Key metrics for optimizing models
When it comes to real-time inference, optimizing a model for performance usually includes metrics such as latency, throughput, and model size. To optimize the model size, the process typically involves having a trained model, checking the size of the model, and if it does not fit into single CPU/GPU memory, you can choose any of the techniques discussed in the Reducing the memory footprint of DL models section to prepare it for deployment.
For deployment, one of the best practices is to standardize the environment. This will involve the use of containers to deploy the model, irrespective of whether you are deploying it on your own server or using Amazon SageMaker. The process is illustrated in Figure 9.6.
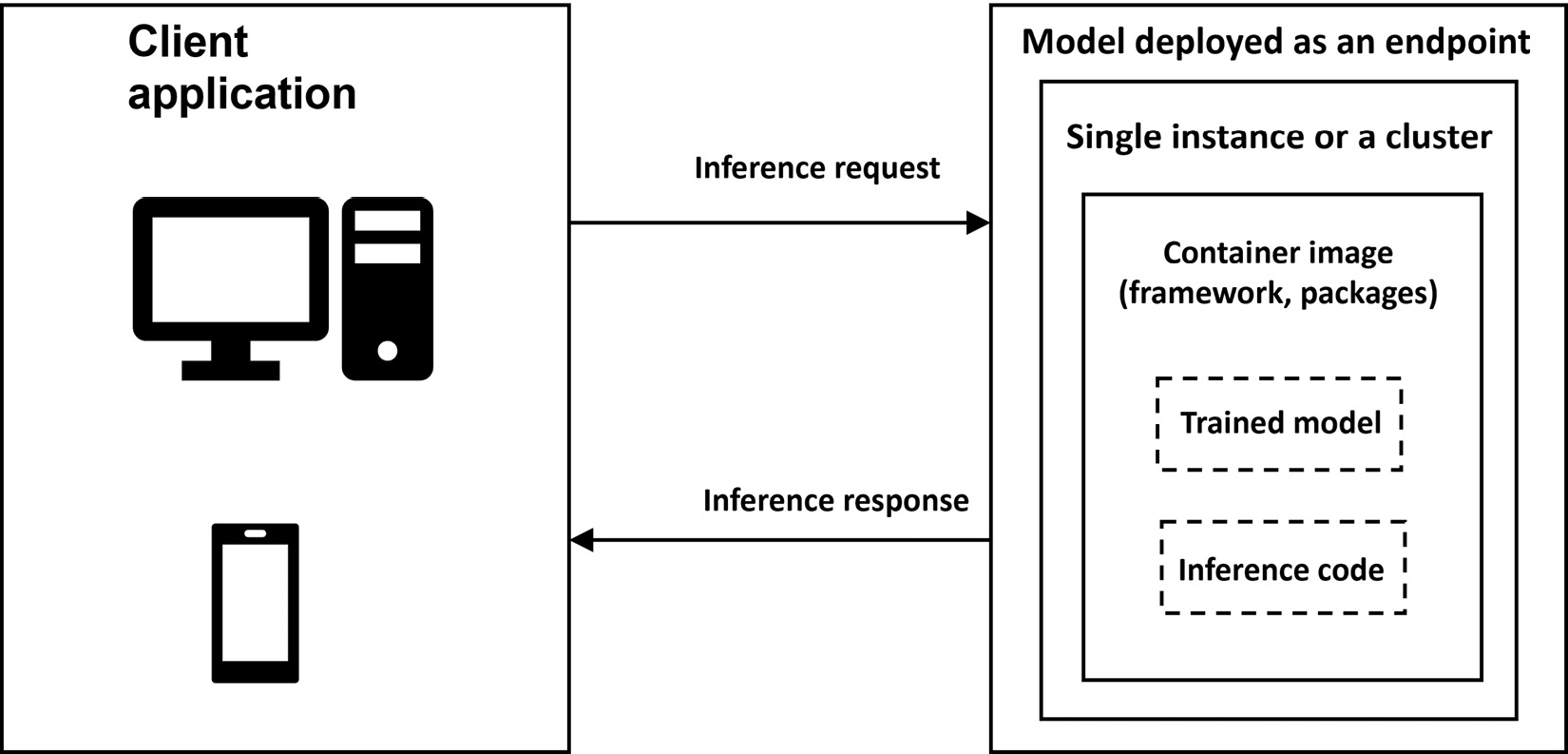
Figure 9.6 – Model deployed as a real-time endpoint
To summarize, we will first prepare the model for deployment, select or create a container to standardize the environment, followed by deploying the container...