Unlike LSTMs, GRUs do not use a memory unit to control the flow of information and can directly make use of all the hidden states. Instead of using a cell state, GRUs use the hidden state to transfer information. GRUs usually train faster than other memory-based neural networks because of the fact that they have fewer parameters to train, fewer tensor operations, and can work well with fewer data. GRUs have two gates. These are known as the reset gate and the update gate. The reset gate is used to determine how to combine new inputs with the previous memory, while the update gate determines how much information to keep from the previous state. If you compare this with LSTMs, the update gate in a GRU is comparable to what the input and forget gates do in an LSTM. It decides what information to add or remove. GRUs also merge the...




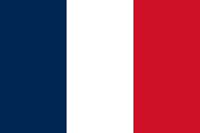


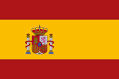




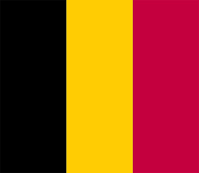
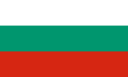
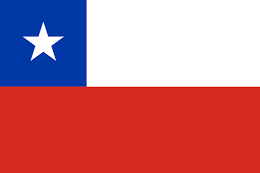
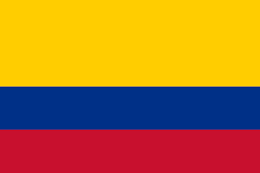
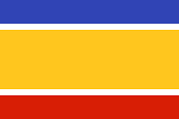

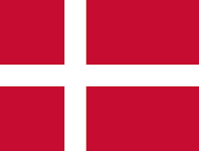



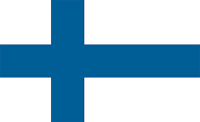

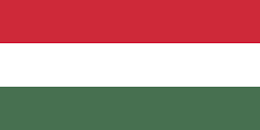






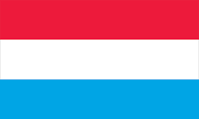
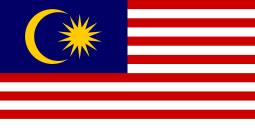

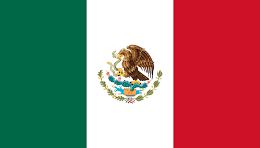

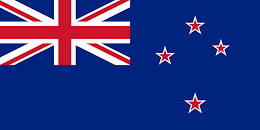







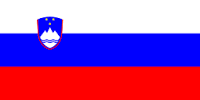




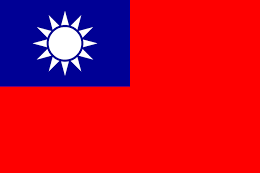


