A GAN is informally described as an iterative game played between a detective and a counterfeiter; while the detective's goal is to minimize its loss by learning to identify real data as real and fake data as fake, the counterfeiter's goal is to minimize its loss by learning to fool the detective by transforming random noise into fake data. This informal definition can be described using the following figure:
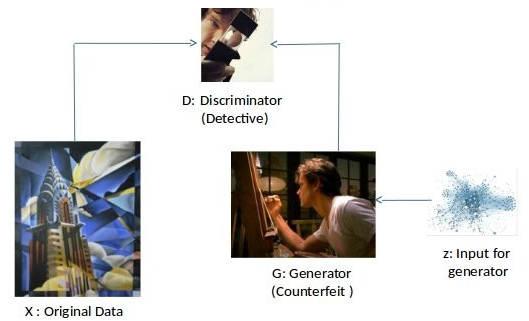
Source: Generative Adversarial Networks – A Deep Learning Architecture (https://hackernoon.com/generative-adversarial-networks-a-deep-learning-architecture-4253b6d12347)
Let's enumerate the building blocks of GANs and define the GAN framework formally:
- The discriminator,
, a detective
- The generator,
, a counterfeiter
- Real data,
,(images, audio, text)
- Fake data,
, (images, audio, text)
- Random noise,
,(uniform, Gaussian...