Naive Bayes classification
Let's get right into it! Let's begin with Naive Bayes classification. This machine learning model relies heavily on the results from the previous chapters, specifically with Bayes' theorem:
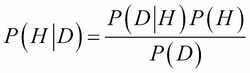
Let's look a little closer at the specific features of this formula:
- P(H) is the probability of the hypothesis before we observe the data, called the prior probability, or just prior
- P(H|D) is what we want to compute, the probability of the hypothesis after we observe the data, called the posterior
- P(D|H) is the probability of the data under the given hypothesis, called the likelihood
- P(D) is the probability of the data under any hypothesis, called the normalizing constant
Naive Bayes classification is a classification model, and therefore a supervised model. Given this, what kind of data do we need?
- Labeled data
- Unlabeled data
(Insert Jeopardy music here)
If you answered labeled data, then you're well on your way to becoming a data scientist!
Suppose we have...