Comparing Amazon EMR with AWS Glue and AWS Glue DataBrew
When you look at today's big data processing frameworks, Spark is very popular for its in-memory processing capability. This is because it gives better performance compared to earlier Hadoop frameworks, such as MapReduce.
Earlier, we talked about different kinds of big data workloads you might have; it could be batch or streaming or a persistent/transient ETL use case.
Now, when you look for AWS services for your Spark workloads, EMR is not the only option AWS provides. You can use AWS Glue or AWS Glue DataBrew as an alternate service too. Customers often get confused between these services, and knowing what capabilities each of them has and when to use them can be tricky.
So, let's get an overview of these alternate services and then talk about what features they have and how to choose them by use case.
AWS Glue
AWS Glue is a serverless data integration service that is simple to use and is based on the Apache Spark engine. It enables you to discover, analyze, and transform the data through Spark-based in-memory processing. You can use AWS Glue for exploring datasets, doing ETL transformations, running real-time streaming pipelines, or preparing data for machine learning.
AWS Glue has the following components that you can benefit from:
- Glue crawlers and Glue Data Catalog: AWS Glue crawlers provide the benefit of deriving a schema from an S3 object store, where they scan a subset of data and create a table in Glue Data Catalog, on top of which you can execute SQL queries through Amazon Athena.
- Glue Studio and jobs: Glue Studio provides a visual interface to design ETL pipelines, which autogenerates PySpark or Scala scripts, which you can modify to integrate your complex business logic for data integration.
- Glue workflows: This enables you to build workflow orchestration for your ETL pipeline that can integrate Glue crawlers or jobs to be executed in sequence or parallel.
Please note, AWS Glue is a serverless offering, which means you don't have access to the underlying infrastructure and its pricing is based on Data Processing Units (DPUs). Each unit of DPU comprises 4 vCPU cores and 16 GB memory.
Example architecture for a batch ETL pipeline
Here is a simple reference architecture that you can follow to build a batch ETL pipeline. The use case is when data lands into the Amazon S3 landing zone from different sources and you need to build a centralized data lake on top of which you plan to do data analysis or reporting:
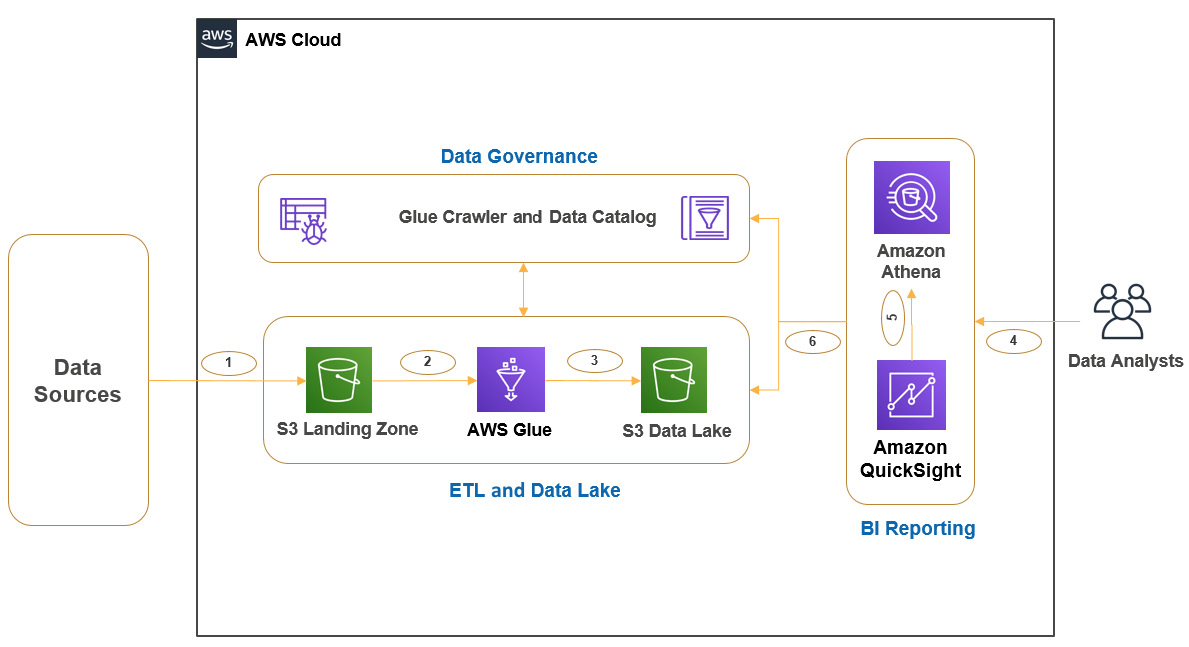
Figure 1.8 – Example architecture representing an AWS Glue ETL pipeline
As you can see in the diagram, we have the following steps:
- Step 1: Data lands into the Amazon S3 landing zone from different data sources, which becomes the raw zone for the data.
