Julia's key feature – multiple dispatch
A function is an object, mapping a tuple of arguments using some expression to a return value. When this function object is unable to return a value, it throws an exception. For different types of arguments the same conceptual function can have different implementations. For example, we can have a function to add two floating point numbers and another function to add two integers. But conceptually, we are only adding two numbers. Julia provides a functionality by which different implementations of the same concept can be implemented easily. The functions don't need to be defined all at once. They are defined in small abstracts. These small abstracts are different argument type combinations and have different behaviors associated with them. The definition of one of these behaviors is called a method.
The types and the number of arguments that a method definition accepts is indicated by the annotation of its signatures. Therefore, the most suitable method is applied whenever a function is called with a certain set of arguments. To apply a method when a function is invoked is known as dispatch. Traditionally, object-oriented languages consider only the first argument in dispatch. Julia is different as all of the function's arguments are considered (not just only the first) and then it choses which method should be invoked. This is known as multiple dispatch.
Multiple dispatch is particularly useful for mathematical and scientific code. We shouldn't consider that the operations belong to one argument more than any of the others. All of the argument types are considered when implementing a mathematical operator. Multiple dispatch is not limited to mathematical expressions as it can be used in numerous real-world scenarios and is a powerful paradigm for structuring the programs.
Methods in multiple dispatch
+
is a function in Julia using multiple dispatch. Multiple dispatch is used by all of Julia's standard functions and operators. For various possible combinations of argument types and count, all of them have many methods defining their behavior. A method is restricted to take certain types of arguments using the ::
type-assertion operator:
julia> f(x::Float64, y::Float64) = x + y
The function definition will only be applied for calls where x
and y
are both values of type Float64
:
julia> f(10.0, 14.0)
24.0
If we try to apply this definition to other types of arguments, it will give a method error.
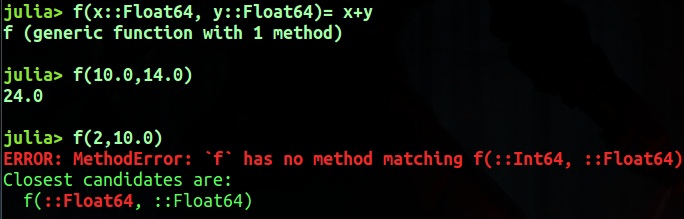
The arguments must be of precisely the same type as defined in the function definition.
The function object is created in the first method definition. New method definitions add new behaviors to the existing function object. When a function is invoked, the number and types of the arguments are matched, and the most specific method definition matching will be executed.
The following example creates a function with two methods. One method definition takes two arguments of the type Float64
and adds them. The second method definition takes two arguments of the type Number
, multiplies them by two and adds them. When we invoke the function with Float64
arguments, then the first method definition is applied, and when we invoke the function with Integer arguments, the second method definition is applied as the number can take any numeric values. In the following example, we are playing with floating point numbers and integers using multiple dispatch.
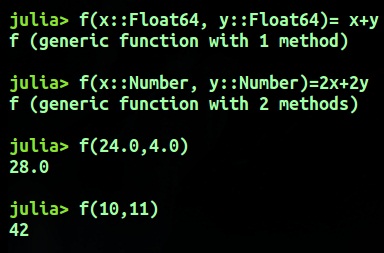
In Julia, all values are instances of the abstract type "Any". When the type declaration is not given with ::
, that means it is not specifically defined as the type of the argument, therefore Any is the default type of method parameter and it doesn't have the restriction of taking any type of value. Generally, one method definition is written in such a way that it will be applied to the certain arguments to which no other method definition applies. It is one of the Julia language's most powerful features.
It is efficient with a great ease of expressiveness to generate specialized code and implement complex algorithms without caring much about the low-level implementation using Julia's multiple dispatch and flexible parametric type system.
Ambiguities – method definitions
Sometimes function behaviors are defined in such a way that there isn't a unique method to apply for a certain set of arguments. Julia throws a warning in such cases about this ambiguity, but proceeds by arbitrarily picking a method. To avoid this ambiguity we should define a method to handle such cases.
In the following example, we define a method definition with one argument of the type Any
and another argument of the type Float64
. In the second method definition, we just changed the order, but this doesn't differentiate it from the first definition. In this case, Julia will give a warning of ambiguous method definition but will allow us to proceed.
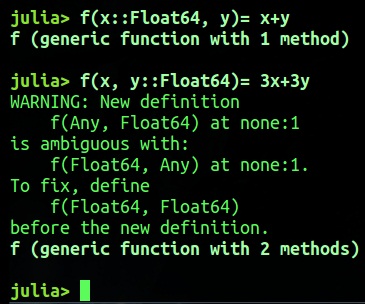