Up until now, we have looked at the forward view or what the agent perceives to be as the next best reward or state. In MC, we looked at the entire episode and then used those values to reverse calculate returns. For TDL methods such as Q-learning and SARSA, we looked a single step ahead or what we referred to as TD (0). However, we want our agents to be able to take into account several steps, n, in advance. If we can do this, then surely our agent will be able to make better decisions.
As we have seen previously, we can average returns across steps using a discount factor, gamma. However, at this point, we need to more careful about how we average or collect returns. Instead, we can define the averaging of all returns over an infinite number of steps forward as follows:
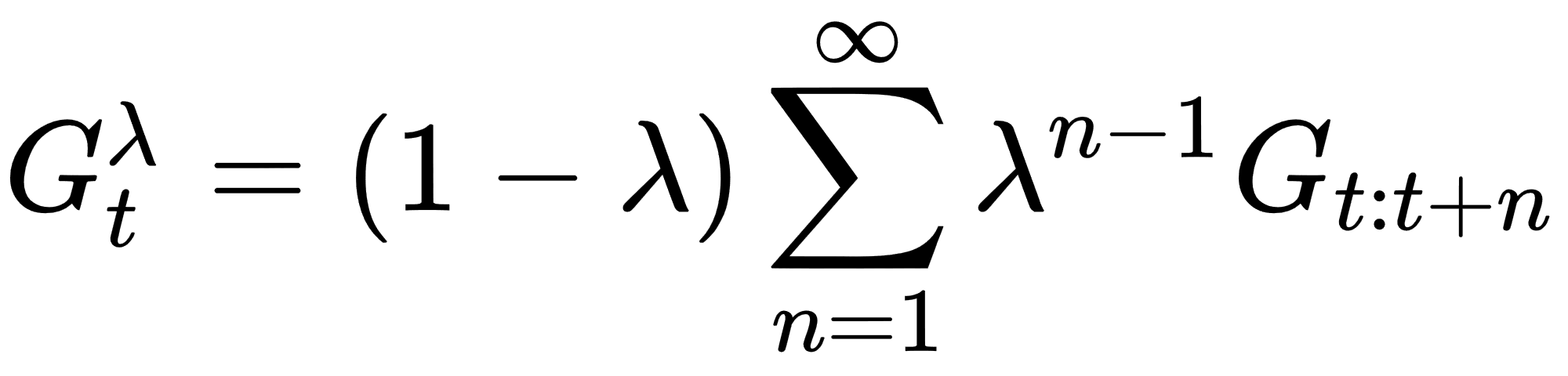
In the preceding equation, we have the following:
This...