The intent of this project is to develop an ML workflow or more accurately a pipeline. The goal is to solve the classification problem on the most famous dataset in data science history.Â
If we saw a flower out in the wild that we know belongs to one of three Iris species, we have a classification problem on our hands. If we made measurements (X) on the unknown flower, the task is to learn to recognize the species to which the flower (and its plant) belongs.
Categorical variables represent types of data which may be divided into groups. Examples of categorical variables are race, sex, age group, and educational level. While the latter two variables may also be considered in a numerical manner by using exact values for age and highest grade completed, it is often more informative to categorize such variables into a relatively small number of groups.
Analysis of categorical data generally involves the use of data tables. A two-way table presents categorical data by counting the number of observations that fall into each group for two variables, one divided into rows and the other divided into columns.Â
In a nutshell, the high-level formulation of the classification problem is given as follows:
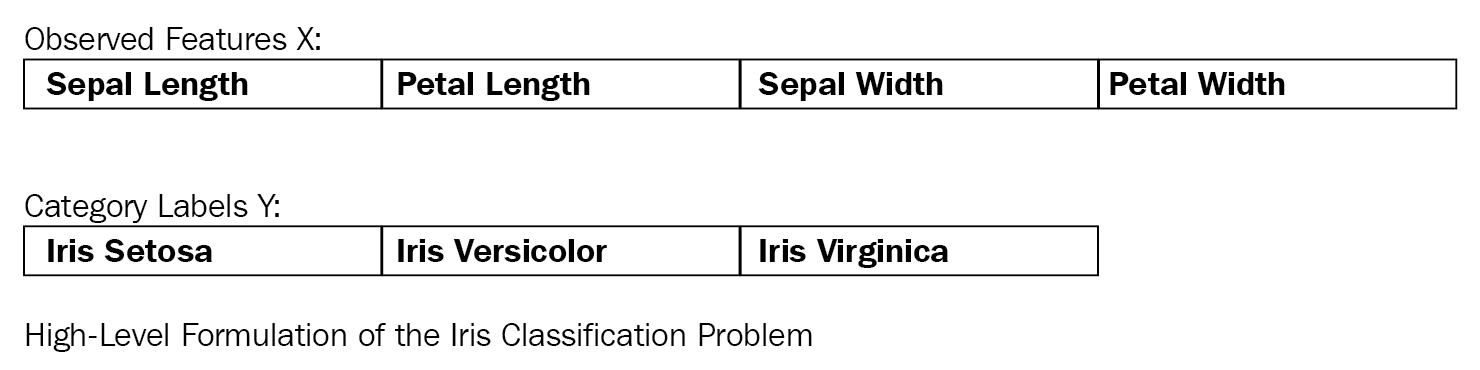
The formulation consists of the following:
- Observed featuresÂ
- Category labelsÂ
Observed features are also known as predictor variables. Such variables have predetermined measured values. These are the inputs X. On the other hand, category labels denote possible output values that predicted variables can take.Â
The predictor variables are as follows:
- sepal_length: It represents sepal length, in centimeters, used as input
- sepal_width: It represents sepal width, in centimeters, used as input
- petal_length: It represents petal length, in centimeters, used as input
- petal_width: It represents petal width, in centimeters, used as input
- setosa: It represents Iris-setosa, true or false, used as target
- versicolour: It represents Iris-versicolour, true or false, used as target
- virginica: It represents Iris-virginica, true or false, used as target
Four outcome variables were measured from each sample; the length and the width of the sepals and petals.
The total build time of the project should be no more than a day in order to get everything working. For those new to the data science area, understanding the background theory, setting up the software, and getting to build the pipeline could take an extra day or two.