In this chapter, we have briefly discussed some basic deep learning concepts, and the reader should now understand what a computational graph is and how it can be modeled using TensorFlow. A deep architecture, in fact, can be seen as a sequence of layers connected to each other. They can have different characteristics and purposes, but the overall graph is always a directed structure that associates input values with a final output layer. Therefore, it's possible to derive a global loss function that will be optimized by a training algorithm. We also saw how TensorFlow computes the gradients of an output tensor with respect to any previous connected layer and therefore how it's possible to implement the standard back-propagation strategy seamlessly to deep architectures. We did not discuss actual deep learning problems and methods because they require much more...




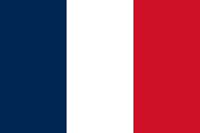


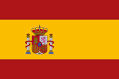



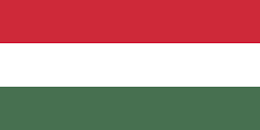

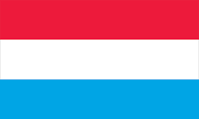





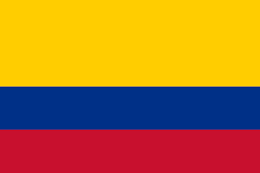
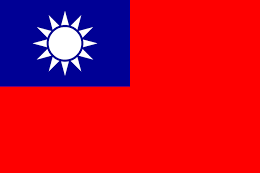
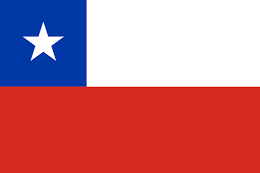



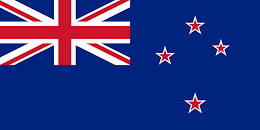
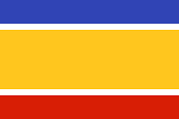
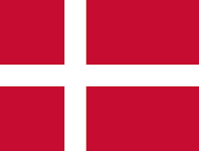
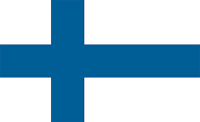







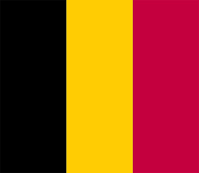

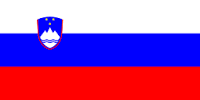





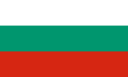


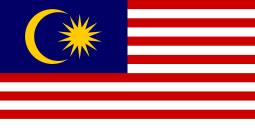



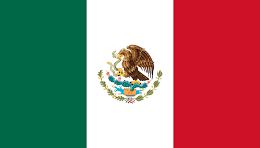
