Gradient descent optimizers
The optimizers discussed here are widely used to train DL models depending on the degree of the non-convexity of the error or cost function.
Momentum
The momentum method uses a moving average gradient instead of a gradient at each time step and reduces the back-and-forth oscillations (fluctuations of the cost function) caused by SGD. This process focuses on the steepest descent path. Figure 4.5a shows movement with no momentum by creating oscillations in SGD while Figure 4.5b shows movement in the relevant direction by accumulating velocity with damped oscillations and closer to the optimum.
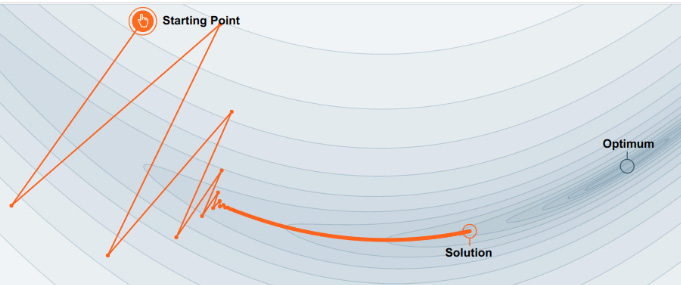
Figure 4.5a: SGD with no momentum
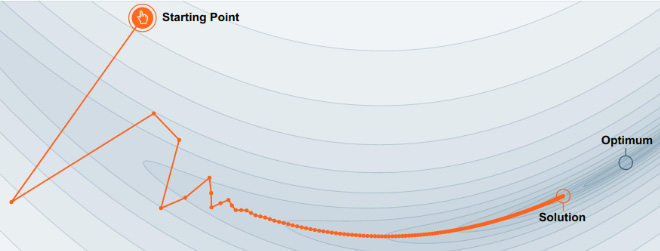
Figure 4.5b: SGD with momentum
The momentum term reduces updates for dimensions whose gradients change directions and as a result, faster convergence is achieved.
Adagrad
The adagrad
optimizer is used when dealing with sparse data as the algorithm performs small updates...