In this chapter, we briefly presented the concept of recommendation systems and how collaborative filtering works. We then presented how neural networks can be utilized in order to avoid explicitly defining rules that dictate how unrated items would be rated by a user, using embedding layers and dot products. Following that, we showed how the performance of these models can be improved if we allow the networks to learn how to combine the embedding layers themselves. This gives the models considerably higher degrees of freedom without drastically increasing the number of parameters, leading to considerable increases in performance. Finally, we showed how the same architecture—with variable numbers of embedding features—can be utilized in order to create base learners for a stacking ensemble. In order to combine the base learners, we utilized a Bayesian ridge...




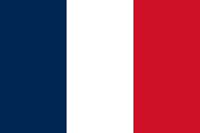


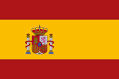



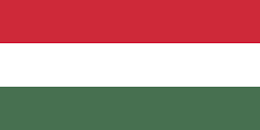

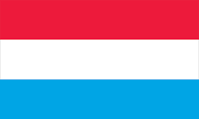





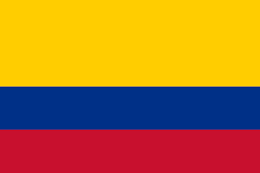
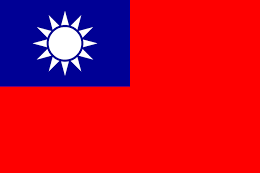
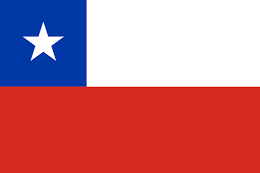



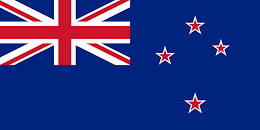
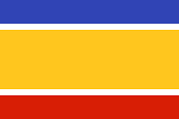
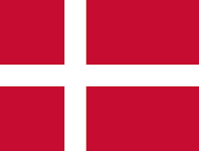
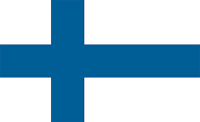







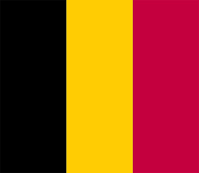

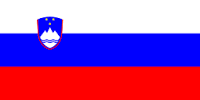





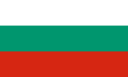


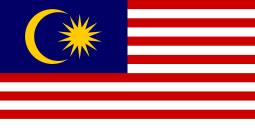



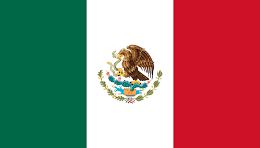
