The training process
Now that you know how the state of the cube is encoded in a 20 × 24 tensor, let's talk about the NN architecture and how it is trained.
The NN architecture
On the figure that follows (taken from the paper), the network architecture is shown.
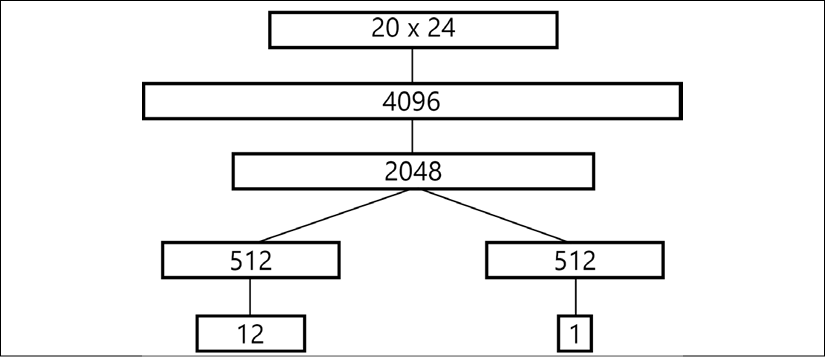
Figure 24.2: The NN architecture transforming the observation (top) to the action and value (bottom)
As the input, it accepts the already familiar cube state representation as a 20 × 24 tensor and produces two outputs:
- The policy, which is a vector of 12 numbers, representing the probability distribution over our actions.
- The value, a single scalar estimating the "goodness" of the state passed. The concrete meaning of a value will be discussed later.
Between the input and output, the network has several fully connected layers with exponential linear unit (ELU) activations. In my implementation, the architecture is exactly the same as in the paper, and the model is...