So, let's try to understand the core mechanics of generative networks and how such approaches differ from the ones we already know. In our quest thus far, most of the networks we have implemented are for the purpose of executing a deterministic transformation of some inputs, in order to get to some sort of outputs. It was not until we explored the topic of reinforcement learning (Chapter 7, Reinforcement Learning with Deep Q-Networks) that we learned the benefits of introducing a degree of stochasticity (that is, randomness) to our modeling efforts. This is a core notion that we will be further exploring as we familiarize ourselves with the manner in which generative networks function. As we mentioned earlier, the central idea behind generative networks is to use a deep neural network to learn the probability distribution of variables...




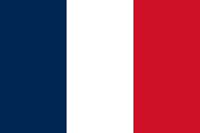


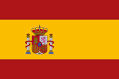



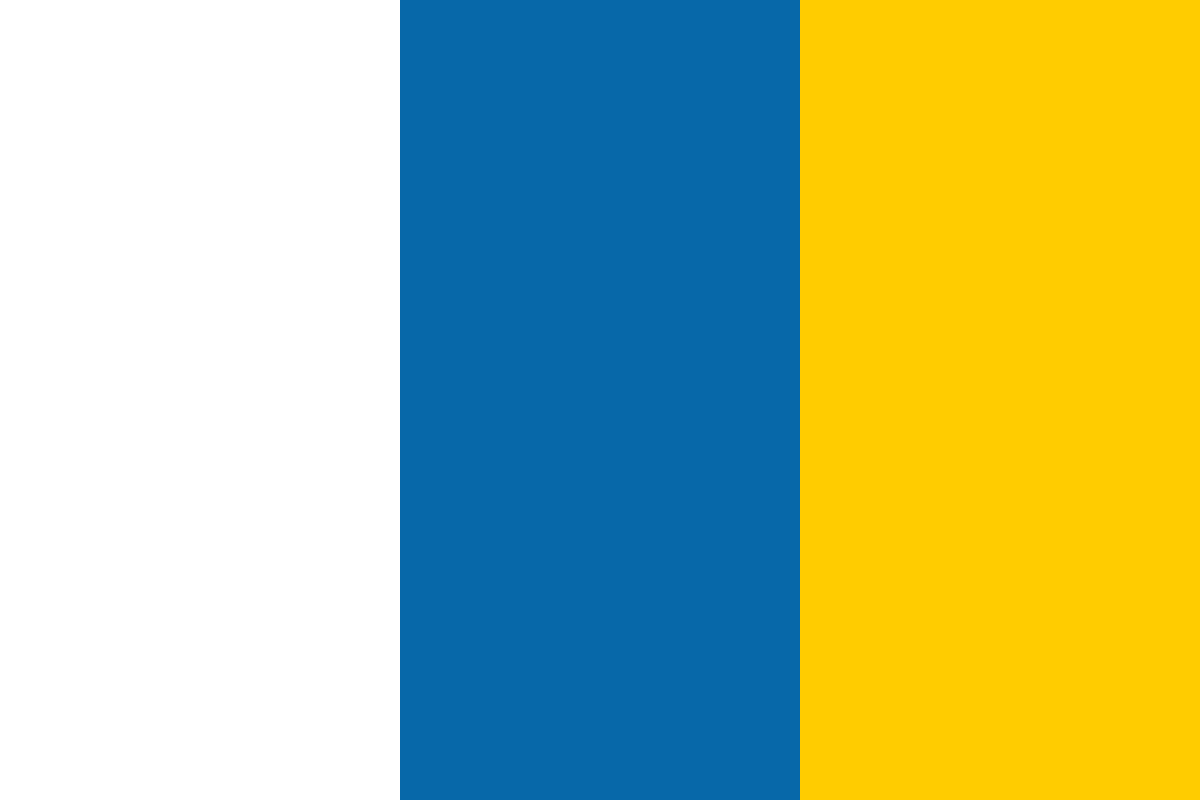
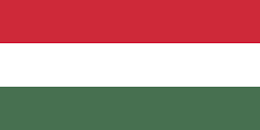

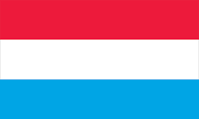





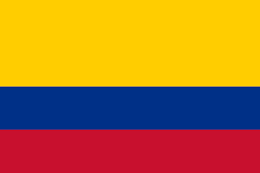
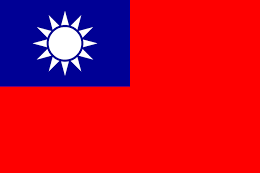
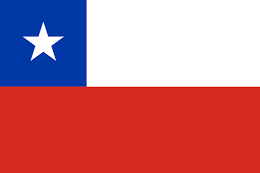



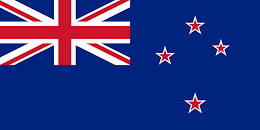
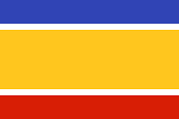
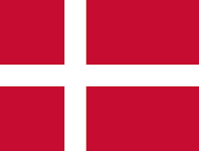
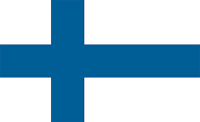







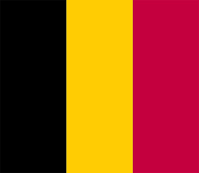

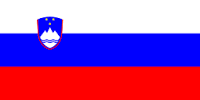





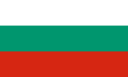


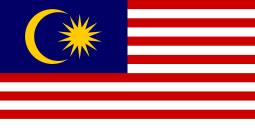



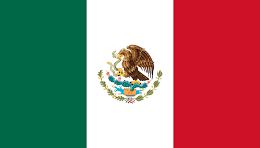
