necessary to determine if the dataset features and quality are sufficient for the modeling tasks. You also need to decide if the data needs labeling, such as fraud
or not-fraud
. During this step, depending on the data quality, a significant amount of data wrangling might be performed to prepare and clean the data and to generate the dataset for model training and model evaluation, depending on the data quality.
Model training and evaluation
Using the training and validation datasets established, a data scientist must run a number of experiments using different ML algorithms and dataset features for feature selection and model development. This is a highly iterative process and could require numerous runs of data processing and model development to find the right algorithm and dataset combination for optimal model performance. In addition to model performance, factors such as data bias and model explainability may need to be considered to comply with internal or regulatory requirements.
Prior to deployment into production, the model quality must be validated using the relevant technical metrics, such as the accuracy score. This is usually accomplished using a holdout dataset, also known as a test dataset, to gauge how the model performs on unseen data. It is crucial to understand which metrics are appropriate for model validation, as they vary depending on the ML problems and the dataset used. For example, model accuracy would be a suitable validation metric for a document classification use case if the number of document types is relatively balanced. However, model accuracy would not be a good metric to evaluate the model performance for a fraud detection use case – this is because the number of frauds is small and even if the model predicts not-fraud
all the time, the model accuracy could still be very high.
Model deployment
After the model is fully trained and validated to meet the expected performance metric, it can be deployed into production and the business workflow. There are two main deployment concepts here. The first involves the deployment of the model itself to be used by a client application to generate predictions. The second concept is to integrate this prediction workflow into a business workflow application. For example, deploying the credit fraud model would either host the model behind an API for real-time prediction or as a package that can be loaded dynamically to support batch predictions. Moreover, this prediction workflow also needs to be integrated into business workflow applications for fraud detection, which might include the fraud detection of real-time transactions, decision automation based on prediction output, and fraud detection analytics for detailed fraud analytics.
Model monitoring
The ML lifecycle does not end with model deployment. Unlike software, whose behavior is highly deterministic since developers explicitly code its logic, an ML model could behave differently in production from its behavior in model training and validation. This could be caused by changes in the production data characteristics, data distribution, or the potential manipulation of request data. Therefore, model monitoring is an important post-deployment step for detecting model performance degradation (a.k.a model drift) or dataset distribution change in the production environment (a.k.a data drift).
Business metric tracking
The actual business impact should be tracked and measured as an ongoing process to ensure the model delivers the expected business benefits. This may involve comparing the business metrics before and after the model deployment, or A/B testing where a business metric is compared between workflows with or without the ML model. If the model does not deliver the expected benefits, it should be re-evaluated for improvement opportunities. This could also mean framing the business problem as a different ML problem. For example, if churn prediction does not help improve customer satisfaction, then consider a personalized product/service offering to solve the problem.