Machine learning reference architecture
The following architecture depicts a bank loan approval workflow based on customer data built on the AWS cloud platform.
Here, customer data ingested into the cloud and ML framework decides on the customer loan application.
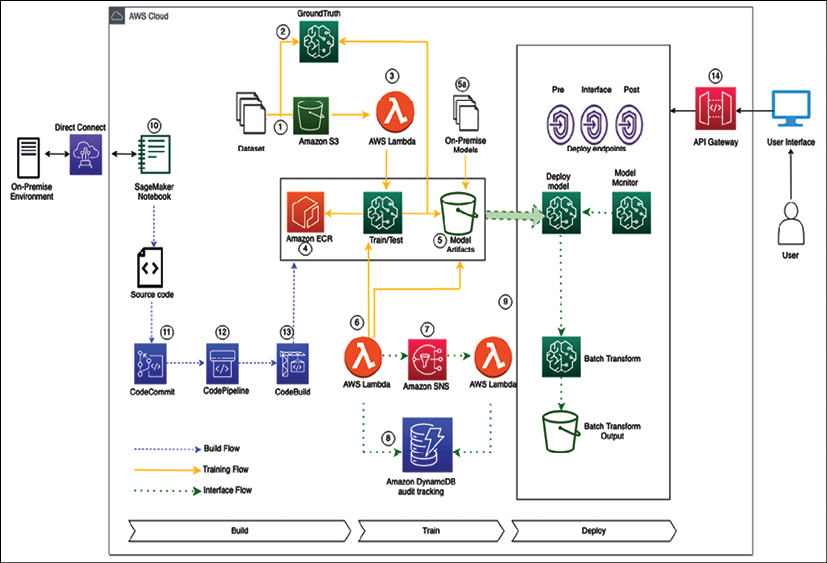
Figure 14.3: ML architecture in the AWS cloud
In designing the above architecture, some fundamental design principles to consider as a guide are:
- Training workflow:
- Datasets enter the process flow using S3. This data may be raw input data or preprocessed from on-premises datasets.
- Ground Truth is used to build a high-quality training labeled dataset for ML models. If required, the data can use the Ground Truth service to label the data.
- AWS Lambda can be used for data integration, preparation, and cleaning before datasets are passed to SageMaker.
- Data scientists will interface with SageMaker to train and test their models. The Docker images used by SageMaker...