In this section, we reintroduce kernel density estimation (KDE). When using kernel density estimation, we are attempting to reveal the shape of a dataset with a limited amount of information. Also, in this section, we're going to investigate which movies in the dataset have the highest rating; we're going to compute the KDE of a select group of movies using their rating; and, finally, compute the KDE overlap of two movies.
Let's go back to our MovieLens dataset notebook and import Data.Maybe, as shown in the following example:
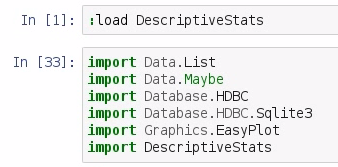
If you recall, this library is used in our KDE function. So, we are going to use the KDE function, which is almost identical to what we saw in the last section. The one addition is that we have added a normal line to the bottom of the function, as demonstrated in the following example:
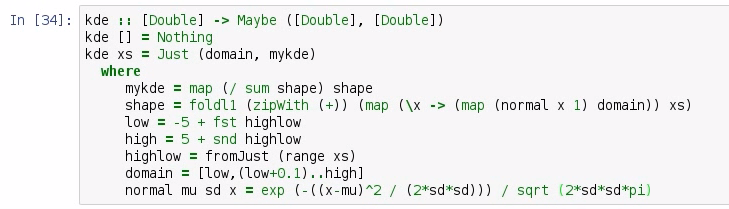
This is a normal...