




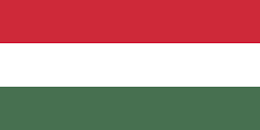

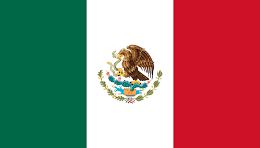


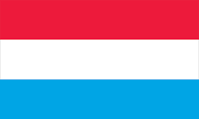



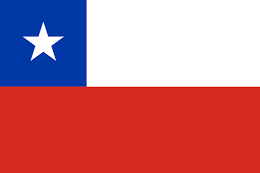



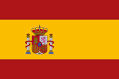


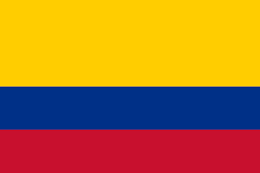
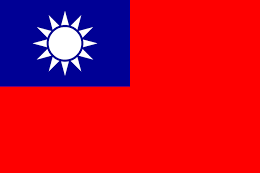


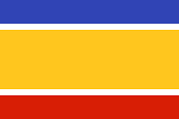
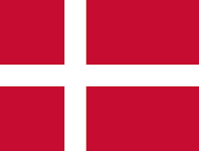
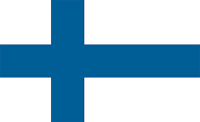



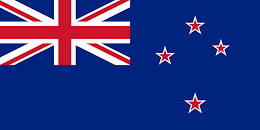


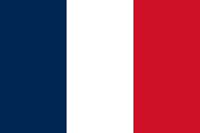



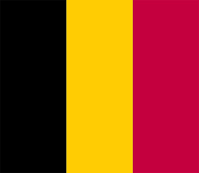

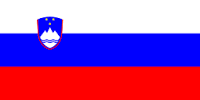




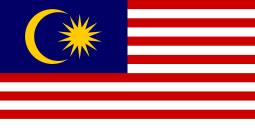


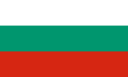




Dive deeper into the world of AI innovation and stay ahead of the AI curve! Subscribe to our AI_Distilled newsletter for the latest insights. Don't miss out – sign up today!
In the modern digital age, artificial intelligence has changed how we handle complex tasks, including image analysis. Advanced models like ChatGPT have made this process more interactive and insightful. Instead of a basic understanding, users can now guide the system through prompts to get a detailed analysis of an image. This approach helps in revealing both broad themes and specific details. In this blog, we will look at how ChatGPT responds to a series of prompts, demonstrating the depth and versatility of AI-powered image analysis. Let’s start
Here's a step-by-step guide to doing image analysis with ChatGPT:
Now to perform the image analysis we have deployed the Chain prompting technique. Here’s an example:
Chain prompting refers to the practice of building a sequence of interrelated prompts that progressively guide an artificial intelligence system to deliver desired responses. By initiating with a foundational prompt and then following it up with subsequent prompts that build upon the previous ones, users can engage in a deeper and more nuanced interaction with the system.
The essence of chain prompting lies in its iterative nature. Instead of relying on a single, isolated question, users employ a series of interconnected prompts that allow for refining, expanding, or branching the AI's output. This approach can be particularly useful in situations where a broad topic needs to be explored in depth, or when the user is aiming to extract multifaceted insights.
For instance, in the domain of image analysis, an initial prompt might request a general description of an image. Subsequent prompts can then delve deeper into specific aspects of the image, ask for comparisons, or even seek interpretations based on the initial description. Now Let’s dissect the nature of prompts given in the example below for analysis. These prompts are guiding the system through a process of image analysis. Starting from a general interpretation, they progressively request more specific and actionable insights based on the content of the image. The final prompt adds a layer of self-reflection, asking the system to assess the nature of the prompts themselves.
The below roadmap was taken from the infographics shared on LinkedIn by Mr Ravit Jain and can be found here.
Analysis: This prompt is a general inquiry to see if the system can extract and interpret information from the provided image. The user is essentially asking if the system has the capability to understand and process visual data.
Response:
Analysis: This prompt requests a comprehensive description of the content within the image, focusing specifically on the "data science landscape." The user is looking for an interpretation of the image that summarizes its main points regarding data science.
Response:
Analysis: This prompt asks the system to provide actionable advice or recommendations. Using the previously described content of the image, the user wants to know which skills are most essential for someone new ("fresher") to the data science field.
Response:
Analysis: This prompt requests a more detailed breakdown or categorization of the image's content. The user is looking for a mapping of the various skills mentioned in the image to specific career paths within data science.
Response:
Analysis: This prompt seems to be a combination of Prompt 4 and a meta-analysis request. The first part reiterates the mapping request from Prompt 4. The second part asks the system to provide a reflective analysis of the prompts themselves in relation to image analysis (which is what we're doing right now).
In conclusion, image analysis, when used with advanced models like ChatGPT, offers significant benefits. Our review of various prompts shows that users can obtain a wide range of insights from basic image descriptions to in-depth interpretations and career advice. The ability to direct the AI with specific questions and modify the analysis based on prior answers provides a customized experience. As technology progresses, the potential of AI-driven image analysis will likely grow. For those in professional, academic, or hobbyist roles, understanding how to effectively engage with these tools will become increasingly important in the digital world.
Dr. Anshul Saxena is an author, corporate consultant, inventor, and educator who assists clients in finding financial solutions using quantum computing and generative AI. He has filed over three Indian patents and has been granted an Australian Innovation Patent. Anshul is the author of two best-selling books in the realm of HR Analytics and Quantum Computing (Packt Publications). He has been instrumental in setting up new-age specializations like decision sciences and business analytics in multiple business schools across India. Currently, he is working as Assistant Professor and Coordinator – Center for Emerging Business Technologies at CHRIST (Deemed to be University), Pune Lavasa Campus. Dr. Anshul has also worked with reputed companies like IBM as a curriculum designer and trainer and has been instrumental in training 1000+ academicians and working professionals from universities and corporate houses like UPES, CRMIT, and NITTE Mangalore, Vishwakarma University, Pune & Kaziranga University, and KPMG, IBM, Altran, TCS, Metro CASH & Carry, HPCL & IOC. With a work experience of 5 years in the domain of financial risk analytics with TCS and Northern Trust, Dr. Anshul has guided master's students in creating projects on emerging business technologies, which have resulted in 8+ Scopus-indexed papers. Dr. Anshul holds a PhD in Applied AI (Management), an MBA in Finance, and a BSc in Chemistry. He possesses multiple certificates in the field of Generative AI and Quantum Computing from organizations like SAS, IBM, IISC, Harvard, and BIMTECH.
Author of the book: Financial Modeling Using Quantum Computing