- Step 2-3: You will be using Glue crawlers and jobs to apply data cleansing, standardization, and transformation, and then make it available in an S3 data lake bucket for consumption.
- Step 4-6: Integrates flow to consume the data lake data for data analysis and business reporting. As you can see, we have integrated Amazon Athena to query data from Glue Data Catalog and S3 through standard SQL and integrated Amazon QuickSight for business intelligence reporting.
If you note, Glue crawlers and Glue Data Catalog are represented as a common centralized component for ETL transformations and data analysis. As your storage layer is Amazon S3, defining virtual schema on top of it will help you to access data through SQL as you do in relational databases.
AWS Glue DataBrew
AWS Glue DataBrew is a visual data preparation tool that assists data analysts and data scientists prepare data for data analysis or machine learning model training and inference. Often, data scientists spend 80% of their time preparing the data for analysis and 20% of the time on model development.
AWS Glue DataBrew solves that problem, where data scientists can save the effort of the steps from custom coding to clean, normalized data by building a transformation rule on the visual UI in minutes. AWS Glue DataBrew has 250+ prebuilt transformations (for example, filtering, adding derived columns, filtering anomalies, correcting invalid values, and joining or merging different datasets) that you can use to clean or transform your data, and it converts the visual transformation steps into a Spark script under the hood, which gives you faster performance.
It saves the transformation rules as recipes that you can apply to multiple jobs and can configure your job output format, partitioning strategy, and execution schedule. It also provides additional data profiling and lineage capability.
AWS Glue DataBrew is serverless, so you don't need to worry about setting up a cluster or managing its infrastructure resources. Its pricing is pretty similar to other AWS services, where you only pay for what you use.
Example architecture for machine learning data preparation
The following is a simple reference architecture that represents a data preparation use case for machine learning prediction and inference:
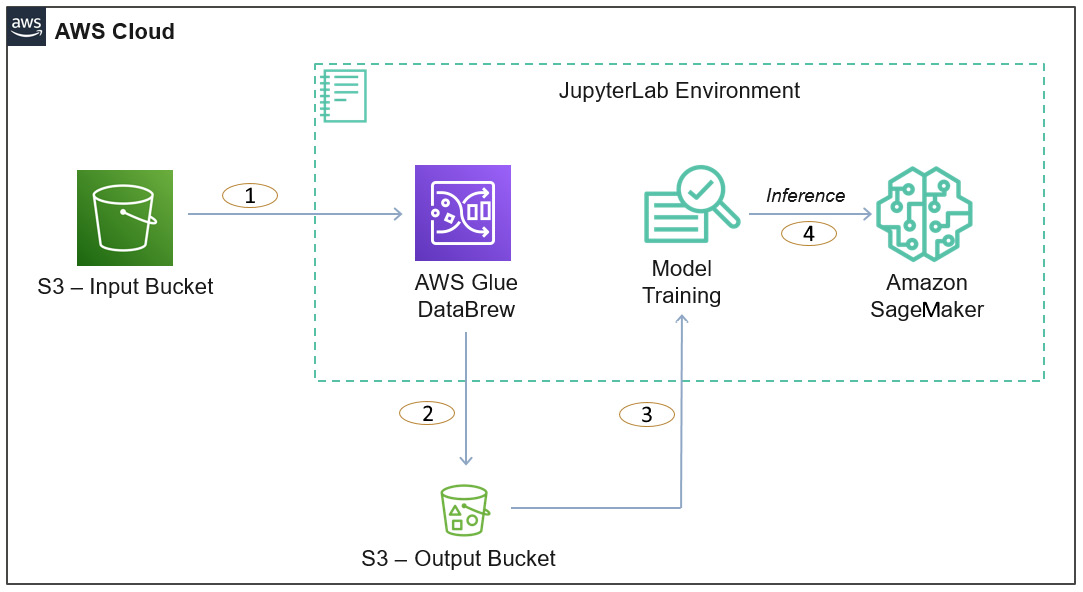
Figure 1.9 – An overall architecture representing data preparation with AWS Glue DataBrew
As you can see in the diagram, we have the following steps:
- Steps 1-2: Represents AWS Glue DataBrew reading data from the S3 input bucket and, after processing, writing the output back to the S3 output bucket
- Steps 3-4: Represents Amazon SageMaker using the processed data of the data bucket for machine learning training and inference, which also integrates Jupyter Notebook for model development
Now, let's look at how to decide which service is best for your use case.
