These are also known as the dimensions of big data. In 2001, Doug Laney first presented what became known as the three Vs of big data to describe some of the characteristics that make big data different from other data processing. These three Vs are volume, velocity, and variety. This the era of technological advancement and loads of research is going on. As a result of this reaches and advancements, these three Vs have become the six Vs of big data as of now. It may also increase in future. As of now, the six Vs of big data are volume, velocity, variety, veracity, variability, and value, as illustrated in the following diagram. These characteristics will be discussed in detailed later in the chapter:
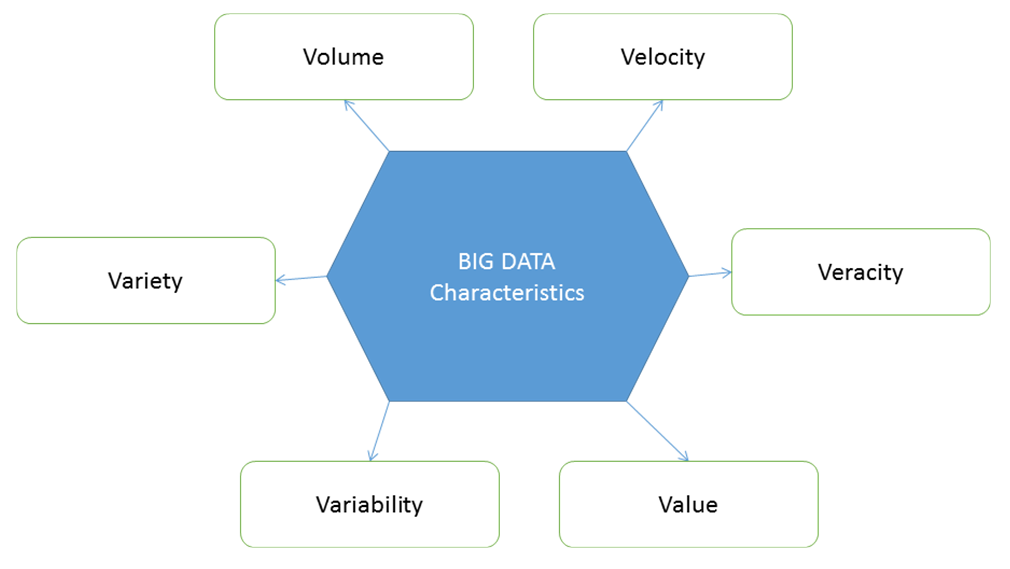
Different computer memory sizes are listed in the following table to give you an idea of the conversions between different units. It will let you understand the size of the data in upcoming examples in this book:
1 Bit |
Binary digit |
8 Bits |
1 byte |
1,024 Bytes |
1 KB (kilobyte) |
1,024 KB |
1 MB (megabyte) |
1,024 MB |
1 GB (gigabyte) |
1,024 GB |
1 TB (terabyte) |
1,024 TB |
1 PB (petabyte) |
1,024 PB |
1 EB (exabyte) |
1,024 EB |
1 ZB (zettabyte) |
1,024 ZB |
1 YB (yottabyte) |
1,024 YB |
1 brontobyte |
1,024 brontobyte |
1 geopbyte |
Â
Now that we have established our basis for subsequent discussions, let's move on to discuss the first characteristics of big data.