Setting up a sweep job for Bayesian sampling
Earlier in the chapter, we cloned our sample notebook to leverage this material. The notebook for this chapter, '
Chapter 4
- Hyperparameter Tuning'
, provides a review on creating a job command to create a logistic regression model by leveraging an sklearn
pipeline and mlflow
capabilities.
The code is then updated and placed into a new directory – the hyperparametertune
folder, which leverages Python’s argparse
module and enables you to pass parameters into scripts. To run the script that has been generated by this notebook, we will create a job command and update the job command to include the hyperparameters as shown in the code snippet here. Conveniently, the job command is the same as was found for the random sampling displayed in Figure 4.16.
The only difference here is that the sampling algorithm is defined as bayesian
, as shown in the figure:
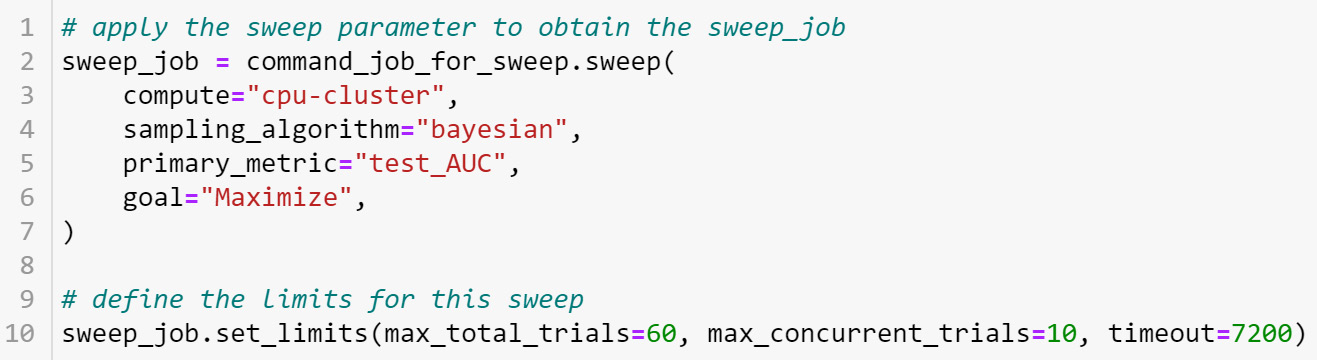
Figure 4.22 – Calling a sweep...