



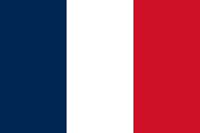


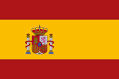



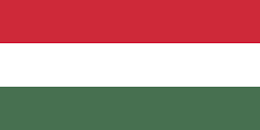

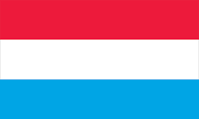





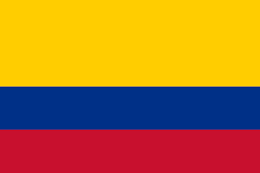
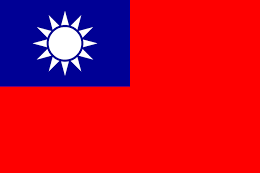
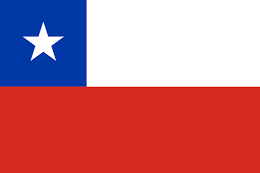



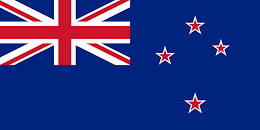
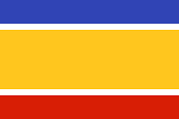
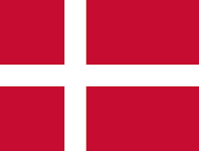
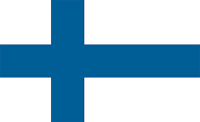