Choosing the right service for your use case
Now, after getting an overview of all three AWS services, you can take note of the following guidelines when choosing the right service for your use case:
- AWS Glue DataBrew: If you are trying to build an ETL job or pipeline with Spark but you are new to Hadoop/Spark or you are not good at writing scripts for ETL transformations, then you can go for AWS Glue Data Brew, where you can use the GUI-based actions to preview your data and apply necessary transformation rules.
This is great when you receive different types of file formats from different systems and don't want to spend time writing code to prepare the data for analysis:
- Pros: Does not require you to learn Spark or scripting languages for preparing your data and also, you can build a data pipeline faster.
- Cons: Just because you are relying on the UI actions to build your pipeline, you lose the flexibility of building complex ETL operations that are not available through the UI. Also, it does not support real-time streaming use cases.
- Target users: Data scientists or data analysts can take advantage of this service as they spend time preparing the data or cleansing it for analysis and their objective is not to apply complex ETL operations.
- Use cases: Data cleansing and preparation with minimal ETL transformations.
- AWS Glue: If your objective is to build complex Spark-based ETL transformations by joining different data sources and you are looking for a serverless solution to avoid infrastructure management hassles, then AWS Glue is great.
On top of the Spark-based ETL job capability, AWS Glues crawlers, Glue Data Catalog, and workflows are also great benefits:
- Pros: Great for serverless Spark workloads that support both batch and streaming pipelines. You can use AWS Glue Studio to generate base code, on top of which you can edit.
- Cons: AWS Glue is limited to only Spark workloads and with Spark, you can use only Scala and Python. Also, if you have persistent cluster requirements, Glue is not a great choice.
- Target users: Data engineers looking for Spark-based ETL engines are best suited to use AWS Glue.
- Use cases: Batch and streaming ETL transformations and building a unified data catalog.
- Amazon EMR: As you have understood by now, AWS Glue or AWS Glue DataBrew are great for Spark-based workloads only and are great if you are looking for serverless options. But there are a lot of other use cases where organizations go with a combination of different Hadoop ecosystem services (for example, Hive, Flink, Presto, HBase, TensorFlow, and MXNet) or would like to have better control of not only the infrastructure, instance type and so on but also specific versions of Hadoop/Spark services they would like to use.
Also, sometimes you will have use cases where you might look for persistent Hadoop clusters that need to be used by multiple teams for different purposes, such as data analysis/preparation, ETL, real-time streaming, and machine learning models. EMR is a great fit there:
- Pros: Gives control to choose cluster capacity, instance types, and Hadoop services you need with version selection and also provides auto- and managed scaling features. Also provides flexibility to use spot instances for cost savings and have better control of the network and security of your cluster.
- Cons: Not a serverless offering like AWS Glue, but that's the purpose of EMR, to give you better control to configure your cluster.
- Target users: EMR can be used by mostly all kinds of users who deal with data on a daily basis, such as data engineers, data analysts, and data scientists.
- Use cases: Batch and real-time streaming, machine learning, interactive analytics, genomics, and so on.
I hope this gave you a good understanding of how you can choose the right AWS service for your Hadoop or Spark workloads and also how they compare with each other in terms of features, pros, and cons.