Technical requirements
In this chapter, we will use the pandas, NumPy, and Matplotlib Python libraries, as well as scikit-learn and Feature-engine. For guidelines on how to obtain these libraries, visit the Technical requirements section of Chapter 1, Imputing Missing Data.
We will also use the open-source Category Encoders
Python library, which can be installed using pip
:
pip install category_encoders
To learn more about Category Encoders
, visit the following link: https://contrib.scikit-learn.org/category_encoders/.
We will also use the Credit Approval dataset, which is available in the UCI Machine Learning Repository at https://archive.ics.uci.edu/ml/datasets/credit+approval.
To prepare the dataset, follow these steps:
- Visit http://archive.ics.uci.edu/ml/machine-learning-databases/credit-screening/ and click on
crx.data
to download the data:
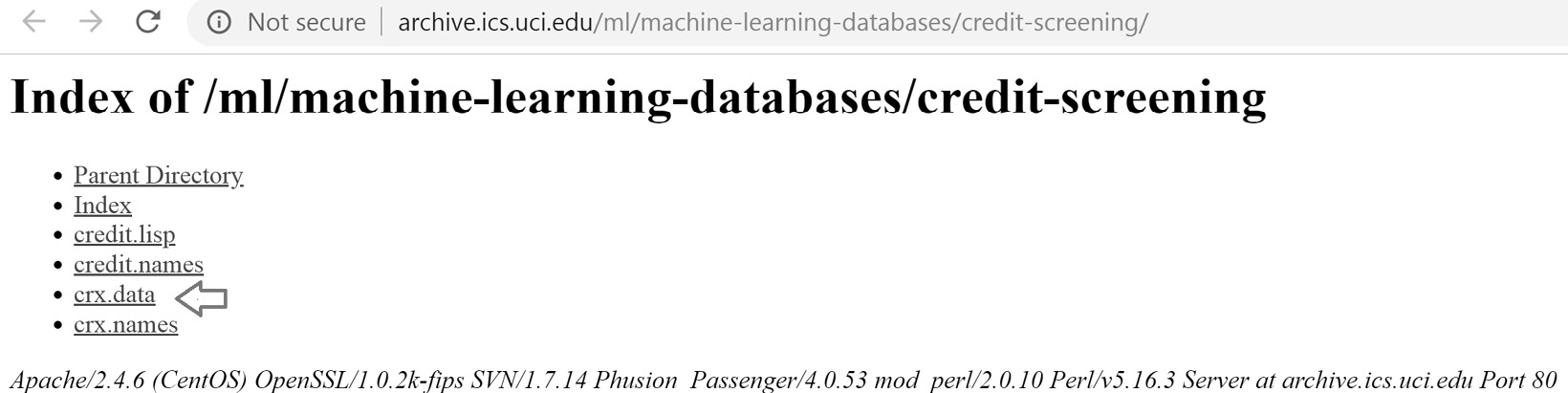
Figure 2.1 – The index directory for the Credit Approval dataset
- Save
crx.data
to the folder where you will run the following commands.
After downloading the data, open up a Jupyter Notebook and run the following commands.
- Import the required libraries:
import random import numpy as np import pandas as pd
- Load the data:
data = pd.read_csv("crx.data", header=None)
- Create a list containing the variable names:
varnames = [f"A{s}" for s in range(1, 17)]
- Add the variable names to the DataFrame:
data.columns = varnames
- Replace the question marks in the dataset with NumPy NaN values:
data = data.replace("?", np.nan)
- Cast some numerical variables as
float
data types:data["A2"] = data["A2"].astype("float") data["A14"] = data["A14"].astype("float")
- Encode the target variable as binary:
data["A16"] = data["A16"].map({"+": 1, "-": 0})
- Rename the target variable:
data.rename(columns={"A16": "target"}, inplace=True)
- Make lists that contain categorical and numerical variables:
cat_cols = [ c for c in data.columns if data[c].dtypes=="O"] num_cols = [ c for c in data.columns if data[c].dtypes!= "O"]
- Fill in the missing data:
data[num_cols] = data[num_cols].fillna(0) data[cat_cols] = data[cat_cols].fillna("Missing")
- Save the prepared data:
data.to_csv("credit_approval_uci.csv", index=False)
You can find a Jupyter Notebook that contains these commands in this book’s GitHub repository at https://github.com/PacktPublishing/Python-Feature-Engineering-Cookbook-Second-Edition/blob/main/ch02-categorical-encoding/donwload-prepare-store-credit-approval-dataset.ipynb.
Note
Some libraries require that you have already imputed missing data, for which you can use any of the recipes from Chapter 1, Imputing Missing Data.