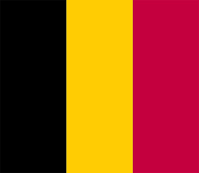

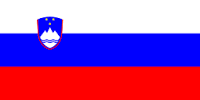





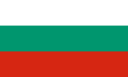


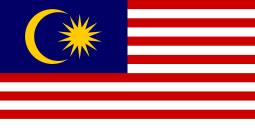



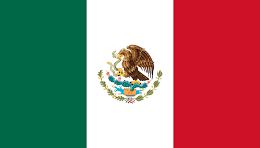

Dive deeper into the world of AI innovation and stay ahead of the AI curve! Subscribe to our AI_Distilled newsletter for the latest insights. Don't miss out – sign up today!
Managing personal finances is a cumbersome process. Receipts, bank statements, credit card bills, and expense records accumulate quickly. Despite our best intentions, many of us often struggle to maintain a clear overview of our financial health. It's not uncommon to overlook expenses or procrastinate on updating our budgets. Inevitably, this leads to financial surprises and missed opportunities for financial growth.
Even when we diligently track our expenses, the data can be overwhelming. Analyzing every transaction to identify patterns, pinpoint areas for improvement, and set financial goals is no easy feat. It's challenging to answer questions like, "Am I spending too much on entertainment?" or "Is my investment portfolio well-balanced?" or "Should I cut back on dining out?" or "Do I need to limit socializing with friends?" and "Is it time to increase my investments?"
Imagine having a personal assistant capable of automating these financial tasks, effortlessly transforming your transaction history into valuable insights. What if, at the end of each month, you received comprehensive financial analyses and actionable recommendations? Thanks to the rapid advancements in generative AI, this dream has become a reality, made possible by the incredible capabilities of LLM. No more endless hours of spreadsheet tinkering or agonizing over budgeting apps.
In this article, we'll delve into the development of a personal financial advisor powered by LangChain. This virtual assistant will not only automate the tracking of your finances but also provide tailored recommendations based on your unique spending patterns and financial goals.
Building an AI-powered personal financial advisor is an exciting endeavor. Here's an overview of how the personal financial advisor operates:
The benefits of having an AI-powered personal financial advisor are numerous:
Without wasting any more time, let’s take a deep breath, make yourselves comfortable, and be ready to learn how to build your AI-based personal financial advisor with LangChain!
LangChain is developed to harness the incredible potential of LLM, LangChain enables the creation of applications that are not only context-aware but also capable of reasoning, all while maintaining a user-friendly and modular approach.
LangChain is more than just a framework; it's a paradigm shift in the world of language model-driven applications. Here's a closer look at what makes LangChain a transformative force:
The power of LangChain lies in its value propositions, which make it an indispensable tool for developers and businesses looking to harness the potential of language models:
LangChain isn't just a run-of-the-mill framework; it's a versatile toolkit designed to empower developers to create sophisticated language model-powered applications. At the heart of LangChain is a set of interconnected modules, each serving a unique purpose. These modules are the building blocks that make LangChain a powerhouse for AI application development.
Let’s start building our personal financial advisor with LangChain! For the sake of simplicity, let’s consider only three data sources: monthly credit card statements, bank account statements, and cash expense logs. The following is an example of the data format for each of the sources.
##
Monthly Credit Card Statement
Date: 2023-09-01
Description: Grocery Store
Amount: $150.00
Balance: $2,850.00
Date: 2023-09-03
Description: Restaurant Dinner
Amount: $50.00
Balance: $2,800.00
Date: 2023-09-10
Description: Gas Station
Amount: $40.00
Balance: $2,760.00
Date: 2023-09-15
Description: Utility Bill Payment
Amount: $100.00
Balance: $2,660.00
Date: 2023-09-20
Description: Salary Deposit
Amount: $3,000.00
Balance: $5,660.00
Date: 2023-09-25
Description: Online Shopping
Amount: $200.00
Balance: $5,460.00
Date: 2023-09-30
Description: Investment Portfolio Contribution
Amount: $500.00
Balance: $4,960.00
##
Bank Account Statement
Date: 2023-08-01
Description: Rent Payment
Amount: $1,200.00
Balance: $2,800.00
Date: 2023-08-05
Description: Grocery Store
Amount: $200.00
Balance: $2,600.00
Date: 2023-08-12
Description: Internet and Cable Bill
Amount: $80.00
Balance: $2,520.00
Date: 2023-08-15
Description: Freelance Gig Income
Amount: $700.00
Balance: $3,220.00
Date: 2023-08-21
Description: Dinner with Friends
Amount: $80.00
Balance: $3,140.00
Date: 2023-08-25
Description: Savings Account Transfer
Amount: $300.00
Balance: $3,440.00
Date: 2023-08-29
Description: Online Shopping
Amount: $150.00
Balance: $3,290.00
##
Cash Expense Log
Date: 2023-07-03
Description: Coffee Shop
Amount: $5.00
Balance: $95.00
Date: 2023-07-10
Description: Movie Tickets
Amount: $20.00
Balance: $75.00
Date: 2023-07-18
Description: Gym Membership
Amount: $50.00
Balance: $25.00
Date: 2023-07-22
Description: Taxi Fare
Amount: $30.00
Balance: -$5.00 (Negative balance indicates a debt)
Date: 2023-07-28
Description: Bookstore
Amount: $40.00
Balance: -$45.00
Date: 2023-07-30
Description: Cash Withdrawal
Amount: $100.00
Balance: -$145.00
To create our personal financial advisor, we’ll use the chat model interface provided by LangChain. There are several important components to build a chatbot with LangChain:
First, we need to set the API key for our LLM. We’ll use OpenAI in this example.
import os
os.environ["OPENAI_API_KEY"] = “your openai key”
Then, we can simply load the necessary chat modules from LangChain.
from langchain.schema import (
AIMessage,
HumanMessage,
SystemMessage
)
from langchain.chat_models import ChatOpenAI
The ChatOpenAI is the main class that connects with the OpenAI LLM. We can pass `HumanMessage` and `SystemMessage` to this class and it will return the response from the LLM in the type of `AIMessage`.
chat = ChatOpenAI(model_name=”gpt-3.5-turbo”)
messages = [SystemMessage(content=prompt),
HumanMessage(content=data)]
chat(messages)
Let’s see the following example where we pass the prompt along with the data and the LLM returns the response via the ChatOpenAI object.
Boom! We just got our first analysis and recommendation from our personal financial advisor. This is a very simple example of how to create our personal financial advisor. Of course, there’s still a lot of room for improvement. For example, currently, we need to pass manually the relevant data sources as the HumanMessage. However, as mentioned before, LangChain provides a built-in class to perform retrieval. This means that we can just create another script to automatically dump all of the relevant data into some sort of document or even database, and then LangChain can directly read the data directly from there. Hence, we can get automated reports every month without needing to manually input the relevant data.
Congratulations on keeping up to this point! Throughout this article, you have learned what is LangChain, what it is capable of, and how to build a personal financial advisor with LangChain. Hope the best for your experiment in creating your personal financial advisor and see you in the next article!
Louis Owen is a data scientist/AI engineer from Indonesia who is always hungry for new knowledge. Throughout his career journey, he has worked in various fields of industry, including NGOs, e-commerce, conversational AI, OTA, Smart City, and FinTech. Outside of work, he loves to spend his time helping data science enthusiasts to become data scientists, either through his articles or through mentoring sessions. He also loves to spend his spare time doing his hobbies: watching movies and conducting side projects.
Currently, Louis is an NLP Research Engineer at Yellow.ai, the world’s leading CX automation platform. Check out Louis’ website to learn more about him! Lastly, if you have any queries or any topics to be discussed, please reach out to Louis via LinkedIn.