When the data is too large to be processed, it is transformed into a reduced representation set of features. The process of transforming the input data into a set of features is called feature extraction. Indeed, feature extraction starts from an initial set of measured data and builds derivative values that can retain the information contained in the original dataset, but emptied of redundant data, as shown in the following figure:
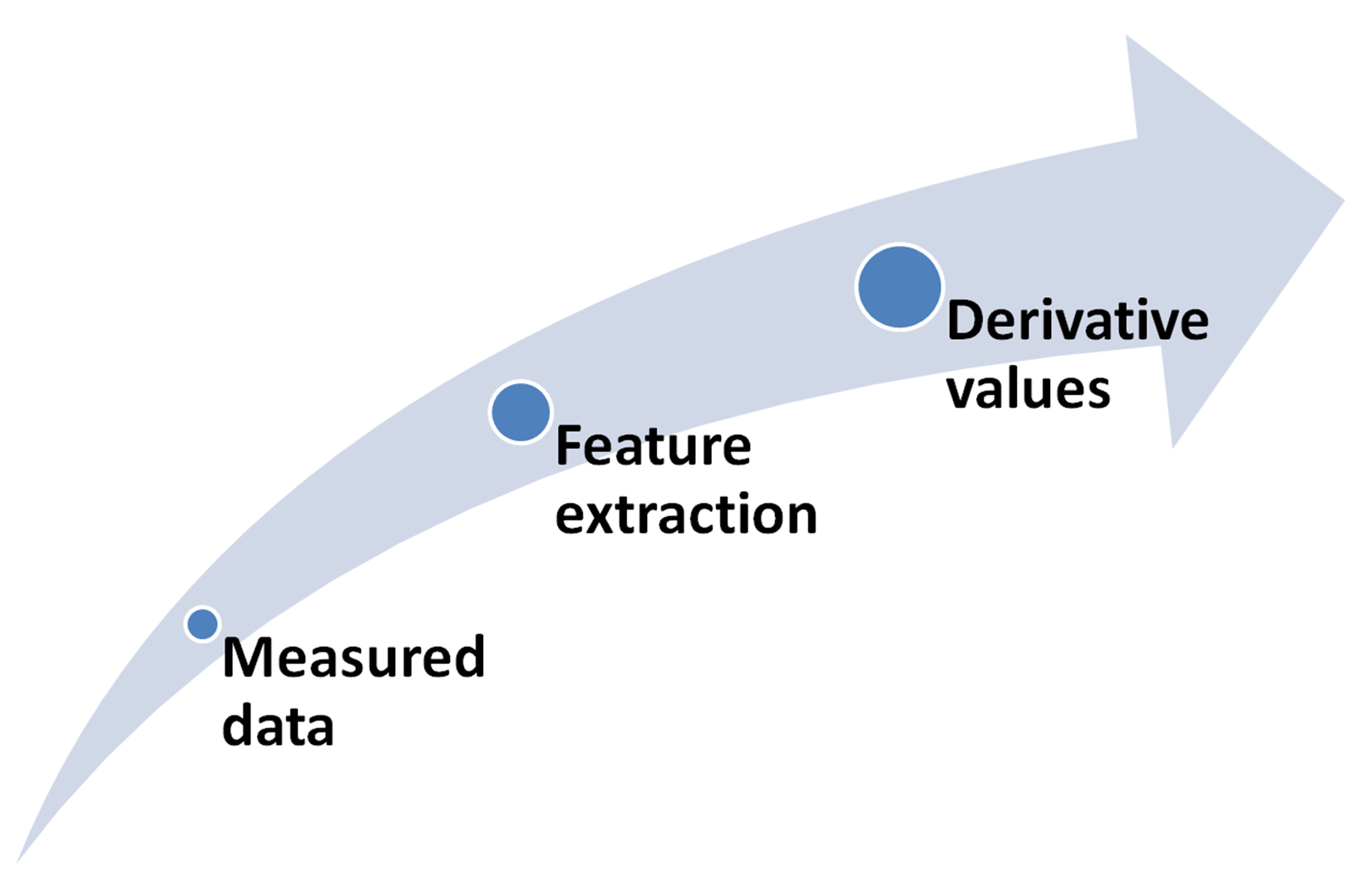
In this way, the subsequent learning and generalization phases will be facilitated and, in some cases, will lead to better interpretations. It is a process of deriving new features from the original features in order to reduce the cost of feature measurement, increase classifier efficiency, and allow higher classification accuracy. If the features extracted are carefully chosen, it is expected...