Data pipeline blueprint
At a high level, data pipelines can be described using the following generic blueprint:
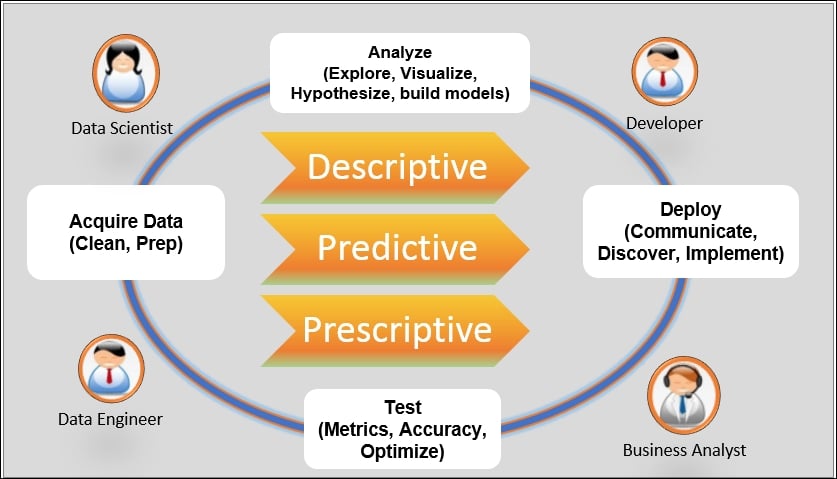
Data pipeline workflow
The main objective of a data pipeline is to operationalize (that is, provide direct business value) the data science analytics outcome in a scalable, repeatable process, and with a high degree of automation. Examples of analytics could be a recommendation engine to entice consumers to buy more products, for example, the Amazon recommended list, or a dashboard showing Key Performance Indicators (KPIs) that can help a CEO make future decisions for the company.
There are multiple persons involved in the building of a data pipeline:
- Data engineers: They are responsible for designing and operating information systems. In other words, data engineers are responsible for interfacing with data sources to acquire the data in its raw form and then massage it (some call this data wrangling) until it is ready to be analyzed. In the Amazon recommender system example, they would implement a streaming processing pipeline that captures and aggregates specific consumer transaction events from the e-commerce system of records and stores them into a data warehouse.
- Data scientists: They analyze the data and build the analytics that extract insight. In our Amazon recommender system example, they could use a Jupyter Notebook that connects to the data warehouse to load the dataset and build a recommendation engine using, for example, collaborative filtering algorithm (https://en.wikipedia.org/wiki/Collaborative_filtering).
- Developers: They are responsible for operationalizing the analytics into an application targeted at line of business users (business analysts, C-Suite, end users, and so on). Again, in the Amazon recommender system, the developer will present the list of recommended products after the user has completed a purchase or via a periodic email.
- Line of business users: This encompasses all users that consume the output of data science analytics, for example, business analysts analyzing dashboards to monitor the health of a business or the end user using an application that provides a recommendation as to what to buy next.
Note
In real-life, it is not uncommon that the same person plays more than one of the roles described here; this may mean that one person has multiple, different needs when interacting with a data pipeline.
As the preceding diagram suggests, building a data science pipeline is iterative in nature and adheres to a well-defined process:
- Acquire Data: This step includes acquiring the data in its raw form from a variety of sources: structured (RDBMS, system of records, and so on) or unstructured (web pages, reports, and so on):
- Data cleansing: Check for integrity, fill missing data, fix incorrect data, and data munging
- Data prep: Enrich, detect/remove outliers, and apply business rules
- Analyze: This step combines descriptive (understand the data) and prescriptive (build models) activities:
- Explore: Find statistical properties, for example, central tendency, standard deviation, distribution, and variable identification, such as univariate and bivariate analysis, the correlation between variables, and so on.
- Visualization: This step is extremely important to properly analyze the data and form hypotheses. Visualization tools should provide a reasonable level of interactivity to facilitate understanding of the data.
- Build model: Apply inferential statistics to form hypotheses, such as selecting features for the models. This step usually requires expert domain knowledge and is subject to a lot of interpretation.
- Deploy: Operationalize the output of the analysis phase:
- Communicate: Generate reports and dashboards that communicate the analytic output clearly for consumption by the line of business user (C-Suite, business analyst, and so on)
- Discover: Set a business outcome objective that focuses on discovering new insights and business opportunities that can lead to a new source of revenue
- Implement: Create applications for end-users
- Test: This activity should really be included in every step, but here we're talking about creating a feedback loop from field usage:
- Create metrics that measure the accuracy of the models
- Optimize the models, for example, get more data, find new features, and so on