Introduced in 2014 by Ian Goodfellow et al. in the paper Generative Adversarial Networks, GANs have revolutionized the field of generative models, opening the road to incredible applications.
GANs are frameworks that are used for the estimation of generative models via an adversarial process in which two models, the Generator and the Discriminator, are trained simultaneously.
The goal of the generative model (Generator) is to capture the data distribution contained in the training set, while the discriminative model acts as a binary classifier. Its goal is to estimate the probability of a sample to come from the training data rather than from the Generator. In the following diagram, the general architecture of adversarial training is shown:
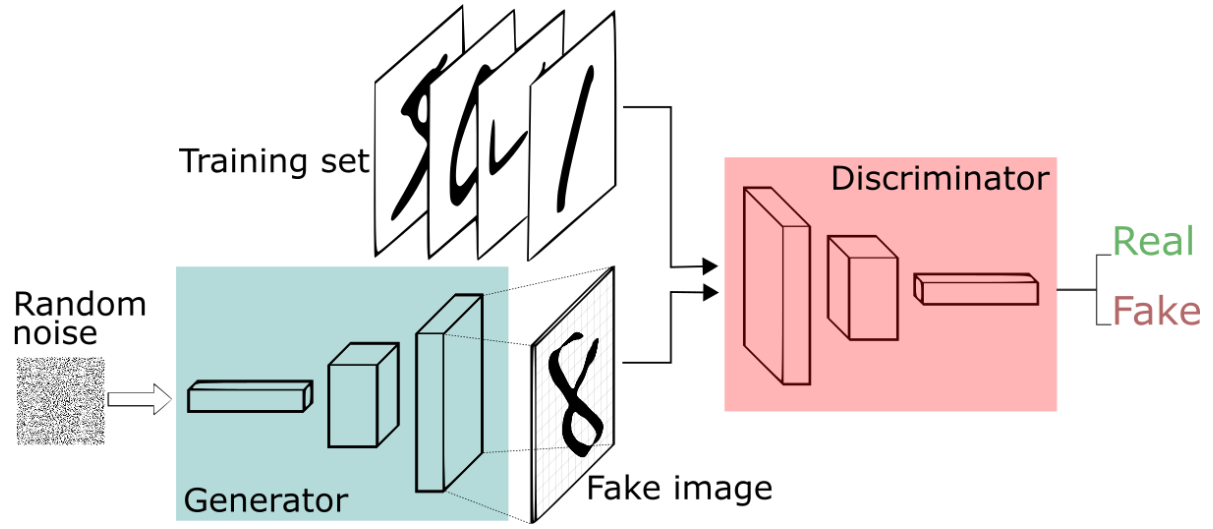
Graphical representation of the adversarial training process. The generator goal is used to fool...