As you may have inferred from the title of this section, the big drawback of the approach introduced in the previous section is that the process requires iterative optimization, as summarized in the following figure:
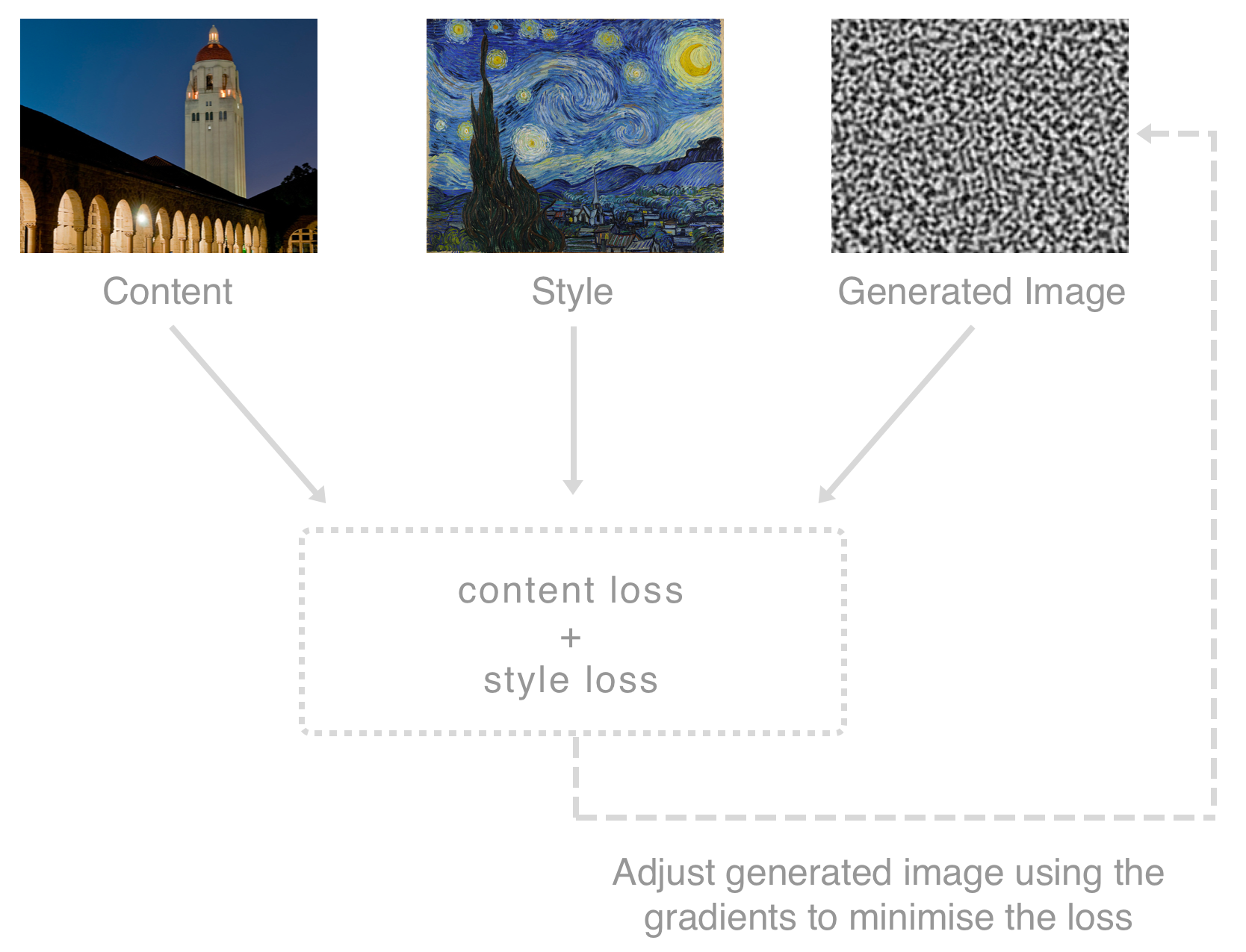
This optimization is akin to training, in terms of performing many iterations to minimize the loss. Therefore, it typically takes a considerable amount of time, even when using a modest computer. As implied at the start of this book, we ideally want to restrict ourselves to performing inference on the edge as it requires significantly less compute power and can be run in near-real time, allowing us to adopt it for interactive applications. Luckily for us, in their paper Perceptual Losses for Real-Time Style Transfer and Super-Resolution, J. Johnson, A. Alahi, and L. Fei-Fei describe a technique that decouples training (optimization...