The desire to model sequential data more effectively, without the limitations of the gradient problem, led researchers to create the LSTM variant of the previous RNN model architecture. LSTM achieves better performance because it incorporates gates to control the process of memory in the cell. The following diagram shows an LSTM cell:
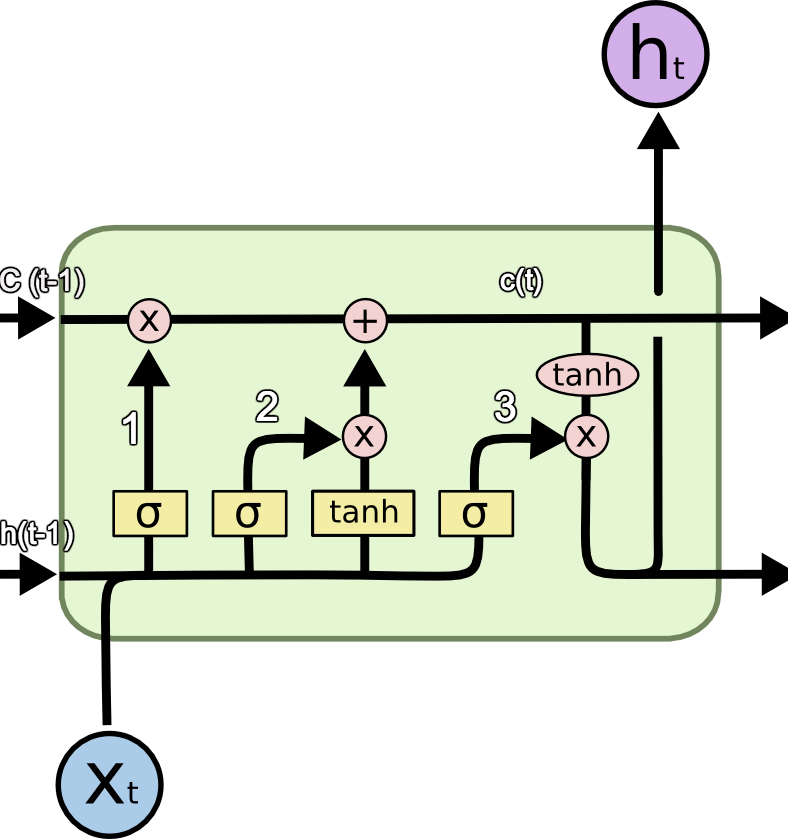
An LSTM unit (source: http://colah.github.io/posts/2015-08-Understanding-LSTMs)
LSTM consist of three primary elements, labeled as 1, 2, and 3 in the preceding diagram:
- The forget gate f(t): This gate provides the ability, in the LSTM cell architecture, to forget information that is not needed. The sigmoid activation accepts the inputs X(t) and h(t-1), and effectively decides to remove pieces of old output information by passing a 0. The output of this gate is f(t)*c(t-1).
- Information from the new input, X(t), that is determined...