Conclusion
As mentioned earlier, even if accuracy is a common measure from the classification report that most people will look at, the way to treat this kind of imbalanced data scenario is to compare the models using a balanced accuracy score, or AUC score, which in this case are the same, since it is a binary classification challenge.
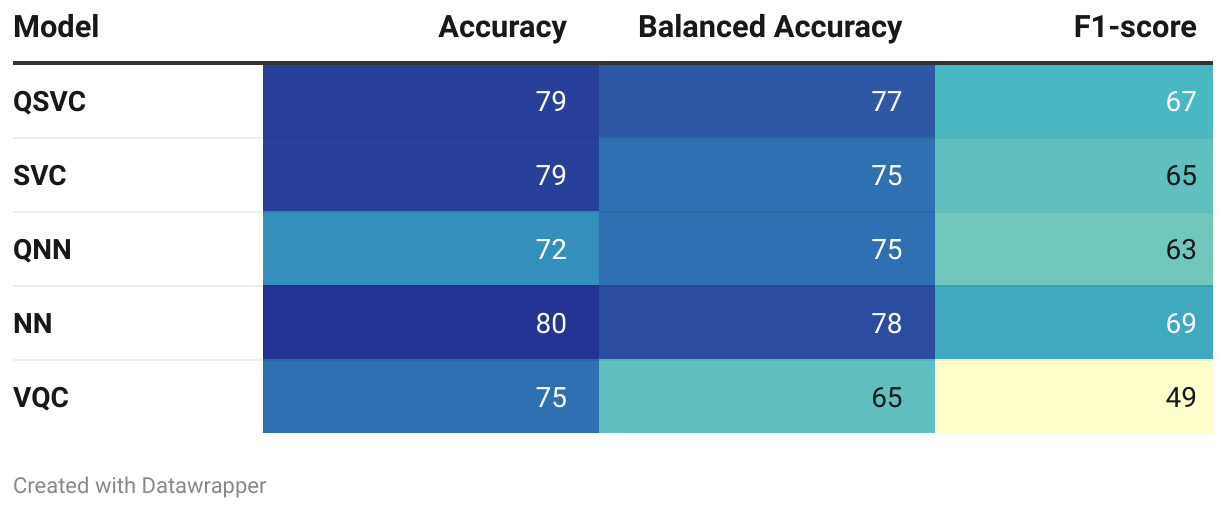
Figure 6.8 – A comparison of classification results between classical and hybrid quantum-classical methods
At first glance, the results do not appear to be conclusive about the benefits of using hybrid quantum-classical for classification problems that the finance sector may face. However, the purpose of the exercise in this chapter is to get people to think about their own business challenges and do more research, since we can see that quantum machine learning could be at least equal or slightly better than classical ML methods (e.g., QSVC versus SVC). When any incremental benefit is achieved in terms of...